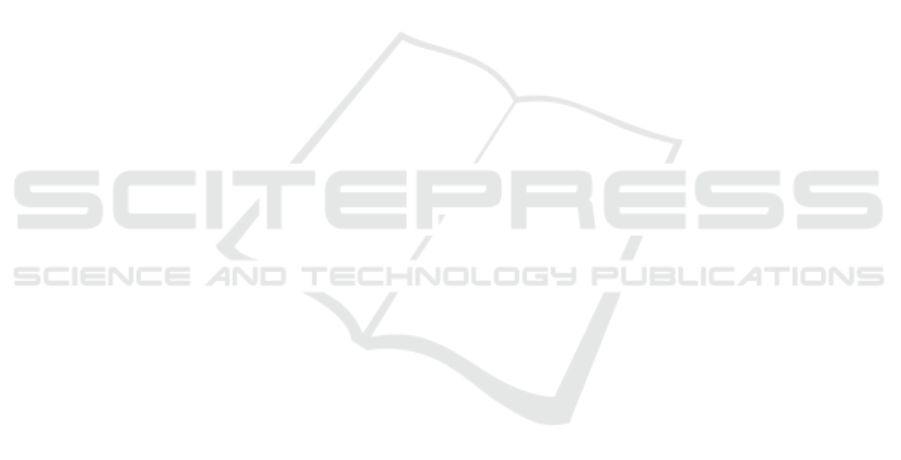
the Minas Gerais Research Foundation – FAPEMIG
(Grants APQ-00567-14 and PPM-00540-17) and the
Coordination for the Improvement of Higher Educa-
tion Personnel – CAPES (DeepEyes Project).
REFERENCES
Bera, A., Kim, S., and Manocha, D. (2016). Realtime
anomaly detection using trajectory-level crowd behav-
ior learning. In CVPRW.
Cao, L., Tian, Y., Liu, Z., Yao, B., Zhang, Z., and Huang,
T. S. (2010). Action detection using multiple spatial-
temporal interest point features. Multimedia and Expo
(ICME), 2010 IEEE International Conference on.
Cheng, K.-W., Chen, Y.-T., and Fang, W.-H. (2015). Video
anomaly detection and localization using hierarchi-
cal feature representation and gaussian process regres-
sion. In CVPR.
Colque, R. V. H. M., Junior, C. A. C., and Schwartz, W. R.
(2015). Histograms of optical flow orientation and
magnitude to detect anomalous events in videos. In
SIBGRAPI.
Crispim, C. F., Koperski, M., Cosar, S., and Bremond, F.
(2016). Semi-supervised understanding of complex
activities from temporal concepts. In AVSS.
Doretto, G., Chiuso, A., Wu, Y. N., and Soatto, S. (2003).
Dynamic textures. International Journal of Computer
Vision.
Eichner, M., Marin-Jimenez, M., Zisserman, A., and Fer-
rari, V. (2012). 2D articulated human pose estimation
and retrieval in (almost) unconstrained still images.
International Journal of Computer Vision.
Fang, Z., Fei, F., Fang, Y., Lee, C., Xiong, N., Shu, L.,
and Chen, S. (2016). Abnormal event detection in
crowded scenes based on deep learning. Multimedia
Tools and Applications.
Feng, Y., Yuan, Y., and Lu, X. (2016). Deep Representation
for Abnormal Event Detection in Crowded Scenes.
Proceedings of the 2016 ACM on Multimedia Confer-
ence.
Hasan, M., Choi, J., Neumann, J., Roy-Chowdhury, A. K.,
and Davis, L. S. (2016). Learning Temporal Regular-
ity in Video Sequences. In CVPR.
Javan Roshtkhari, M. and Levine, M. D. (2013). An on-line,
real-time learning method for detecting anomalies in
videos using spatio-temporal compositions. Comput.
Vis. Image Underst.
Jurafsky, D. and Martin, J. H. (2009). Speech and Language
Processing (2Nd Edition). Prentice-Hall, Inc.
Leyva, R., Sanchez, V., and Li, C. T. (2017). Video anomaly
detection with compact feature sets for online perfor-
mance. IEEE Transactions on Image Processing.
Li, F., Yang, W., and Liao, Q. (2016). An efficient anomaly
detection approach in surveillance video based on ori-
ented GMM. In ICASSP.
Liu, W., Anguelov, D., Erhan, D., Szegedy, C., Reed, S.,
Fu, C.-Y., and Berg, A. C. (2015). SSD: Single shot
multibox detector. arXiv preprint arXiv:1512.02325.
Loy, C. C. (2010). Activity Understanding and Unusual
Event Detection in Surveillance Videos. Queen Mary
University of London.
Mora-Colque, R. V. H., Caetano, C., de Andrade, M. T. L.,
and Schwartz, W. R. (2017). Histograms of optical
flow orientation and magnitude and entropy to detect
anomalous events in videos. IEEE Trans. Circuits
Syst. Video Techn.
Ribeiro, M., Lazzaretti, A. E., and Lopes, H. S. (2017).
A study of deep convolutional auto-encoders for
anomaly detection in videos. Pattern Recognition Let-
ters.
Sabokrou, M., Fayyaz, M., Fathy, M., and Klette, R. (2017).
Deep-cascade: Cascading 3d deep neural networks for
fast anomaly detection and localization in crowded
scenes. IEEE Transactions on Image Processing,
pages 1992–2004.
Wang, J. and Xu, Z. (2016). Spatio-temporal texture mod-
elling for real-time crowd anomaly detection. Com-
puter Vision and Image Understanding.
Xu, D., Ricci, E., Yan, Y., Song, J., and Sebe, N. (2015).
Learning deep representations of appearance and mo-
tion for anomalous event detection. In British Ma-
chine Vision Conference (BMVC).
Xu, D., Ricci, E., Yan, Y., Song, J., and Sebe, N. (2017).
Detecting anomalous events in videos by learning
deep representations of appearance and motion. Com-
put. Vis. Image Underst.
Xu, D., Wu, X., Song, D., Li, N., and Chen, Y.-L. (2013).
Hierarchical activity discovery within spatio-temporal
context for video anomaly detection. In International
Conference on Image Processing (ICIP).
Yu, S. D. X. W. X. (2014). Crowded abnormal detec-
tion based on mixture of kernel dynamic texture. In
ICALIP.
Zhou, S., Shen, W., Zeng, D., Fang, M., Wei, Y., and
Zhang, Z. (2016). Spatial-temporal convolutional neu-
ral networks for anomaly detection and localization in
crowded scenes. Signal Processing: Image Commu-
nication.
VISAPP 2018 - International Conference on Computer Vision Theory and Applications
300