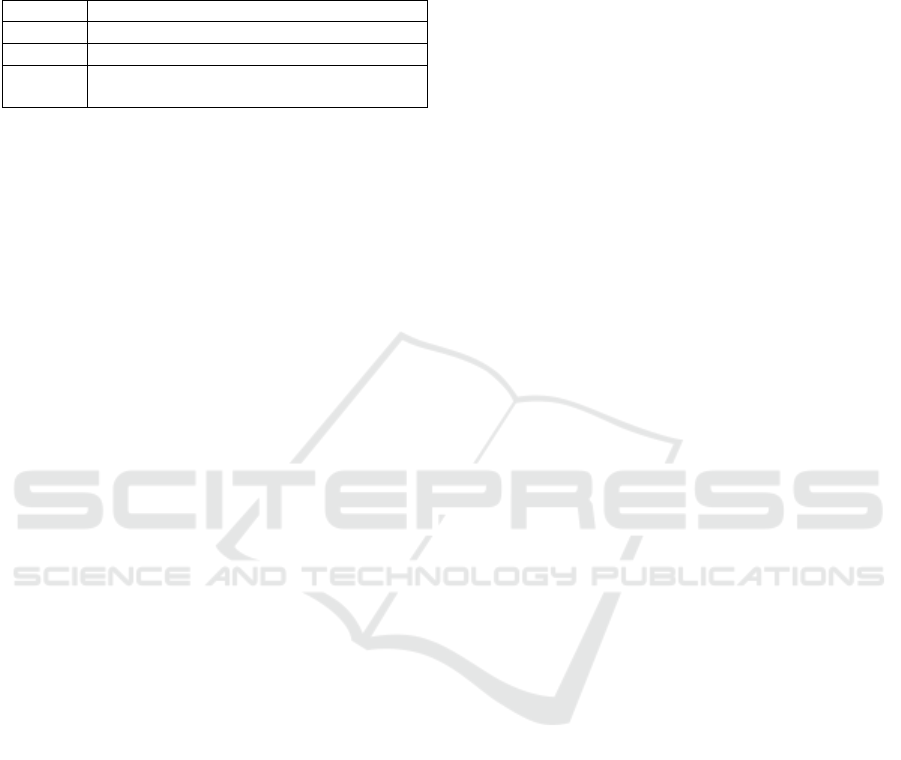
overall speed and speed rhythm (COV). All features
used to evolve the best classifier of each task are
summarised in table 5.
Table 5: Most discriminating features for each task.
(4)(7)(9) (14) from Table 1
(0)(2)(3)(4)(5)(6) (14) from Table 2
(8) (13) (14) (16) (17) (20) from Table 1*
and Table 2
* replaced opening phase with pronation phase and closing
phase with supination phase.
4 CONCLUSIONS
It is clear from the classification results that hand
opening-closing and hand pronation-supination have
the same potential as finger tapping to be used as a
tool in the characterisation of Bradykinesia using GP
to inform clinical assessment. The overall accuracy
was lower than shown in previous studies of GP
classifications using finger tapping data, but we
believe this is due to smaller numbers of subjects.
Almost all classifiers across ten runs for all tasks are
consistent with good accuracies above 70%.
Although the most discriminative movement features
in this study may not be generalised to inform clinical
assessment because of the small sample numbers, it
was demonstrated that by using GP, it could easily be
acquired.
Movement features are computed based on the
current clinical definition of Bradykinesia. However,
CGP has the ability to accept raw positional or speed
data points and perform an unbiased search that will
not be constrained by pre-defined characteristics.
Future work will process PS and HO data using a
sliding window, similar to FT acceleration data in
continuous time series adopted by (Lones et al. 2014).
By using raw data points to induce classifiers, it opens
the possibility of finding new features of
Bradykinesia from the movement tasks.
REFERENCES
Ahmad, A.M. et al., 2012. Breast cancer detection using
cartesian genetic programming evolved artificial neural
networks. Proceedings of the fourteenth international
conference on Genetic and evolutionary computation
conference - GECCO ’12, p.1031.
Ahmad, A.M., Khan, G.M. & Mahmud, S.A., 2013.
Classification of Arrhythmia Types Using Cartesian
Genetic Programming Evolved Artificial Neural
Networks. , pp.282–291.
Christodoulakis, G. et al., 2010. On the filtering and
smoothing of biomechanical data. Communication
Systems Networks and Digital Signal Processing
(CSNDSP), 2010 7th International Symposium on,
pp.512–516.
Dunnewold, R.J., Jacobi, C.E. & van Hilten, J.J., 1997.
Quantitative assessment of bradykinesia in patients
with Parkinson’s disease. Journal of neuroscience
methods, 74, pp.107–112.
Espay, A.J. et al., 2011. Differential response of speed,
amplitude, and rhythm to dopaminergic medications in
Parkinson’s disease. Movement Disorders, 26(14),
pp.2504–2508.
Fawcett, T., 2006. An introduction to ROC analysis.
Pattern Recognition Letters, 27, pp.861–874..
Fernandez, H.H., Machado, A.G. & Pandya, M., 2014. A
Practical Approach to Movement Disorders: Diagnosis
and Management., Demos Medical Publishing.
Goetz, C.G. et al., 2008. Movement Disorder Society-
Sponsored Revision of the Unified Parkinson’s Disease
Rating Scale (MDS-UPDRS): Scale presentation and
clinimetric testing results. Movement Disorders,
23(15), pp.2129–2170.
Hazell, A. & Smith, S.L., 2008. Towards an Objective
Assessment of Alzheimer â€
TM
s Disease : The
Application of a Novel Evolutionary Algorithm in the
Analysis of Figure Copying Tasks. , pp.2073–2079.
Heldman, D. a et al., 2011. The modified bradykinesia
rating scale for Parkinson’s disease: reliability and
comparison with kinematic measures. Movement
disorders : official journal of the Movement Disorder
Society, 26(10), pp.1859–63.
Hope, D.C., Munday, E. & Smith, S.L., 2007. Evolutionary
Algorithms in the Classification of Mammograms.
2007 IEEE Symposium on Computational Intelligence
in Image and Signal Processing, (CIISP), pp.258–265.
Jankovic, J., 2008. Parkinson’s disease: clinical features
and diagnosis. Journal of neurology, neurosurgery, and
psychiatry, 79(4), pp.368–76.
Jobbágy, A. et al., 2005. Analysis of finger-tapping
movement. Journal of neuroscience methods, 141(1),
pp.29–39.
Lacy, S.E., Lones, M. a & Smith, S.L., 2013.
Characterisation of Movement Disorder in Parkinson 's
Disease using Evolutionary Algorithms Categories and
Subject Descriptors., (August 2009), pp.1479–1485.
Ling, H. et al., 2012. Hypokinesia without decrement
distinguishes progressive supranuclear palsy from
Parkinson’s disease. Brain, 135, pp.1141–1153.
Lones, M.A. et al., 2013. Evolving Classifiers to Inform
Clinical Assessment of Parkinson ’ s Disease. , pp.76–
82.
Lones, M.A. et al., 2012. Evolving Classifiers to Recognise
the Movement Characteristics of Parkinson’s Disease
Patients. , pp.1–18.
Lones, M.A. et al., 2014. Evolving Classifiers to Recognize
the Movement Characteristics of Parkinson’s Disease
Patients. , 18(4), pp.559–576.
BIOSIGNALS 2018 - 11th International Conference on Bio-inspired Systems and Signal Processing
68