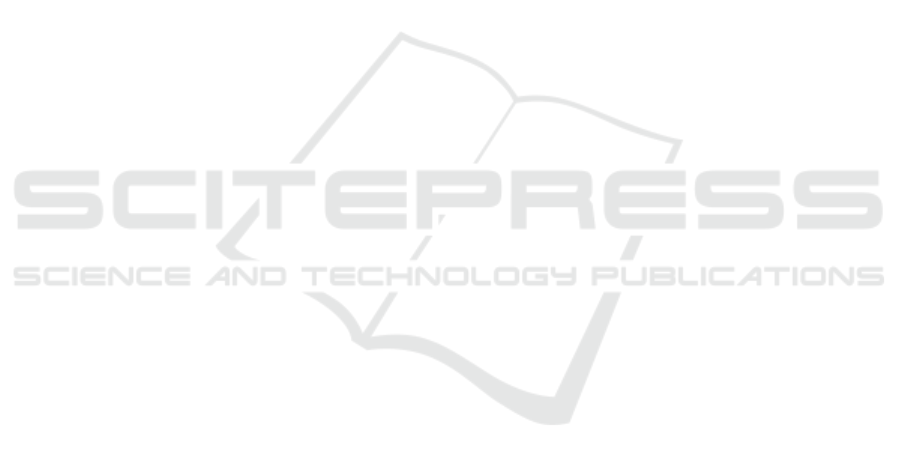
REFERENCES
Arandjelovi
´
c, R. and Zisserman, A. (2012). Three things
everyone should know to improve object retrieval. In
IEEE Conference on Computer Vision and Pattern Re-
cognition (CVPR), pages 2911–2918. IEEE.
Arevalillo-Herráez, M., Ferri, F. J., and Domingo, J. (2010).
A naive relevance feedback model for content-based
image retrieval using multiple similarity measures.
Pattern Recognition, 43(3):619–629.
Babenko, A. and Lempitsky, V. (2015). Aggregating local
deep features for image retrieval. In IEEE Internati-
onal Conference on Computer Vision (ICCV), pages
1269–1277.
Babenko, A., Slesarev, A., Chigorin, A., and Lempitsky, V.
(2014). Neural codes for image retrieval. In Euro-
pean conference on computer vision, pages 584–599.
Springer.
Cai, D., He, X., Li, Z., Ma, W.-Y., and Wen, J.-R. (2004).
Hierarchical clustering of www image search results
using visual, textual and link information. In Pro-
ceedings of the 12th annual ACM international con-
ference on Multimedia, pages 952–959. ACM.
Chen, Y., Wang, J. Z., and Krovetz, R. (2005). Clue:
cluster-based retrieval of images by unsupervised le-
arning. IEEE transactions on Image Processing,
14(8):1187–1201.
Cox, I. J., Miller, M. L., Minka, T. P., Papathomas, T. V., and
Yianilos, P. N. (2000). The bayesian image retrieval
system, pichunter: theory, implementation, and psy-
chophysical experiments. IEEE transactions on image
processing, 9(1):20–37.
Deselaers, T., Paredes, R., Vidal, E., and Ney, H. (2008).
Learning weighted distances for relevance feedback in
image retrieval. In Pattern Recognition, 2008. ICPR
2008. 19th International Conference on, pages 1–4.
IEEE.
Freytag, A., Schadt, A., and Denzler, J. (2015). Interactive
image retrieval for biodiversity research. In German
Conference on Pattern Recognition, pages 129–141.
Springer.
Glowacka, D., Teh, Y. W., and Shawe-Taylor, J. (2016).
Image retrieval with a bayesian model of relevance
feedback. arXiv:1603.09522.
Gordo, A., Almazan, J., Revaud, J., and Larlus, D. (2016).
End-to-end learning of deep visual representations for
image retrieval. arXiv:1610.07940.
Guo, G.-D., Jain, A. K., Ma, W.-Y., and Zhang, H.-J.
(2002). Learning similarity measure for natural image
retrieval with relevance feedback. IEEE Transactions
on Neural Networks, 13(4):811–820.
Huiskes, M. J. and Lew, M. S. (2008). The mir flickr retrie-
val evaluation. In MIR ’08: Proceedings of the 2008
ACM International Conference on Multimedia Infor-
mation Retrieval, New York, NY, USA. ACM.
Ishikawa, Y., Subramanya, R., and Faloutsos, C. (1998).
Mindreader: Querying databases through multiple ex-
amples. Computer Science Department, page 551.
Jégou, H., Douze, M., Schmid, C., and Pérez, P. (2010).
Aggregating local descriptors into a compact image
representation. In IEEE Conference on Computer Vi-
sion and Pattern Recognition (CVPR), pages 3304–
3311. IEEE.
Jégou, H. and Zisserman, A. (2014). Triangulation embed-
ding and democratic aggregation for image search. In
IEEE Conference on Computer Vision and Pattern Re-
cognition (CVPR), pages 3310–3317.
Jin, X. and French, J. C. (2003). Improving image retrieval
effectiveness via multiple queries. In ACM internatio-
nal workshop on Multimedia databases, pages 86–93.
ACM.
Kim, D.-H. and Chung, C.-W. (2003). Qcluster: rele-
vance feedback using adaptive clustering for content-
based image retrieval. In ACM SIGMOD international
conference on Management of data, pages 599–610.
ACM.
Lloyd, S. (1982). Least squares quantization in pcm. IEEE
transactions on information theory, 28(2):129–137.
Loeff, N., Alm, C. O., and Forsyth, D. A. (2006). Discri-
minating image senses by clustering with multimodal
features. In Proceedings of the COLING/ACL on Main
conference poster sessions, pages 547–554. Associa-
tion for Computational Linguistics.
Niblack, C. W., Barber, R., Equitz, W., Flickner, M. D.,
Glasman, E. H., Petkovic, D., Yanker, P., Faloutsos,
C., and Taubin, G. (1993). Qbic project: querying
images by content, using color, texture, and shape.
In IS&T/SPIE’s Symposium on Electronic Imaging:
Science and Technology, pages 173–187. Internatio-
nal Society for Optics and Photonics.
Poser, K. and Dransch, D. (2010). Volunteered geographic
information for disaster management with applica-
tion to rapid flood damage estimation. Geomatica,
64(1):89–98.
Rocchio, J. J. (1971). Relevance feedback in information
retrieval. The Smart retrieval system-experiments in
automatic document processing.
Simonyan, K. and Zisserman, A. (2014). Very deep con-
volutional networks for large-scale image recognition.
arXiv:1409.1556.
Tong, S. and Chang, E. (2001). Support vector machine
active learning for image retrieval. In ACM internatio-
nal conference on Multimedia, pages 107–118. ACM.
Yu, W., Yang, K., Yao, H., Sun, X., and Xu, P. (2017). Ex-
ploiting the complementary strengths of multi-layer
cnn features for image retrieval. Neurocomputing,
237:235–241.
Zha, Z.-J., Yang, L., Mei, T., Wang, M., and Wang, Z.
(2009). Visual query suggestion. In ACM internati-
onal conference on Multimedia, pages 15–24. ACM.
Zhi, T., Duan, L.-Y., Wang, Y., and Huang, T. (2016). Two-
stage pooling of deep convolutional features for image
retrieval. In Image Processing (ICIP), 2016 IEEE In-
ternational Conference on, pages 2465–2469. IEEE.
VISAPP 2018 - International Conference on Computer Vision Theory and Applications
256