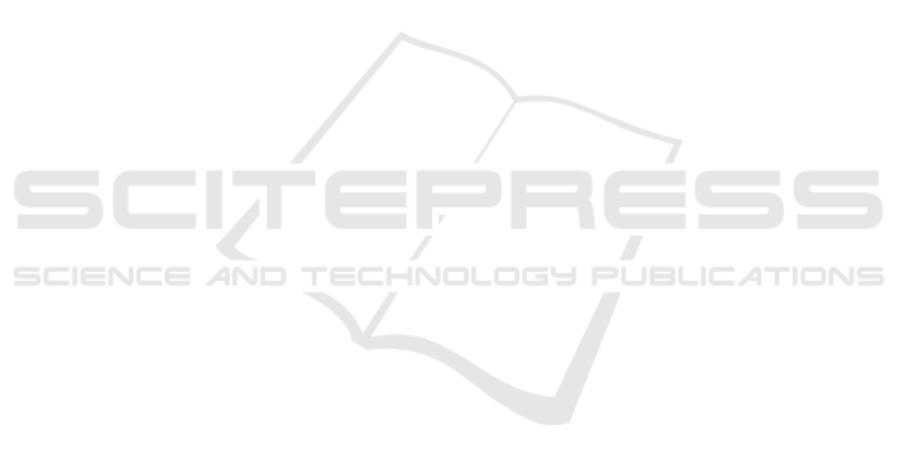
pital patients, to be updated with stay progression,
generating reliable daily risk scores to aid clinical
decision-making and facilitate preventive measures.
ACKNOWLEDGEMENTS
Thomas Hartvigsen thanks the US Department of Ed-
ucation for supporting his PhD studies via the grant
P200A150306 on “GAANN Fellowships to Support
Data-Driven Computing Research”, while Cansu Sen
thanks WPI for granting her the Arvid Anderson
Fellowship (2015-2016) to pursue her PhD studies.
Sarah Brownell thanks the National Science Founda-
tion for undergraduate research funding for Summer
2017 through the NSF REU grant #1560229 entitled
”REU SITE: Data Science Research for Safe, Sustain-
able and Healthy Communities”. We also thank the
DSRG and Data Science Community at WPI for their
continued support and feedback.
REFERENCES
Aureden, K., Arias, K., Burns, L., et al. (2010). Guide
to the elimination of methicillin-resistant staphylococ-
cus aureus (mrsa): Transmission in hospital settings.
washington, dc. APIC.
Breiman, L. (2001). Random forests. Machine learning,
45(1):5–32.
Celi, L. A., Mark, R. G., Stone, D. J., and Montgomery,
R. A. (2013). “big data” in the intensive care unit.
closing the data loop. American Journal of Respira-
tory and Critical Care Medicine, 187(11):1157–1160.
Chang, Y., Yeh, M., Li, Y., Hsu, C., Lin, C., Hsu, M., and
Chiu, W. (2011). Predicting hospital-acquired infec-
tions by scoring system with simple parameters. PloS
One, 6(8):e23137.
CMS (2011). Electronic health records (ehr) incentive pro-
grams. https://www.cms.gov/Regulations-and-Guida
nce/Legislation/EHRIncentivePrograms/index.html.
Congress, U. S. (2009). American recovery and reinvest-
ment act. www.healthit.gov/policy-researchers-
implementers/health-it-legislation.
Dantes, R., Mu, Y., Belflower, R., Aragon, D., Dumyati,
G., Harrison, L. H., Lessa, F. C., Lynfield, R., Nadle,
J., Petit, S., et al. (2013). National burden of inva-
sive methicillin-resistant staphylococcus aureus infec-
tions, united states, 2011. JAMA Internal Medicine,
173(21):1970–1978.
Dubberke, E. R., Yan, Y., Reske, K., Butler, A., Doherty,
J., Pham, V., and Fraser, V. (2011). Development and
validation of a clostridium difficile infection risk pre-
diction model. Infection Control & Hospital Epidemi-
ology, 33(4):360–366.
Dutta, R. and Dutta, R. (2006). Maximum probability rule
based classification of mrsa infections in hospital en-
vironment: Using electronic nose. Sensors and Actu-
ators B: Chemical, 120(1):156–165.
Fukuta, Y., Cunningham, C. A., Harris, P. L., Wagener,
M. M., and Muder, R. R. (2012). Identifying the
risk factors for hospital-acquired methicillin-resistant
staphylococcus aureus (mrsa) infection among pa-
tients colonized with mrsa on admission. Infec-
tion Control & Hospital Epidemiology, 33(12):1219–
1225.
Hajian-Tilaki, K. (2013). Receiver operating characteristic
(roc) curve analysis for medical diagnostic test evalua-
tion. Caspian Journal of Internal Medicine, 4(2):627.
Jensen, P. B., Jensen, L. J., and Brunak, S. (2012). Mining
electronic health records: Towards better research ap-
plications and clinical care. Nature Reviews. Genetics,
13(6):395.
Johnson, A. E., Pollard, T. J., Shen, L., Lehman, L.-w. H.,
Feng, M., Ghassemi, M., Moody, B., Szolovits, P.,
Celi, L. A., and Mark, R. G. (2016). Mimic-iii,
a freely accessible critical care database. Scientific
Data, 3.
Jones, D. A., Shipman, J. P., Plaut, D. A., and Selden, C. R.
(2010). Characteristics of personal health records:
Findings of the medical library association/national
library of medicine joint electronic personal health
record task force. JMLA: Journal of the Medical Li-
brary Association, 98(3):243.
Khalilia, M., Chakraborty, S., and Popescu, M. (2011). Pre-
dicting disease risks from highly imbalanced data us-
ing random forest. BMC Medical Informatics and De-
cision Making, 11(1):51.
Lebedev, A., Westman, E., Van Westen, G., Kramberger,
M., Lundervold, A., Aarsland, D., Soininen, H.,
Kłoszewska, I., Mecocci, P., Tsolaki, M., et al. (2014).
Random forest ensembles for detection and prediction
of alzheimer’s disease with a good between-cohort ro-
bustness. NeuroImage: Clinical, 6:115–125.
Maree, C., Daum, R., Boyle-Vavra, S., Matayoshi,
K., and Miller, L. (2007). Community-associated
methicillin-resistant staphylococcus aureus isolates
and healthcare-associated infections. Emerging Infec-
tious Diseases, 13(2):236.
Murdoch, T. and Detsky, A. (2013). The inevitable applica-
tion of big data to health care. Jama, 309(13):1351–
1352.
Neu, H. C. (1992). The crisis in antibiotic resistance. Sci-
ence, 257(5073):1064–1074.
Nseir, S., Grailles, G., Soury-Lavergne, A., Minacori, F.,
Alves, I., and Durocher, A. (2010). Accuracy of
american thoracic society/infectious diseases society
of america criteria in predicting infection or coloniza-
tion with multidrug-resistant bacteria at intensive-care
unit admission. Clinical Microbiology and Infection,
16(7):902–908.
Raghupathi, W. and Raghupathi, V. (2014). Big data an-
alytics in healthcare: Promise and potential. Health
Information Science and Systems, 2(1):3.
HEALTHINF 2018 - 11th International Conference on Health Informatics
166