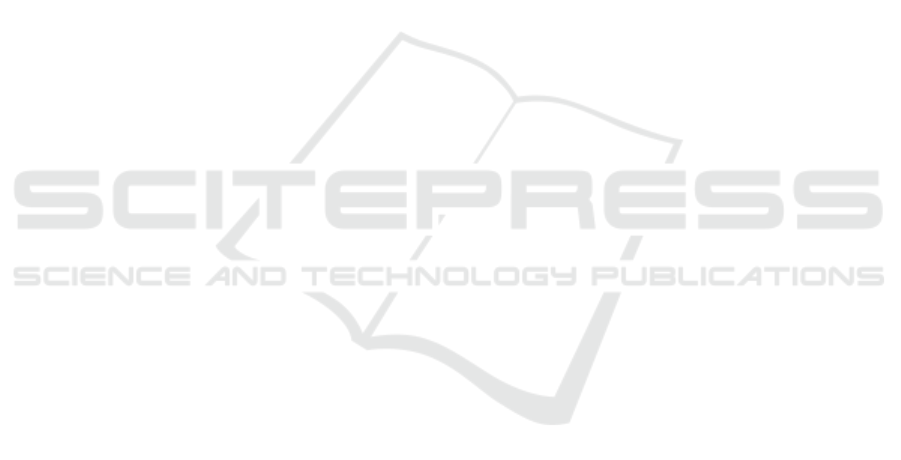
Jun, Y. and Choi, K. (2010). Design of patient-specific hip
implants based on the 3D geometry of the human fe-
mur. Advances in Engineering Software, 41(4):537–
547.
Keustermans, J., Vandermeulen, D., and Suetens, P. (2012).
Integrating Statistical Shape Models into a Graph Cut
Framework for Tooth Segmentation. pages 242–249.
Springer, Berlin, Heidelberg.
Khalifa, F., Soliman, A., Elmaghraby, A., Gimel’farb, G.,
and El-Baz, A. (2017). 3D Kidney Segmentation
from Abdominal Images Using Spatial-Appearance
Models. Computational and Mathematical Methods
in Medicine, 2017:1–10.
Kr
ˇ
cah, M., Sz
´
ekely, G., and Blanc, R. (2011). Fully auto-
matic and fast segmentation of the femur bone from
3D-CT images with no shape prior. Proceedings - In-
ternational Symposium on Biomedical Imaging, pages
2087–2090.
Krizhevsky, A., Sutskever, I., and Hinton, G. E. (2012).
ImageNet Classification with Deep Convolutional
Neural Networks.
Larios, N., Soran, B., Shapiro, L., Martinez-Munoz, G.,
Lin, J., and Dietterich, T. (2010). Haar Random Forest
Features and SVM Spatial Matching Kernel for Sto-
nefly Species Identification. In 2010 20th Internatio-
nal Conference on Pattern Recognition, pages 2624–
2627. IEEE.
Loh, W.-Y. (2011). Classification and regression trees.
Mahapatra, D. (2014). Analyzing Training Information
From Random Forests for Improved Image Segmen-
tation. IEEE Transactions on Image Processing,
23(4):1504–1512.
Milletari, F., Navab, N., and Ahmadi, S.-A. (2016). V-Net:
Fully Convolutional Neural Networks for Volumetric
Medical Image Segmentation. In 2016 Fourth Inter-
national Conference on 3D Vision (3DV), pages 565–
571. IEEE.
Pakdel, A., Robert, N., Fialkov, J., Maloul, A., and Whyne,
C. (2012). Generalized method for computation
of true thickness and x-ray intensity information in
highly blurred sub-millimeter bone features in clini-
cal CT images. Physics in Medicine and Biology,
57(23):8099–8116.
Parthasarathy, J. (2014). 3D modeling, custom implants and
its future perspectives in craniofacial surgery. Annals
of Maxillofacial Surgery, 4(1):9.
Pedregosa, F., Varoquaux, G., Gramfort, A., Michel, V.,
Thirion, B., Grisel, O., Blondel, M., Prettenhofer, P.,
Weiss, R., Dubourg, V., Vanderplas, J., Passos, A.,
Cournapeau, D., Brucher, M., Perrot, M., and Du-
chesnay, E. (2011). Scikit-learn: Machine learning
in Python. Journal of Machine Learning Research,
12:2825–2830.
