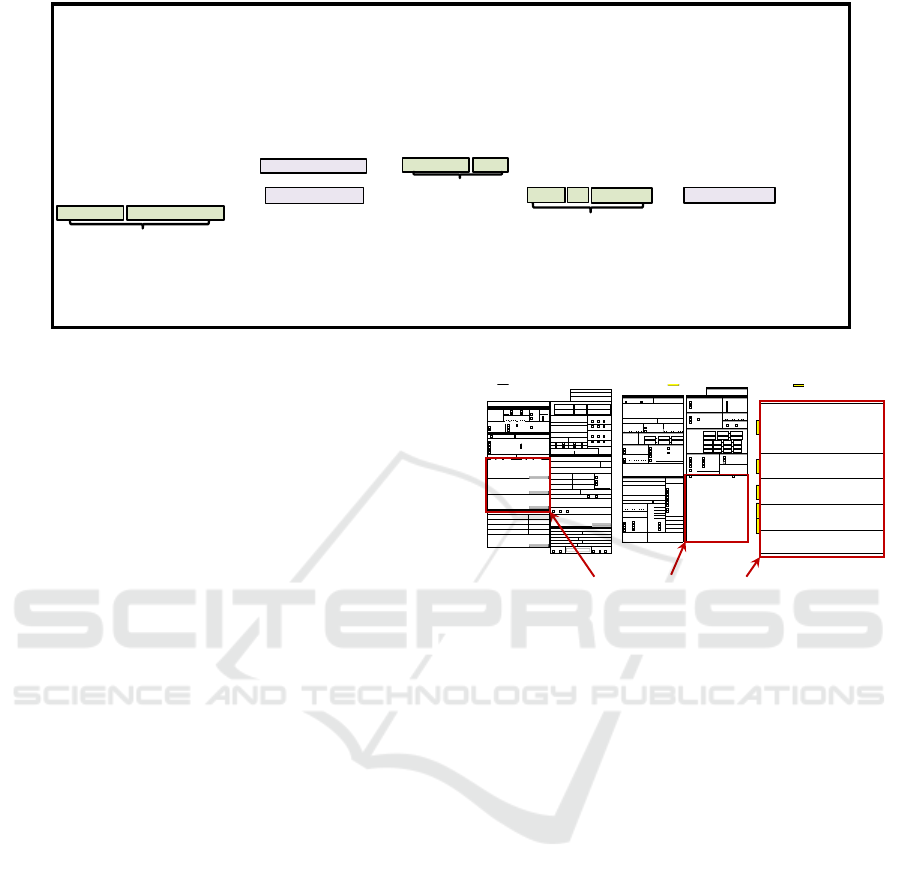
FDA Adverse Event Reporting System (FAERS)
Individual Case Safety Reports (ICSRs)
Event Narrative
This spontaneous report from a patient concerns a XX-year-old Caucasian female from the United States: Local
ID: X-XXXXXXXXXX.
The patient's weight was XXX pounds and height was XX.X inches. Concurrent conditions included abdominal
bloating, abdominal gas, diabetic paresis and type 2 diabetes. The patient had previously experienced
allergy when taking mycins ( a
ntibacterials
for systemic use )and sulfa.
The patient was treated with
canagliflozin
!!
300 mg once a day for type 2 diabetes and domperidone
for
diabetic gastroparesis .
In XXX-XXXX, the patient contacted her physician about the events and was prescribed an increased dosage of
domperidone. The dose of domperidone was increased and the dose of canagliflozin was not changed. The
patient reported the increased dose of domperidone had not relieved her worsening symptoms. The patient had
not recovered from increased belching, worsening of abdominal pain, very gaseous, worsening of bloating, and
not feeling well today. This report was identified by the call center as a product quality complaint.
gastroparesis!diabetic!
systemic! use!
type! 2!
diabetes!
antibacterials!
domperidone!
canagliflozin!
[REASON])
[MEDICATION]!
[REASON])
[REASON])
[MEDICATION]!
[MEDICATION]!
