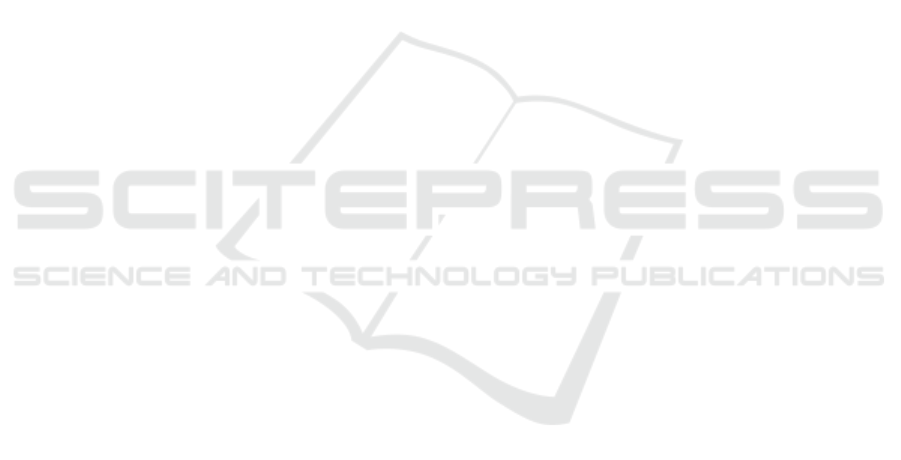
5 CONCLUSIONS
In this paper, we proposed a one-class transfer
learning method named OCSTM, for personalized
anomalous facial expression detection. Unlike other
anomaly detection methods, the OCSTM learns a per-
sonalized model from the target and source samples
by re-weighting the samples based on their proximity
to the target samples. Therefore, re-weighted sam-
ples help the target model to avoid overfitting even
if the sample size of the target samples is small, and
the classifier handles the individual differences appro-
priately. Experiments conducted on UNBC-MSPEA
database show that OCSTM outperforms original
one-class SVM including the generic and single-task
model, and the state-of-the-art ML method. Further-
more, since the selection of feature extraction meth-
ods highly influences the performance of one-class
methods, we investigated suitable features for OC-
STM in anomalous facial expression detection. The
results show that DFL produces higher accuracies
than LBPH and SIFTD because low-dimension fea-
tures, i.e., DFL, contain less irrelevant information
than high-dimension ones, i.e., LBPH and SIFTD.
ACKNOWLEDGEMENTS
This work was partially supported by JSPS KAK-
ENHI Grant Number JP15K12100.
REFERENCES
Ahonen, T., Hadid, A., and Pietik
¨
ainen, M. (2006). Face
Description with Local Binary Patterns: Applica-
tion to Face Recognition. IEEE Trans. on PAMI,
28(12):2037–2041.
Amari, S. and Wu, S. (1999). Improving Support Vector
Machine Classifiers by Modifying Kernel Functions.
Neural Networks, 12(6):783–789.
Chapelle, O. (2007). Training a Support Vector Machine in
the Primal. Neural Computation, 19(5):1155–1178.
Chen, J. and Liu, X. (2014). Transfer Learning with One-
Class Data. Pattern Recognition Letters, 37:32–40.
Chen, J., Liu, X., Tu, P., and Aragones, A. (2013). Learn-
ing Person-Specific Models for Facial Expression and
Action Unit Recognition. Pattern Recognition Letters,
34(15):1964–1970.
Chu, W.-S., Torre, F. D. L., and Cohn, J. F. (2013). Selective
Transfer Machine for Personalized Facial Action Unit
Detection. In CVPR, pages 3515–3522.
Ekman, P. and Friesen, W. V. (1978). Facial Action Coding
System. Consulting Psychologists Press, Palo Alto,
CA.
Gorski, J., Pfeuffer, F., and Klamroth, K. (2007). Bicon-
vex Sets and Optimization with Biconvex Functions:
a Survey and Extensions. Mathematical Methods of
Operations Research, 66(3):373–407.
Gretton, A., Smola, A., Huang, J., Schmittfull, M., Borg-
wardt, K., and Sch
¨
olkopf, B. (2009). Covariate Shift
by Kernel Mean Matching, chapter 8, pages 131–160.
MIT Press, Cambridge, MA.
He, X., Mourot, G., Maquin, D., Ragot, J., Beauseroy,
P., Smolarz, A., and Grall-Ma
¨
es, E. (2014). Multi-
Task Learning with One-Class SVM. Neurocomput-
ing, 133:416–426.
Lucey, P., Cohn, J. F., Prkachin, K. M., Solomon,
P. E., and Matthews, I. (2011). Painful Data: The
UNBC-McMaster Shoulder Pain Expression Archive
Database. In FG, pages 57–64.
Matthews, I. and Baker, S. (2004). Active Appearance
Models Revisited. International Journal of Computer
Vision, 60(2):135–164.
Mohammadian, A., Aghaeinia, H., Towhidkhah, F., et al.
(2016). Subject Adaptation Using Selective Style
Transfer Mapping for Detection of Facial Action
Units. Expert Systems with Applications, 56:282–290.
Prkachin, K. M. and Solomon, P. E. (2008). The Structure,
Reliability and Validity of Pain Expression: Evidence
from Patients with Shoulder Pain. Pain, 139(2):267–
274.
Sangineto, E., Zen, G., Ricci, E., and Sebe, N. (2014). We
are not All Equal: Personalizing Models for Facial Ex-
pression Analysis with Transductive Parameter Trans-
fer. In ACM Multimedia, pages 357–366.
Sch
¨
olkopf, B., Platt, J. C., Shawe-Taylor, J., Smola, A. J.,
and Williamson, R. C. (2001). Estimating the Support
of a High-Dimensional Distribution. Neural Compu-
tation, 13(7):1443–1471.
Shan, C., Gong, S., and McOwan, P. W. (2009). Facial Ex-
pression Recognition Based on Local Binary Patterns:
A Comprehensive Study. Image and Vision Comput-
ing, 27(6):803–816.
Zen, G., Porzi, L., Sangineto, E., Ricci, E., and Sebe, N.
(2016). Learning Personalized Models for Facial Ex-
pression Analysis and Gesture Recognition. IEEE
Trans. on Multimedia, 18(4):775–788.
Zeng, J., Chu, W.-S., Torre, F. D. L., Cohn, J. F., and Xiong,
Z. (2015). Confidence Preserving Machine for Facial
Action Unit Detection. In ICCV, pages 3622–3630.
Zeng, Z., Fu, Y., Roisman, G. I., Wen, Z., Hu, Y., and
Huang, T. S. (2006). One-Class Classification for
Spontaneous Facial Expression Analysis. In FG,
pages 281–286.
Zeng, Z., Pantic, M., Roisman, G. I., and Huang, T. S.
(2009). A Survey of Affect Recognition Methods:
Audio, Visual, and Spontaneous Expressions. IEEE
Trans. on PAMI, 31(1):39–58.
One-class Selective Transfer Machine for Personalized Anomalous Facial Expression Detection
283