Pinheiro, M. and Alves, J. L. (2015). A new level-set ba-
sed protocol for accurate bone segmentation from CT
imaging. IEEE Access, 3:1894–1906.
Prasoon, A., Petersen, K., Igel, C., Lauze, F., Dam, E., and
Nielsen, M. (2013). Deep Feature Learning for Knee
Cartilage Segmentation Using a Triplanar Convolutio-
nal Neural Network. pages 246–253. Springer, Berlin,
Heidelberg.
Rathnayaka, K., Sahama, T., Schuetz, M. A., and Schmutz,
B. (2011). Effects of CT image segmentation methods
on the accuracy of long bone 3D reconstructions. Me-
dical Engineering & Physics, 33(2):226–233.
Ronneberger, O., Fischer, P., and Brox, T. (2015). U-Net:
Convolutional Networks for Biomedical Image Seg-
mentation.
Schroeder, W. J., Martin, K. M., and Lorensen, W. E. The
Design and Implementation Of An Object-Oriented
Toolkit For 3D Graphics And Visualization.
Sharma, K., Peter, L., Rupprecht, C., Caroli, A., Wang, L.,
Remuzzi, A., Baust, M., and Navab, N. (2015). Semi-
Automatic Segmentation of Autosomal Dominant Po-
lycystic Kidneys using Random Forests.
Sharma, K., Rupprecht, C., Caroli, A., Aparicio, M. C., Re-
muzzi, A., Baust, M., and Navab, N. (2017). Auto-
matic Segmentation of Kidneys using Deep Learning
for Total Kidney Volume Quantification in Autosomal
Dominant Polycystic Kidney Disease. Scientific Re-
ports, 7(1):2049.
Shim, H., Chang, S., Tao, C., Wang, J.-H., Kwoh, C. K., and
Bae, K. T. (2009). Knee Cartilage: Efficient and Re-
producible Segmentation on High-Spatial-Resolution
MR Images with the Semiautomated Graph-Cut Algo-
rithm Method. Radiology, 251(2):548–556.
Tetsworth, K., Block, S., and Glatt, V. (2017). Putting 3D
modelling and 3D printing into practice: virtual sur-
gery and preoperative planning to reconstruct complex
post-traumatic skeletal deformities and defects. Sicot-
J, 3:16.
Virzi, A., Marret, J.-B., Muller, C., Berteloot, L., Boddaert,
N., Sarnacki, S., and Bloch, I. (2017). A new method
based on template registration and deformable models
for pelvic bones semi-automatic segmentation in pe-
diatric MRI. Proceedings - International Symposium
on Biomedical Imaging, pages 323–326.
Wu, D., Sofka, M., Birkbeck, N., and Zhou, S. K. (2014).
Segmentation of multiple knee bones from CT for ort-
hopedic knee surgery planning. Lecture Notes in Com-
puter Science (including subseries Lecture Notes in
Artificial Intelligence and Lecture Notes in Bioinfor-
matics), 8673 LNCS(PART 1):372–380.
Xi, T., Schreurs, R., Heerink, W. J., Berg
´
e, S. J., and
Maal, T. J. J. (2014). A novel region-growing ba-
sed semi-automatic segmentation protocol for three-
dimensional condylar reconstruction using cone beam
computed tomography (CBCT). PLoS ONE, 9(11):9–
14.
Yokota, F., Okada, T., Takao, M., Sugano, N., Tada, Y.,
Tomiyama, N., and Sato, Y. (2013). Automated CT
Segmentation of Diseased Hip Using Hierarchical and
Conditional Statistical Shape Models. pages 190–197.
Springer, Berlin, Heidelberg.
Zheng, S., Jayasumana, S., Romera-Paredes, B., Vineet, V.,
Su, Z., Du, D., Huang, C., and Torr, P. H. S. (2015).
Conditional Random Fields as Recurrent Neural Net-
works.
Semi-automatic CT Image Segmentation using Random Forests Learned from Partial Annotations
131