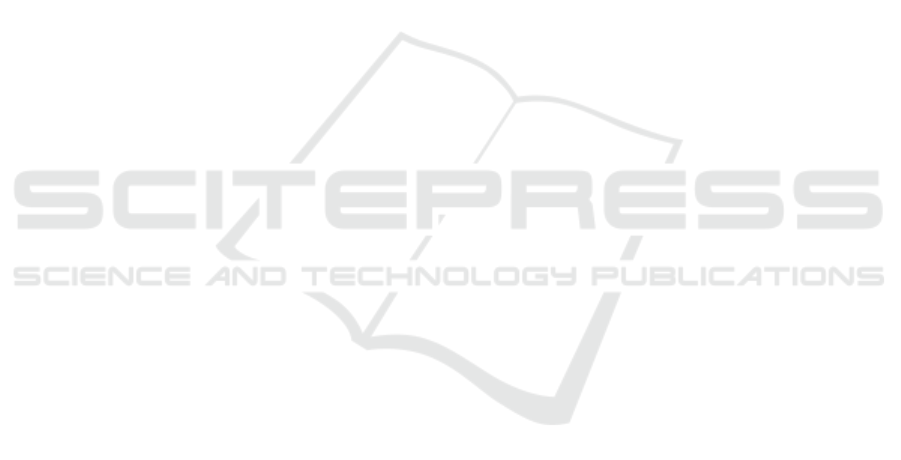
latency and persistence.’, The Journal of general
virology, 96 (Pt 4), pp. 739–51. doi: 10.1099/
vir.0.070862-0.
Griffiths-Jones, S. (2010) ‘miRBase: microRNA
sequences and annotation.’, Current protocols in
bioinformatics / editoral board, Andreas D. Baxevanis
... [et al.], Chapter 12, p. Unit 12.9.1-10. doi:
10.1002/0471250953.bi1209s29.
Hall, M. et al. (2009) ‘The WEKA data mining software’,
ACM SIGKDD Explorations Newsletter, 11(1), p. 10.
doi: 10.1145/1656274.1656278.
Hamzeiy, H., Allmer, J. and Yousef, M. (2014)
‘Computational methods for microRNA target
prediction.’, Methods in molecular biology (Clifton,
N.J.), 1107, pp. 207–21. doi: 10.1007/978-1-62703-
748-8_12.
Hsu, S.-D. et al. (2014) ‘miRTarBase update 2014: an
information resource for experimentally validated
miRNA-target interactions.’, Nucleic acids research,
42 (Database issue), pp. D78-85. doi: 10.1093/nar/
gkt1266.
Khalifa, W. et al. (2016) ‘The impact of feature selection
on one and two-class classification performance for
plant microRNAs.’, PeerJ. United States, 4, p. e2135.
doi: 10.7717/peerj.2135.
Letunic I, Bork P. Interactive Tree Of Life v2: online
annotation and display of phylogenetic trees made
easy. Nucleic Acids Res. 2011;39:W475–8.
Londin, E. et al. (2015) ‘Analysis of 13 cell types reveals
evidence for the expression of numerous novel
primate- and tissue-specific microRNAs’, Proceedings
of the National Academy of Sciences, 112(10), pp.
E1106–E1115. doi: 10.1073/pnas.1420955112.
Matthews, B. W. (1975) ‘Comparison of the predicted and
observed secondary structure of T4 phage lysozyme’,
BBA - Protein Structure, 405(2), pp. 442–451. doi:
10.1016/0005-2795(75)90109-9.
Mendoza, M. R. et al. (2013) ‘RFMirTarget: Predicting
Human MicroRNA Target Genes with a Random
Forest Classifier’, PLoS ONE, 8(7). doi: 10.1371/
journal.pone.0070153.
Peterson, S. M. et al. (2014) ‘Common features of
microRNA target prediction tools’, Frontiers in
Genetics. doi: 10.3389/fgene.2014.00023.