Figure 1: A sample FAERS report highlighting detailed information on the ADR incident within the narrative.
Research (Longadge and Dongre, 2013; Japko-
wicz and Stephen, 2002) has found that, learning on
imbalanced training datasets can cause a significant
deterioration in the performance of the supervised
machine learning methods, particularly when clas-
sifying instances belonging to the under-represented
class.
2. Lack of a Single Best Performing Classification
Method: It is challenging to choose the appropri-
ate learning algorithm to train and classify the new
instances. Conventional approaches to biomedical
NER tend to use a single machine learning method
such as Support Vector Machines (SVM), Conditio-
nal Random Fields (CRF), Maximum Entropy (ME)
(Bishop, 2006) classify named entities in the text.
Each of these methods have some advantages over
the others and differs significantly in their performan-
ces in classifying the named entities. (Uzuner et al.,
2010a) shows that the teams that used different su-
pervised machine learning methods on the same da-
taset obtained significantly different results from one
another. Additionally, the performances of a single
system across the various named entities is shown to
differ. (Uzuner et al., 2010a) concluded that although
the state-of-the-art NLP systems perform well in ex-
tracting some of the named entities (such as medica-
tion, dosages), while other entities (duration, reason
for administration) have shown to be very challen-
ging.
1.4 The Scope of this Work
The general problems of class imbalance and ensem-
ble learning systems for classification have been stu-
died in the literature (Galar et al., 2012). However, in
the context of biomedical NER, a collective approach
to deal with both the class imbalance problem and the
U.S. Department of Health and Human Services
Food and Drug Administration
B. ADVERSE EVENT OR PRODUCT PROBLEM
D. SUSPECT MEDICAL DEVICE
E. INITIAL REPORTER
Form Approved: OMB No. 0910-0291, Expires: 9/30/2018
See PRA statement on reverse.
FORM FDA 3500A (10/15)
Submission of a report does not constitute an admission that medical
personnel, user facility, importer, distributor, manufacturer or product
caused or contributed to the event.
MEDWATCH
For use by user-facilities,
importers, distributors and manufacturers
for MANDATORY reporting
UF/Importer Report #
FDA Use Only
Mfr Report #
PLEASE TYPE OR USE BLACK INK
1.
5. Describe Event or Problem
2. Common Device Name
11. Concomitant Medical Products and Therapy Dates
(Exclude treatment of event)
3. Manufacturer Name, City and State
6. If Implanted, Give Date
(dd-mmm-yyyy)
2. Health
Professional?
8. Is this a single-use device that was
reprocessed and reused on a patient?
9. If Yes to Item 8, Enter Name and Address of Reprocessor
10. Device Available for Evaluation? (Do not send to FDA)
4. Model #
Catalog #
Serial #
6. Relevant Tests/Laboratory Data, Including Dates
1. Brand Name
7. Other Relevant History, Including Preexisting Medical Conditions (e.g.,
allergies, pregnancy, smoking and alcohol use, liver/kidney problems, etc.)
2b. Procode
Lot #
Expiration Date
(dd-mmm-yyyy)
Unique Identifier (UDI) #
5. Operator of Device
7. If Explanted, Give Date
(dd-mmm-yyyy)
3. Occupation (Select from list)
4. Initial Reporter Also Sent
Report to FDA
Adverse Event
and/or
Product Problem (e.g., defects/malfunctions)
Yes
Yes
Yes Yes
No
No Returned to Manufacturer on:
No No Unk
Health
Professional
Lay User/Patient
Other
(Continue on page 3)
(Continue on page 3)
(Continue on page 3)
(Continue on page 3)
A. PATIENT INFORMATION
1. Patient Identifier
In Confidence
2. Age
or Date of Birth (e.g., 08 Feb 1925)
3. Sex 4. Weight
Female
Year(s) Month(s)
Days(s)Week(s)
Male
lb
kg
Note: For date prompts of “dd-mmm-yyyy” please use 2-digit day, 3-letter month
abbreviation, and 4-digit year; for example, 01-Jul-2015.
