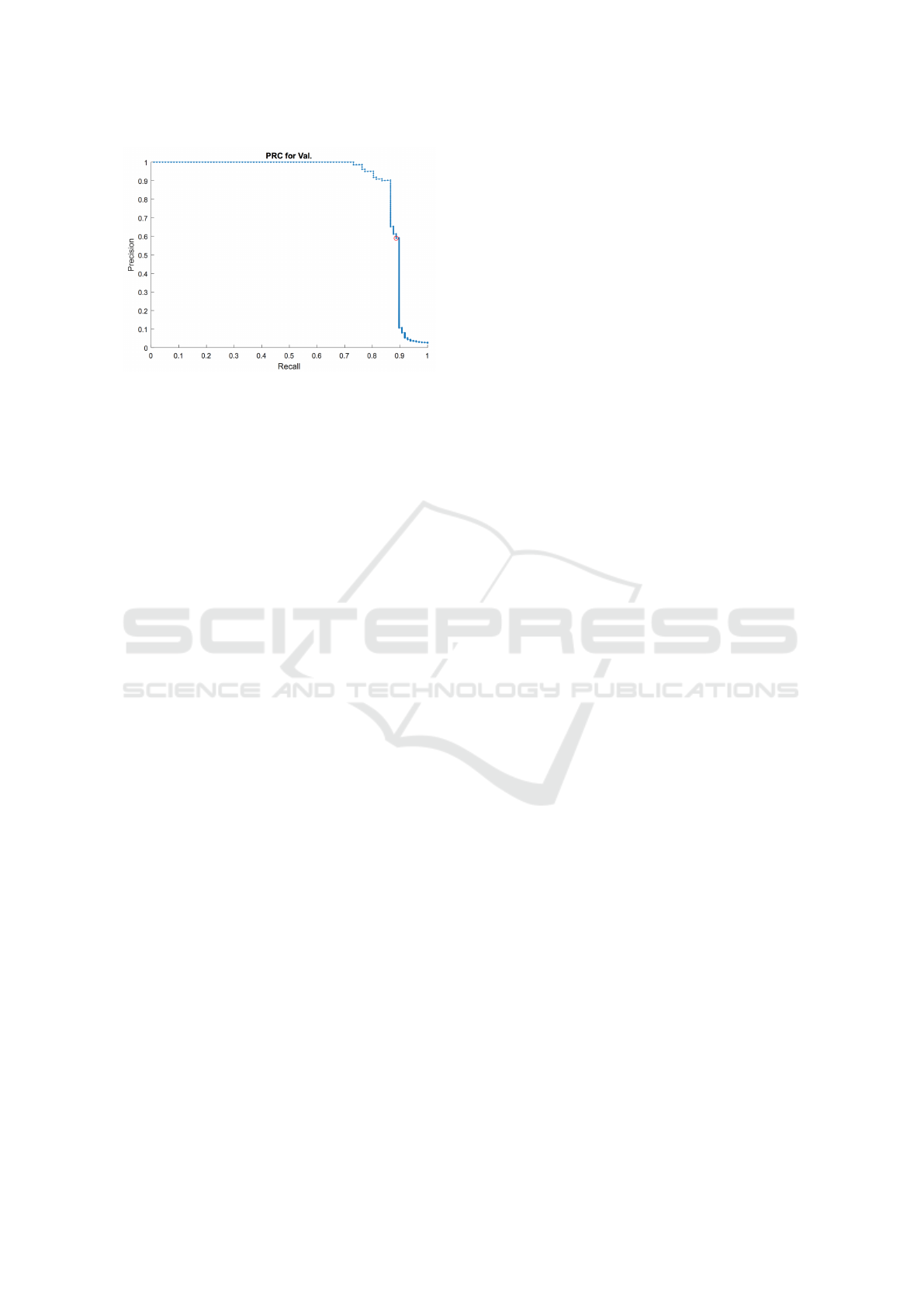
Figure 6: Precision-Recall curve obtained on the validation
set at the first stage and used for the operating point se-
lection. Red point identifies the selected operating point.
4 CONCLUSION
In this paper we have proposed a method for detecting
falls while sporting, with particular reference to the
running. The method is optimized so as to run di-
rectly on board of a wearable embedded device, wit-
hout any additional external server in charge of the
elaboration. The experimental results, conducted over
a dataset made publicly available for benchmarking
purposes, confirm the effectiveness of the proposed
approach, where the possibility of running on embed-
ded devices is not payed in terms of accuracy.