Saçar, M. and Allmer, J. (2014) ‘Machine Learning
Methods for MicroRNA Gene Prediction’, in Yousef,
M. and Allmer, J. (eds) miRNomics: MicroRNA
Biology and Computational Analysis SE - 10. Humana
Press (Methods in Molecular Biology), pp. 177–187.
doi: 10.1007/978-1-62703-748-8_10.
Sacar, M. D. and Allmer, J. (2013) ‘Data mining for
microrna gene prediction: On the impact of class
imbalance and feature number for microrna gene
prediction’, in 2013 8th International Symposium on
Health Informatics and Bioinformatics. IEEE, pp. 1–6.
doi: 10.1109/HIBIT.2013.6661685.
Saçar, M. D. and Allmer, J. (2013) ‘Current Limitations
for Computational Analysis of miRNAs in Cancer’,
Pakistan Journal of Clinical and Biomedical
Research, 1(2), pp. 3–5.
Saçar, M. D., Bağcı, C. and Allmer, J. (2014)
‘Computational Prediction of MicroRNAs from
Toxoplasma gondii Potentially Regulating the Hosts’
Gene Expression.’, Genomics, proteomics and
bioinformatics, 12(5), pp. 228–238. doi: 10.1016/
j.gpb.2014.09.002.
Saçar Demirci, M. D., Bağcı, C. and Allmer, J. (2016)
‘Differential Expression of T. gondii MicroRNAs in
Murine and Human Hosts’, in Non-coding RNAs and
inter-kingdom communication. Springer.
Saçar Demirci, M. D., Baumbach, J. and Allmer, J. (2017)
‘On the performance of pre-microRNA detection
algorithms’, Nature communications, 8(1), p. 330. doi:
10.1038/s41467-017-00403-z.
Vapnik, V. N. (1995) The nature of statistical learning
theory. New York, New York, USA: Springer-Verlag.
Vergoulis, T. et al. (2012) ‘TarBase 6.0: capturing the
exponential growth of miRNA targets with
experimental support.’, Nucleic acids research, 40
(Database issue), pp. D222-9. doi: 10.1093/nar/
gkr1161.
Xu, Q.-S. and Liang, Y.-Z. (2001) ‘Monte Carlo cross
validation’, Chemometrics and Intelligent Laboratory
Systems, 56(1), pp. 1–11. doi: 10.1016/S0169-
7439(00)00122-2.
Yousef, M. et al. (2007) ‘Naïve Bayes for microRNA
target predictions--machine learning for microRNA
targets.’, Bioinformatics (Oxford, England), 23(22),
pp. 2987–92. doi: 10.1093/bioinformatics/btm484.
Yousef, M., Nigatu, D., et al. (2017) ‘Categorization of
Species based on their MicroRNAs Employing
Sequence Motifs, Infor-mation-Theoretic Sequence
Feature Extraction, and k-mers’, EURASIP Journal on
Advances in Signal Processing.
Yousef, M. et al. (2017) ‘Distinguishing Between
MicroRNA Targets From Diverse Species Using
Sequence Motifs And K-Mers, Proceedings of
BIOSTEC 2017, 10th International Joint Conference
on Biomedical Engineering Systems and
Technologies’, Porto., 3.
Yousef, M., Khalifa, W., et al. (2017) ‘MicroRNA
categorization using sequence motifs and k-mers’,
BMC Bioinformatics, 18(1), p. 170. doi: 10.1186/
s12859-017-1584-1.
Yousef, M., Allmer, J. and Khalifa, W. (2016a) ‘Accurate
Plant MicroRNA Prediction Can Be Achieved Using
Sequence Motif Features’, Journal of Intelligent
Learning Systems and Applications, 8(1), pp. 9–22.
doi: 10.4236/jilsa.2016.81002.
Yousef, M., Allmer, J. and Khalifa, W. (2016b) ‘Feature
Selection for MicroRNA Target Prediction – Compari-
son of One-Class Feature Selection Methodologies’, in
Proceedings of the 9th International Joint Conference
on Biomedical Engineering Systems and Technologies,
pp. 216–225. doi: 10.5220/0005701602160225.
BIOINFORMATICS 2018 - 9th International Conference on Bioinformatics Models, Methods and Algorithms
118