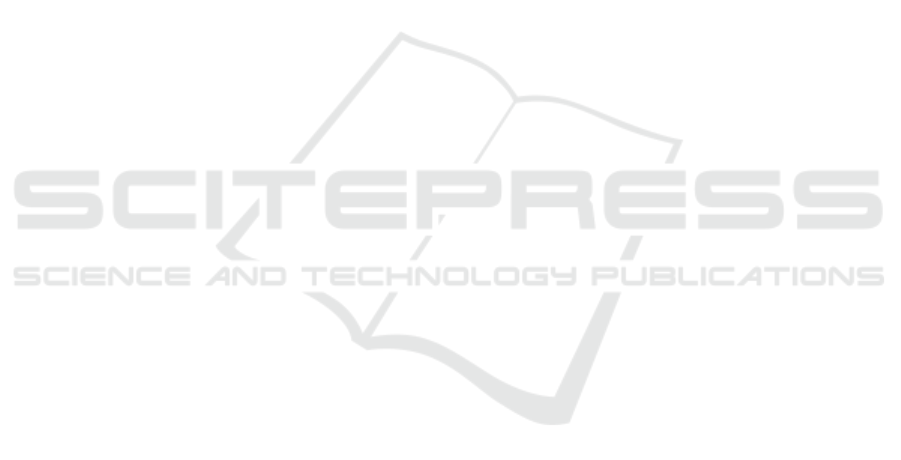
2008). In addition, they observed that the effect of the
temporal delay of feedback depended on the amount
of information that had to be held in working memory
(Lieberman et al., 2008). Despite these observations
pointing towards the importance of minimizing the
temporal delay between brain-activation (and recor-
ding) and projection of feedback, few studies using
neurofeedback address this issue. The present study
demonstrates a highly variable closed-loop system de-
lay depending on the system configuration. These re-
sults show the importance of measuring and reporting
the system delay in order to correctly interpret the be-
havioral effects of neurofeedback training.
5 CONCLUSION
This study shows the importance of testing the system
delay with the final experimental setup before con-
ducting a real-time BCI experiment. We specifically
observe the lowest system delays when streaming a
moderate amount of data through the RNB. A small
amount of data may cause substantially larger system
delays due to inbuilt data aggregation of the software.
REFERENCES
Ang, K. K., Chua, K. S. G., Phua, K. S., Wang, C.,
Chin, Z. Y., Kuah, C. W. K., Low, W., and Guan, C.
(2015). A randomized controlled trial of eeg-based
motor imagery brain-computer interface robotic reha-
bilitation for stroke. Clinical EEG and neuroscience,
46(4):310–320.
Ang, K. K. and Guan, C. (2017). Eeg-based strategies to
detect motor imagery for control and rehabilitation.
IEEE Transactions on Neural Systems and Rehabili-
tation Engineering, 25(4):392–401.
Ang, K. K., Guan, C., Chua, K. S. G., Ang, B. T., Kuah,
C., Wang, C., Phua, K. S., Chin, Z. Y., and Zhang, H.
(2010). Clinical study of neurorehabilitation in stroke
using eeg-based motor imagery brain-computer inter-
face with robotic feedback. In Engineering in Medi-
cine and Biology Society (EMBC), 2010 Annual Inter-
national Conference of the IEEE, pages 5549–5552.
IEEE.
BrainProducts (2015). Getting eeg data in real-time for
bci, neurofeedback and more. [online] Available
at: http://pressrelease.brainproducts.com/real-time-
eeg/ [Accessed 4 sep. 2017].
Daly, J. J., Cheng, R., Rogers, J., Litinas, K., Hrovat, K.,
and Dohring, M. (2009). Feasibility of a new applica-
tion of noninvasive brain computer interface (bci): a
case study of training for recovery of volitional motor
control after stroke. Journal of Neurologic Physical
Therapy, 33(4):203–211.
Darvishi, S., Ridding, M. C., Abbott, D., and Baumert, M.
(2013). Investigation of the trade-off between time
window length, classifier update rate and classifica-
tion accuracy for restorative brain-computer interfa-
ces. In Engineering in Medicine and Biology Society
(EMBC), 2013 35th Annual International Conference
of the IEEE, pages 1567–1570. IEEE.
Gomez-Rodriguez, M., Grosse-Wentrup, M., Hill, J., Gha-
rabaghi, A., Sch
¨
olkopf, B., and Peters, J. (2011). To-
wards brain-robot interfaces in stroke rehabilitation.
In Rehabilitation Robotics (ICORR), 2011 IEEE In-
ternational Conference on, pages 1–6. IEEE.
Kim, T., Zimmerman, P., Wade, M., and Weiss, C. (2005).
The effect of delayed visual feedback on telerobotic
surgery. Surgical Endoscopy and Other Interventional
Techniques, 19(5):683–686.
Lieberman, D. A., Vogel, A. C. M., and Nisbet, J. (2008).
Why do the effects of delaying reinforcement in ani-
mals and delaying feedback in humans differ? a
working-memory analysis. The Quarterly Journal of
Experimental Psychology, 61(2):194–202.
Mohanty, R., Sinha, A., Remsik, A., Allen, J., Nair, V.,
Caldera, K., Sattin, J., Edwards, D., Williams, J. C.,
and Prabhakaran, V. (2017). Machine learning-based
prediction of changes in behavioral outcomes using
functional connectivity and clinical measures in brain-
computer interface stroke rehabilitation. In Internatio-
nal Conference on Augmented Cognition, pages 543–
557. Springer.
Opitz, B., Ferdinand, N. K., and Mecklinger, A. (2011). Ti-
ming matters: the impact of immediate and delayed
feedback on artificial language learning. Frontiers in
human neuroscience, 5.
Ruffino, C., Papaxanthis, C., and Lebon, F. (2017). Neural
plasticity during motor learning with motor imagery
practice: Review and perspectives. Neuroscience,
341:61–78.
Silvoni, S., Ramos-Murguialday, A., Cavinato, M., Volpato,
C., Cisotto, G., Turolla, A., Piccione, F., and Birbau-
mer, N. (2011). Brain-computer interface in stroke: a
review of progress. Clinical EEG and Neuroscience,
42(4):245–252.
Skinner, B. F. (1954). The science of learning and the art of
teaching. Cambridge, Mass, USA, pages 99–113.
Evaluation of Closed-loop Feedback System Delay - A Time-critical Perspective for Neurofeedback Training
193