Although the method has been though for de-
tecting falls while running, its architecture is general
enough to also deal with other sports. In the future,
we plan to extend the proposed approach so as to deal
with other typologies of sports. Future works also in-
clude an extension of the dataset and then of the expe-
rimentation, so as to confirm the effectiveness of the
proposed approach.
ACKNOWLEDGEMENTS
This research has been partially supported by
A.I.Tech s.r.l. (www.aitech.vision).
REFERENCES
Abbate, S., Avvenuti, M., Bonatesta, F., Cola, G., Corsini,
P., and Vecchio, A. (2012). A smartphone-based fall
detection system. Pervasive and Mobile Computing,
8(6):883 – 899. Special Issue on Pervasive Healthcare.
Albert, M. V., Kording, K., Herrmann, M., and Jayaraman,
A. (2012). Fall classification by machine learning
using mobile phones. PLOS ONE, 7(5):1–6.
Bagal, F., Becker, C., Cappello, A., Chiari, L., Aminian, K.,
Hausdorff, J. M., Zijlstra, W., and Klenk, J. (2012).
Evaluation of accelerometer-based fall detection algo-
rithms on real-world falls. PLOS ONE, 7(5):1–9.
Bourke, A., van de Ven, P., Gamble, M., OConnor, R.,
Murphy, K., Bogan, E., McQuade, E., Finucane,
P., Laighin, G., and Nelson, J. (2010). Evalua-
tion of waist-mounted tri-axial accelerometer based
fall-detection algorithms during scripted and continu-
ous unscripted activities. Journal of Biomechanics,
43(15):3051 – 3057.
Bourke, A. K. and Lyons, G. M. (2008). A threshold-based
fall-detection algorithm using a bi-axial gyroscope
sensor. Medical Engineering and Physics, 30(1):84–
90.
Bourke, A. K., van de Ven, P. W. J., Chaya, A. E., OLaig-
hin, G. M., and Nelson, J. (2008). Testing of a long-
term fall detection system incorporated into a custom
vest for the elderly. In 2008 30th Annual International
Conference of the IEEE Engineering in Medicine and
Biology Society, pages 2844–2847.
Brun, L., Saggese, A., and Vento, M. (2014). Dynamic
scene understanding for behavior analysis based on
string kernels. IEEE Transactions on Circuits and Sy-
stems for Video Technology, 24(10):1669–1681.
Chen, J., Kwong, K., Chang, D., Luk, J., and Bajcsy, R.
(2005). Wearable sensors for reliable fall detection.
In 2005 IEEE Engineering in Medicine and Biology
27th Annual Conference, pages 3551–3554.
Choi, Y., Ralhan, A. S., and Ko, S. (2011). A study on ma-
chine learning algorithms for fall detection and mo-
vement classification. In 2011 International Confe-
rence on Information Science and Applications, pages
1–8.
Delahoz, Y. S. and Labrador, M. A. (2014). Survey on fall
detection and fall prevention using wearable and ex-
ternal sensors. Sensors, 14(10):19806–19842.
Fahmi, P. N. A., Viet, V., and Deok-Jai, C. (2012). Semi-
supervised fall detection algorithm using fall indica-
tors in smartphone. In Proceedings of the 6th Interna-
tional Conference on Ubiquitous Information Mana-
gement and Communication, pages 122:1–122:9.
Habib, M. A., Mohktar, M. S., Kamaruzzaman, S. B., Lim,
K. S., Pin, T. M., and Ibrahim, F. (2014). Smartphone-
based solutions for fall detection and prevention:
Challenges and open issues. Sensors, 14(4):7181–
7208.
Igual, R., Medrano, C., and Plaza, I. (2013). Challenges, is-
sues and trends in fall detection systems. BioMedical
Engineering OnLine, 12(1):1 – 24.
Jie Yin, J., Qiang Yang, Q., and Pan, J. (2008). Sensor-
Based Abnormal Human-Activity Detection. IEEE
Transactions on Knowledge and Data Engineering,
20(8):1082–1090.
Kalace, A., Fu, D., and Yoshida, S. (2008). Who global
report on falls prevention in older age. World Health
Organization Press: Ginevra, Switzerland.
Khan, S. S. and Hoey, J. (2017). Review of fall detection
techniques: A data availability perspective. Medical
Engineering and Physics, 39:12 – 22.
A Wearable Embedded System for Detecting Accidents while Running
547