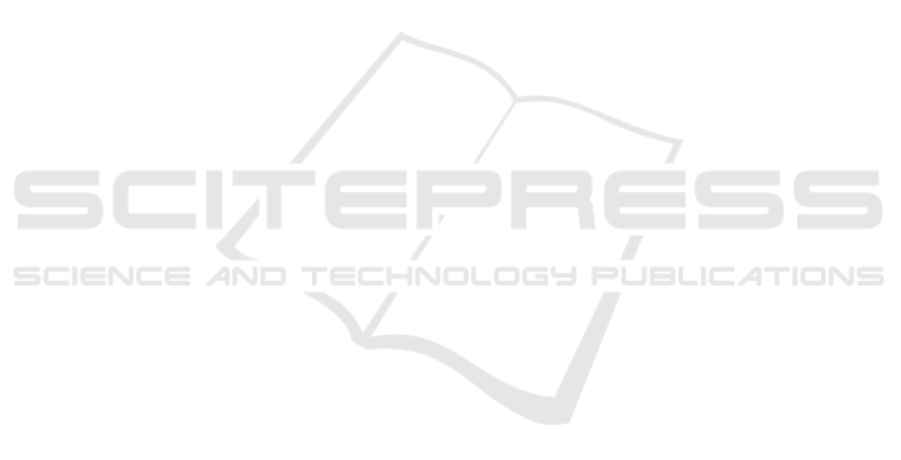
achieves comparable results with OFCM feature de-
scriptor + BoW showing a more compact representa-
tion. In addition, CCW presented better recognition
accuracy when compared to methods of the literature
that also employed co-occurrence to encode informa-
tion on action recognition tasks
Possible directions for future works include to
evaluate other features with CCW representation.
Moreover, we would like to evaluate CCW in other
video-related tasks. It is important to emphasize that,
since the CCWis a spatiotemporal feature representa-
tion, it can be also applied to other computer vision
applications involving video description.
ACKNOWLEDGMENTS
The authors would like to thank the Brazilian National
Research Council – CNPq (Grants #311053/2016-
5 and #449638/2014-6), the Minas Gerais Research
Foundation – FAPEMIG (Grants APQ-00567-14 and
PPM-00540-17) and the Coordination for the Im-
provement of Higher Education Personnel – CAPES
(DeepEyes Project).
REFERENCES
Banerjee, P. and Nevatia, R. (2011). Learning neighborhood
cooccurrence statistics of sparse features for human
activity recognition. In AVSS.
Caetano, C., dos Santos, J. A., and Schwartz, W. R. (2016).
Optical flow co-occurrence matrices: A novel spa-
tiotemporal feature descriptor. In ICPR.
Dalal, N. and Triggs, B. (2005). Histograms of oriented
gradients for human detection. In CVPR.
Dalal, N., Triggs, B., and Schmid, C. (2006). Human de-
tection using oriented histograms of flow and appear-
ance. In ECCV.
Danafar, S. and Gheissari, N. (2007). Action recognition for
surveillance applications using optic flow and svm. In
ACCV.
Haralick, R. M., Shanmugam, K. S., and Dinstein, I.
(1973). Textural features for image classification.
IEEE Transactions on Systems, Man, and Cybernet-
ics.
Howard, A. G., Zhu, M., Chen, B., Kalenichenko, D.,
Wang, W., Weyand, T., Andreetto, M., and Adam,
H. (2017). Mobilenets: Efficient convolutional neu-
ral networks for mobile vision applications. CoRR.
Keval, H. (2006). Cctv control room collaboration and com-
munication: Does it work? In Human Centred Tech-
nology Workshop.
Kl
¨
aser, A., Marszałek, M., and Schmid, C. (2008). A spatio-
temporal descriptor based on 3d-gradients. In BMVC.
Krig, S. (2014). Interest point detector and feature descrip-
tor survey. In Computer Vision Metrics. Apress.
Kuehne, H., Jhuang, H., Garrote, E., Poggio, T., and Serre,
T. (2011). Hmdb: A large video database for human
motion recognition. In ICCV.
Laptev, I. (2005). On space-time interest points. IJCV.
Laptev, I., Marszalek, M., Schmid, C., and Rozenfeld,
B. (2008). Learning realistic human actions from
movies. In CVPR.
Liu, D., Hua, G., Viola, P., and Chen, T. (2008). Integrated
feature selection and higher-order spatial feature ex-
traction for object categorization. In CVPR.
Liu, H., Liu, M., and Sun, Q. (2014). Learning direc-
tional co-occurrence for human action classification.
In ICASSP.
Nosaka, R., Ohkawa, Y., and Fukui, K. (2012). Feature ex-
traction based on co-occurrence of adjacent local bi-
nary patterns. In PSIVT.
Poppe, R. (2010). A survey on vision-based human action
recognition. Image Vision Comput.
Reddy, V., Sanderson, C., and Lovell, B. (2011). Improved
anomaly detection in crowded scenes via cell-based
analysis of foreground speed, size and texture. In
CVPRW.
Rodriguez, M., Ahmed, J., and Shah, M. (2008). Action
mach a spatio-temporal maximum average correlation
height filter for action recognition. In CVPR.
Schuldt, C., Laptev, I., and Caputo, B. (2004). Recognizing
human actions: A local svm approach. In ICPR.
Shi, F., Laganiere, R., and Petriu, E. (2015). Gradient
boundary histograms for action recognition. In WACV.
Sivic, J. and Zisserman, A. (2003). Video Google: A text
retrieval approach to object matching in videos. In
ICCV.
Sun, Q. and Liu, H. (2013). Learning spatio-temporal co-
occurrence correlograms for efficient human action
classification. In ICIP.
Wang, H., Klaser, A., Schmid, C., and Liu, C.-L. (2011).
Action recognition by dense trajectories. In CVPR.
Wang, H., Ullah, M. M., Klaser, A., Laptev, I., and Schmid,
C. (2009). Evaluation of local spatio-temporal fea-
tures for action recognition. In BMVC.
Wiliem, A., Madasu, V., Boles, W., and Yarlagadda, P.
(2012). A suspicious behaviour detection using a
context space model for smart surveillance systems.
CVIU.
Xiang, T. and Gong, S. (2008). Video behavior profiling for
anomaly detection. TPAMI.
Yang, Y. and Newsam, S. (2011). Spatial pyramid co-
occurrence for image classification. In ICCV.
Zalevsky, Z., Rivlin, E., and Rudzsky, M. (2005). Motion
characterization from co-occurrence vector descriptor.
PRL.
Zhang, L., Zhen, X., and Shao, L. (2012). High order co-
occurrence of visualwords for action recognition. In
ICIP.
Zhang, Z., Chen, Y., and Saligrama, V. (2014). A
novel visual word co-occurrence model for person re-
identification. In ECCVW.
Zhang, Z. and Saligrama, V. (2017). Prism: Person reiden-
tification via structured matching. TCSVT.
VISAPP 2018 - International Conference on Computer Vision Theory and Applications
308