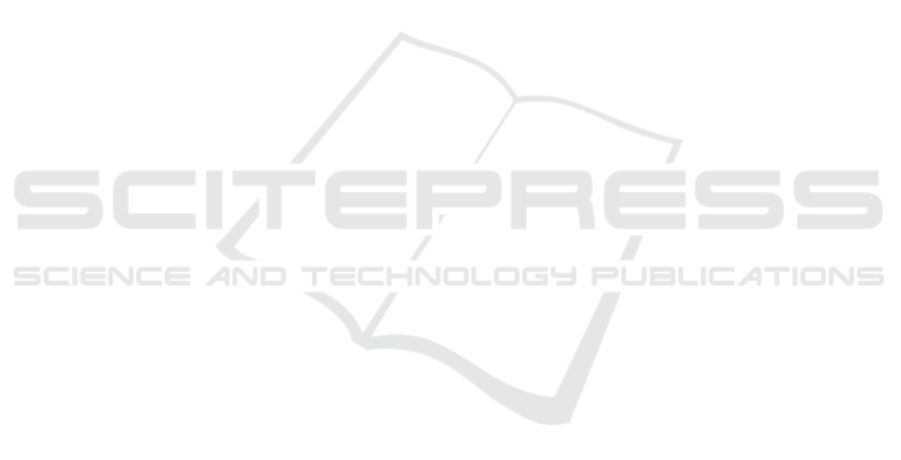
The best result for integrated feature space have
higher prediction accuracy 92.0%, in comparison
with EEG-only (72.4 %) mental status evaluation for
AF3, T7, O1, T8, AF4 channels. The best results for
MD-only data are 89.3% for LDA and NB methods.
4 DISCUSSION AND
CONCLUSION
According to the results of the of EEG data analysis
for various combinations of functional loads, the most
informative channels for Theta and Alpha rhythms in
the frontal, hip and occipital areas were identified.
For three different classifiers, the best accuracy of
classification of the five functional states in the EEG
generated characteristic space is at the level of 72.4%
for LDA method.
In turn, the classification in the attribute space of
the accelerometer, for the LDA and NB classifiers,
allows to reach 89.3% accuracy of identification of
five functional states. The processing of the joint
indicative space of the EEG –MD integrated core
features allowed to increase the classification
accuracy to 92.0% for DT method.
The results obtained in this paper reflect changes
in the power levels of the EEG indices in various
functional states, which makes it possible to
characterize the functional state of a person. The
decrease in the control effect of the cerebral cortex
(alpha-rhythm activity) increases the amplitude of the
average acceleration of the head movement. The
rather high classification accuracy obtained for the
signs of EEG signals isolated using the PCA method
suggests that changes in physiological processes
underlie these changes.
An increase in the accuracy of classification (on
19.6% in comparison with EEG-only feature), when
using the characteristics of both feature spaces can
mean that each of the signals carries information only
about a part of the changes in functional processes.
Thus, the task of determining the relationship
between EEG signals and the accelerometer on a
wider set of functional samples, when classifying
different mental states of a person at short time
intervals, is promising.
ACKNOWLEDGEMENTS
The work was supported by Act 211 Government of
the Russian Federation, contract № 02.A03.21.0006.
REFERENCES
Borisov, V., Syskov, A., Tetervak, V., Kublanov, V., 2017.
Mobile Brain - Computer Interface Application for
Mental Status Evaluation., in: Proceedings - 2017
International Multi-Conference on Engineering,
Computer and Information Sciences SIBIRCON.
Presented at the 2017 International Multi-Conference
on Engineering, Computer and Information Sciences
SIBIRCON, IEEE, Novosibirsk, Russia.
Danilov, Y.P., Tyler, M.E., Kaczmarek, K.A., 2008.
Vestibular sensory substitution using tongue
electrotactile display, in: Human Haptic Perception:
Basics and Applications. Birkhäuser Basel, pp. 467–
480. doi:10.1007/978-3-7643-7612-3_39.
David, H., Whitaker, K.W., Ries, A.J., Vettel, J.M.,
Cortney, B., Kerick, S.E., McDowell, K., 2014.
Usability of four commercially-oriented EEG systems.
J. Neural Eng. 11, 046018. doi:10.1088/1741-
2560/11/4/046018.
Egorova, D.D., Kazakov, Y.E., Kublanov, V.S., 2014.
Principal Components Method for Heart Rate
Variability Analysis. Biomed. Eng. 48, 37–41.
doi:10.1007/s10527-014-9412-7.
EMOTIV Epoc - 14 Channel Wireless EEG Headset
[WWW Document], n.d.. Emotiv. URL https://
www.emotiv.com/epoc/ (accessed 9.5.17).
Jolliffe, I., 2014. Principal Component Analysis, in: Wiley
StatsRef: Statistics Reference Online. John Wiley &
Sons, Ltd. doi:10.1002/9781118445112.stat06472.
Kublanov, V., Dolganov, A., Borisov, V., 2016.
Application of the discriminant analysis for diagnostics
of the arterial hypertension: Analysis of short-term
heart rate variability signals. Presented at the
NEUROTECHNIX 2016 - Proceedings of the 4th
International Congress on Neurotechnology,
Electronics and Informatics, pp. 45–52.
Kublanov, V.S., Dolganov, A.Y., Belo, D., Gamboa, H.,
2017. Comparison of Machine Learning Methods for
the Arterial Hypertension Diagnostics [WWW
Document]. Appl. Bionics Biomech.
doi:10.1155/2017/5985479.
Kutilek, P., Charfreitag, J., Hozman, J., 2010. Comparison
of Methods of Measurement of Head Position in
Neurological Practice, in: XII Mediterranean
Conference on Medical and Biological Engineering
and Computing 2010, IFMBE Proceedings. Springer,
Berlin, Heidelberg, pp. 455–458. doi:10.1007/978-3-
642-13039-7_114.
Lin, Y.-P., Jung, T.-P., 2017. Improving EEG-based
emotion classification using conditional transfer
learning. Front. Hum. Neurosci. 11.
doi:10.3389/fnhum.2017.00334.
Machado, I.P., Luísa, G., Gamboa, H., Paixão, V., Costa,
R.M., 2015. Human activity data discovery from
triaxial accelerometer sensor: Non-supervised learning
sensitivity to feature extraction parametrization. Inf.
Process. Manag. 51, 201–214.
doi:10.1016/j.ipm.2014.07.008.
Feature Extraction and Selection for EEG and Motion Data in Tasks of the Mental Status Assessing - Pilot Study using Emotiv EPOC+
Headset Signals
171