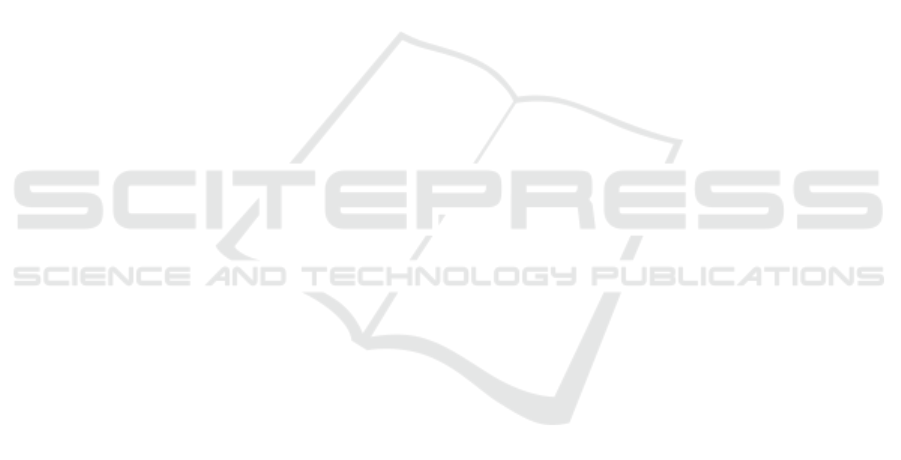
Verification: A conducive case for verification is
the creation of comments, containing insights and/or
findings as depicted in (f), highlighted in orange. The
annotated text is shown with supplementary informa-
tion like author, date, time, and certainty level. Other
experts have the possibility to vote on that comment
and add additional comments themselves. By linking
these additional comments a communication between
the experts is possible.
Knowledge Generation: To support knowledge gen-
eration all the comments from (f) can be collected and
visualized. Ordered by certainty value, and/or agree-
ment level users can view and appraise the consoli-
dated result. That gives an overview on the gained in-
sights and findings including the discussion between
the experts. A fitting visualization is the use of visual
separation, to obviously show that the annotations are
now in the center of the analysis, similar to the work
of (Zhao et al., 2017).
5 CONCLUSION AND FUTURE
WORK
We have shown that annotations can be characterized
and conveniently integrated into the knowledge gen-
eration model from (Sacha et al., 2014). The next step
will be the continuing evaluation by implementing our
projected use case. The examples for practical use
given in this paper, are only a small extract of the pos-
sibilities. Ongoing effort must be spent on analyzing
the effects of other annotation characteristics on the
visual analysis. That particularly applies to automat-
ically derived annotations, as this work concentrates
on the manually gathered amendments. Additionally,
more research effort must be invested into the clar-
ification of questions as ”How can we store annota-
tions?” and ”How can we integrate annotations into
the data space?”.
ACKNOWLEDGEMENTS
This work has been supported by the German Fed-
eral Ministry of Education and Research. Christoph
Schmidt has been supported by the project TOPOs.
REFERENCES
Alm, R., Aehnelt, M., and Urban, B. (2015). Processing
manufacturing knowledge with ontology-based anno-
tations and cognitive architectures. In Proceedings
of the 15th International Conference on Knowledge
Technologies and Data-driven Business - i-KNOW.
Association for Computing Machinery (ACM).
Callahan, S. P., Freire, J., Santos, E., Scheidegger, C. E.,
Silva, C. T., and Vo, H. T. (2006). Vistrails: Visu-
alization meets data management. In Proceedings of
the 2006 ACM SIGMOD International Conference on
Management of Data, New York, NY, USA.
Chang, E., Goh, K., Sychay, G., and Wu, G. (2003). CBSA:
content-based soft annotation for multimodal image
retrieval using bayes point machines. IEEE Transac-
tions on Circuits and Systems for Video Technology.
Fromont, R. (2017). Toward a format-neutral annotation
store. Computer Speech & Language.
Groth, D. and Streefkerk, K. (2006). Provenance and anno-
tation for visual exploration systems. IEEE Transac-
tions on Visualization and Computer Graphics.
Heer, J. and Shneiderman, B. (2012). Interactive dynamics
for visual analysis. Queue.
Heer, J., Vi
´
egas, F. B., and Wattenberg, M. (2007). Voy-
agers and voyeurs: Supporting asynchronous collabo-
rative information visualization. In Proceedings of the
SIGCHI Conference on Human Factors in Computing
Systems.
M, S. K. and Wilson, J. (2015). A novel method for
automatic discovery, annotation and interactive visu-
alization of prominent clusters in mobile subscriber
datasets. In 2015 IEEE 9th International Confer-
ence on Research Challenges in Information Science
(RCIS).
Mahyar, N., Sarvghad, A., and Tory, M. (2012). Note-
taking in co-located collaborative visual analytics:
Analysis of an observational study. Information Vi-
sualization.
R
¨
ohlig, M., Stachs, O., and Schumann, H. (2016). Detec-
tion of Diabetic Neuropathy - Can Visual Analytics
Methods Really Help in Practice? In EuroVis Work-
shop on Reproducibility, Verification, and Validation
in Visualization (EuroRV3).
Rosenthal, P., Ritter, M., Kowerko, D., and Heine, C.
(2016). Ophthalvis - making data analytics of optical
coherence tomography reproducible. In Proceedings
of EuroRV3, the EuroVis Workshop on Reproducibil-
ity, Verification, and Validation in Visualization.
Sacha, D., Stoffel, A., Stoffel, F., Kwon, B. C., Ellis, G., and
Keim, D. A. (2014). Knowledge generation model for
visual analytics. IEEE Transactions on Visualization
and Computer Graphics.
Sacha, D., Zhang, L., Sedlmair, M., Lee, J. A., Peltonen, J.,
Weiskopf, D., North, S. C., and Keim, D. A. (2017).
Visual interaction with dimensionality reduction: A
structured literature analysis. IEEE Transactions on
Visualization and Computer Graphics.
Saur
´
ı, R. (2017). Building FactBank or How to Annotate
Event Factuality One Step at a Time, pages 905–939.
Springer Netherlands, Dordrecht.
von Ahn, L., Maurer, B., McMillen, C., Abraham, D., and
Blum, M. (2008). recaptcha: Human-based character
recognition via web security measures. Science, pages
1465–1468.
Annotations as a Support for Knowledge Generation - Supporting Visual Analytics in the Field of Ophthalmology
271