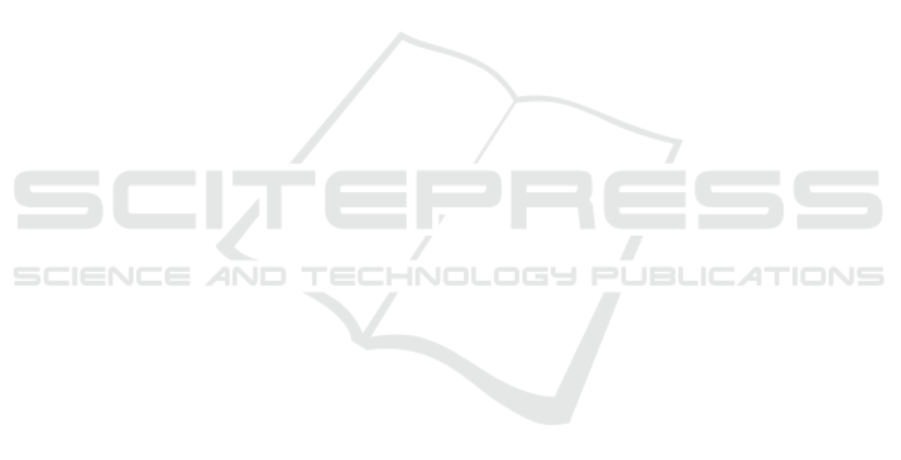
REFERENCES
An, X., Kuang, D., Guo, X., Zhao, Y., and He, L. (2014).
A Deep Learning Method for Classification of EEG
Data Based on Motor Imagery, pages 203–210. Sprin-
ger International Publishing, Cham.
Antoniades, A., Spyrou, L., Took, C. C., and Sanei, S.
(2016). Deep learning for epileptic intracranial eeg
data. In 2016 IEEE 26th International Workshop on
Machine Learning for Signal Processing (MLSP), pa-
ges 1–6.
Bozhkov, L. (2016). Overview of deep learning architectu-
res for classifying brain signals. In KSI Transactions
on Knowledge Society, volume IX, pages 54–59. Kno-
wledge Society Institute.
Deeplearning4j Development Team (2017). Deeplear-
ning4j: Open-source distributed deep learning for the
JVM, Apache Software Foundation License 2.0. [on-
line] available at: http://deeplearning4j.org [Accessed
20 Feb. 2017].
Greaves, A. S. (2014). Classification of EEG with recurrent
neural networks.
Jirayucharoensak, S., Pan-Ngum, S., and Israsena, P.
(2014). EEG-based emotion recognition using deep
learning network with principal component based co-
variate shift adaptation. The Scientific World Journal,
2014.
Lawhern, V. J., Solon, A. J., Waytowich, N. R., Gordon,
S. M., Hung, C. P., and Lance, B. J. (2016). Eegnet: A
compact convolutional network for eeg-based brain-
computer interfaces. CoRR, abs/1611.08024.
LeCun, Y., Bengio, Y., and Hinton, G. (2015). Deep lear-
ning. Nature, 521(7553):436–444.
LeCun, Y. A., Bottou, L., Orr, G. B., and M
¨
uller, K.-
R. (2012). Efficient backprop. In Neural networks:
Tricks of the trade, pages 9–48. Springer.
Srivastava, N., Hinton, G., Krizhevsky, A., Sutskever, I.,
and Salakhutdinov, R. (2014). Dropout: A simple way
to prevent neural networks from overfitting. The Jour-
nal of Machine Learning Research, 15(1):1929–1958.
Stober, S., Sternin, A., Owen, A. M., and Grahn, J. A.
(2015). Deep feature learning for EEG recordings.
arXiv preprint arXiv:1511.04306.
Tabar, Y. R. and Halici, U. (2016). A novel deep learning
approach for classification of EEG motor imagery sig-
nals. Journal of neural engineering, 14(1):016003.
Vallabhaneni, A., Wang, T., and He, B. (2005). Braincom-
puter interface. In Neural engineering, pages 85–121.
Springer.
Va
ˇ
reka, L., Prokop, T., Mou
ˇ
cek, R., Mautner, P., and
ˇ
St
ˇ
ebet
´
ak, J. (2017). Application of Stacked Autoen-
coders to P300 Experimental Data, pages 187–198.
Springer International Publishing, Cham.
Va
ˇ
reka, L. and Mautner, P. (2017). Stacked autoencoders
for the P300 component detection. Frontiers in Neu-
roscience, 11:302.
Va
ˇ
reka, L., Prokop, T.,
ˇ
St
ˇ
ebet
´
ak, J., and Mou
ˇ
cek, R. (2016).
Guess the number - applying a simple brain-computer
interface to school-age children. In Proceedings of
the 9th International Joint Conference on Biomedi-
cal Engineering Systems and Technologies - Volume
4: BIOSIGNALS, (BIOSTEC 2016), pages 263–270.
INSTICC, ScitePress.
Vincent, P., Larochelle, H., Lajoie, I., Bengio, Y., and
Manzagol, P.-A. (2010). Stacked denoising autoen-
coders: Learning useful representations in a deep net-
work with a local denoising criterion. J. Mach. Learn.
Res., 11:3371–3408.
Deep Learning Techniques for Classification of P300 Component
453