2. Outcome Attributed to Adverse Event (Check all that apply)
3. Date of Event (dd-mmm-yyyy) 4. Date of this Report (dd-mmm-yyyy)
Death
Life-threatening Disability or Permanent Damage
Congenital Anomaly/Birth DefectsHospitalization – initial or prolonged
Other Serious (Important Medical Events)
Required Intervention to Prevent Permanent Impairment/Damage (Devices)
C. SUSPECT PRODUCT(S)
1. Name, Manufacturer/Compounder, Strength
#1 – Name and Strength
#2 – Name and Strength
2. Concomitant Medical Products and Therapy Dates (Exclude treatment of event)
#1 – NDC # or Unique ID
#2 – NDC # or Unique ID
#1 – Manufacturer/Compounder
#2 – Manufacturer/Compounder
#1 – Lot #
#2 – Lot #
4. Therapy Dates (If unknown, give duration) from/
to (or best estimate)) (dd-mmm-yyyy)
5. Diagnosis for Use (Indication)
8. Expiration Date (dd-mmm-yyyy)
9. Event Abated After Use
Stopped or Dose Reduced?
10. Event Reappeared After
Reintroduction?
Yes
Yes
Yes
Yes
No
No
No
No
Doesn’t
apply
Doesn’t
apply
Doesn’t
apply
Doesn’t
apply
#1
#1
#1
#2
#2
#1 #2
#1
#2
#2
- -
- -
- -- -
- -- -
- -
1. Name and Address
Last Name:
Address:
City:
Country:
Phone #:
First Name:
State/Province/Region:
Email:
ZIP/Postal Code:
5.a. Ethnicity (Check
single best answer)
Hispanic/Latino
Not Hispanic/Latino
5.b. Race (Check all that apply)
Asian American Indian or Alaskan Native
Black or African American White
Native Hawaiian or Other Pacific Islander
Include date (dd-mmm-yyyy):
- -
6. Is the Product
Compounded?
7. Is the Product Over-
the-Counter?
Yes Yes
Yes Yes
No No
No No
#1 #1
#2 #2
3.
Dose Frequency Route Used
#1
#2
Page 1 of 3
-- --
(Continue on page 3)
G. ALL MANUFACTURERS
Health Professional
2. Phone Number
1. Contact Office (and Manufacturing Site for Devices)
Name
3. Report Source
(Check all that apply)
Literature
Foreign
Study
Consumer
Email Address
Compounding Outsourcing Facility 503B?
Address
Yes
Company
Representative
User Facility
Distributor
Other:
ANDA #
10.
Additional Manufacturer Narrative
11.
Corrected Data
and / or
F. FOR USE BY USER FACILITY/IMPORTER (Devices Only)
FDA USE ONLY
H. DEVICE MANUFACTURERS ONLY
3. User Facility or Importer Name/Address
1. Check One 2. UF/Importer Report Number
User Facility
4. Contact Person
6. Date User Facility or
Importer Became Aware
of Event (dd-mmm-yyyy)
(Specify)
7. Type of Report
9. Approximate
Age of Device
Device
Code
10. Event Problem Codes (Refer to coding manual)
Patient
Code
11. Report Sent to FDA? (If Yes,
enter date (dd-mmm-yyyy))
8. Date of This Report
(dd-mmm-yyyy)
12. Location Where Event Occurred
14. Manufacturer Name/Address
5. Phone Number
13. Report Sent to Manufacturer? (If
Yes, enter date (dd-mmm-yyyy))
Outpatient
Diagnostic Facility
Outpatient Treatment
Facility
Conclusions
Nursing Home
Follow-up #
Initial
Other:
NDA #
No
Yes
Evaluation Summary Attached
Home
8. Adverse Event Term(s)
30-day
6. If IND, Give Protocol #
7. Type of Report
(Check all that apply)
4. Date Received by
Manufacturer (dd-mmm-yyyy)
5.
Combination
Product
No
9. Manufacturer Report Number
Yes
5-day
Modification/
Adjustment
7-day
Yes
Yes
10-day
Periodic
PMA/
510(k) #
1. Type of Reportable Event 2. If Follow-up, What Type?
Ambulatory
Surgical Facility
3. Device Evaluated by Manufacturer?
4. Device Manufacture Date
(dd-mmm-yyyy)
5. Labeled for Single Use?
No (Attach page to explain why not) or
provide code:
Importer
No
6. Event Problem and Evaluation Codes (Refer to coding manual)
7. If Remedial Action Initiated, Check Type
Method
9. If action reported to FDA under
21 USC 360i(f), list correction/
removal reporting number:
8. Usage of Device
Yes
Death
Hospital
Notification
Inspection
Patient Monitoring
Results
Yes
Not Returned to Manufacturer
Serious Injury
Malfunction
Relabeling
Correction
Additional Information
Response to FDA Request
Device Evaluation
Initial Use of Device
Reuse
Unknown
Other:
Recall
Repair
Replace
15-day
Pre-1938
BLA #
IND #
FORM FDA 3500A (10/15) (continued)
MEDWATCH
Initial
Follow-up # ____
Device
Code
Patient
Code
Yes
OTC
Department of Health and Human Services
Food and Drug Administration
Office of Chief Information Officer
Paperwork Reduction Act (PRA) Staff
PRAStaff@fda.hhs.gov
This section applies only to requirements of the Paperwork Reduction Act of 1995.
The public reporting burden for this collection of information has been estimated to average 73
minutes per response, including the time for reviewing instructions, searching existing data
sources, gathering and maintaining the data needed, and completing and reviewing the collection
of information. Send comments regarding this burden estimate or any other aspect of this
collection of information, including suggestions for reducing this burden to:
Please DO NOT RETURN this form to the above PRA Staff email address.
OMB Statement: "An agency may not
conduct or sponsor, and a person is not
required to respond to, a collection of
information unless it displays a currently
valid OMB control number."
- - - -
- -
- -
- -
- -
Page 2 of 3
(CONTINUATION PAGE)
For use by user-facilities,
importers, distributors, and manufacturers
for MANDATORY reporting
Concomitant Medical Products and Therapy Dates (Exclude treatment of event) (For continuation of C.2 and/or D.11; please distinguish)
Other Remarks
B.5. Describe Event or Problem (continued)
B.6. Relevant Tests/Laboratory Data, Including Dates (continued)
B.7. Other Relevant History, Including Preexisting Medical Conditions (e.g., allergies, pregnancy, smoking and alcohol use, hepatic/renal dysfunction, etc.) (continued)
FORM FDA 3500A (10/15) (continued)
MEDWATCH
Page 3 of 3
Report Narrative (Free Text)
Figure 2: FAERS report – Medwatch 3500A.
limitations of any one individual classification met-
hod has not been studied extensively. In this paper,
we thus design a novel methodology called Tiered En-
semble Learning System with Diversity (TELS-D) to
address the above challenges in NER. TELS-D invol-
ves four core steps: 1) To address the class imbalance
inherent in medical data used for machine learning
training, we create a balanced training environment
by applying undersampling techniques. 2) We gene-
rate an ensemble of diverse classifiers by training a set
of heterogeneous learning algorithms in this balanced
training environment. 3) We combine the intermedi-
ate results generated by each of the classifiers in the
ensemble to create a meta-training feature set. 4) We
train a “learner-over-learners” meta-algorithm over
the meta-level features to correctly learn and classify
the named entities in the narratives.
To evaluate our model, we perform comprehen-
sive experiments on biomedical reports datasets. Our
experiments demonstrate that our proposed methodo-
logy TELS-D outperforms the individual learners in
the ensemble. TELS-D achieves a higher accuracy
of 0.52 F-measure compared to any of the individual
classifiers with F-measure ranging from 0.22-0.33, in
recognizing the relevant information categories from
the narratives.
HEALTHINF 2018 - 11th International Conference on Health Informatics
178