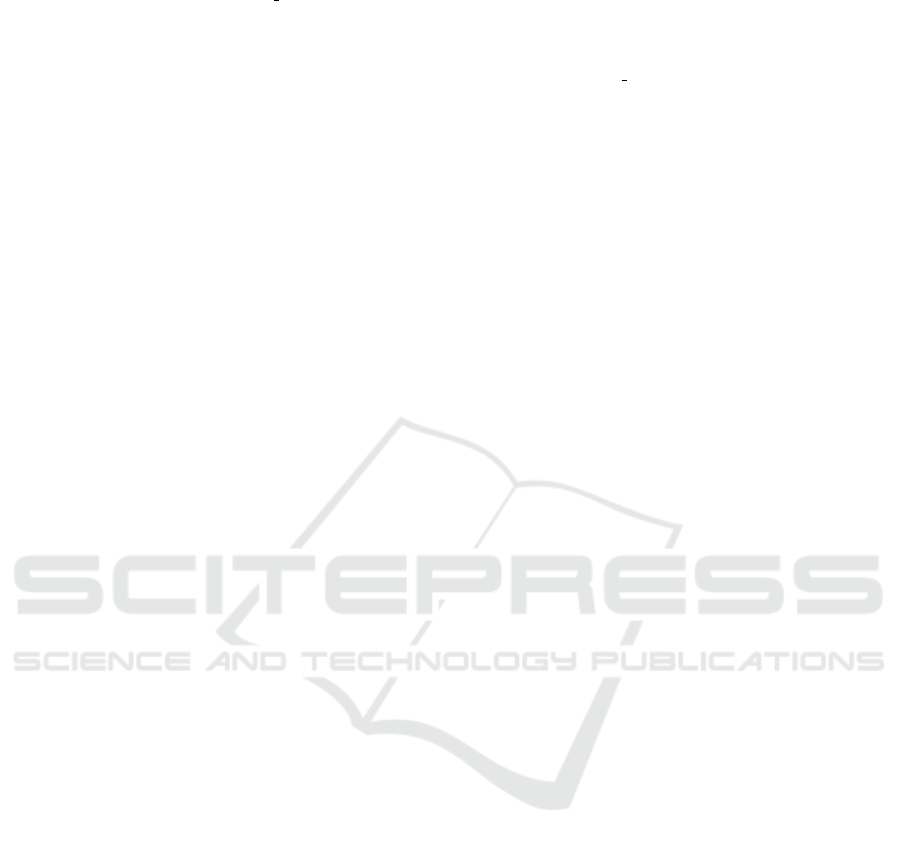
doi:10.1109/CVPR.2011.5995637. Retrieved from
http://ivrl.epfl.ch/supplementary material/cvpr11/.
Burger, H. C., Schuler, C. J., and Harmeling, S. (2012).
Image denoising: Can plain neural networks compete
with BM3D? In IEEE Conference on Computer Vi-
sion and Pattern Recognition, 2392-2399. IEEE. doi:
10.1109/CVPR.2012.6247952.
Dabov, K., Foi, A., Katkovnik, V., and Egiazarian, K.
(2007). Image denoising by sparse 3-d transform-
domain collaborative filtering. In IEEE Transac-
tions on Image Processing, 16(8), 2080-2095. IEEE.
doi:10.1109/TIP.2007.901238.
Dong, C., Loy, C. C., He, K., and Tang, X. (2015). Im-
age super-resolution using deep convolutional net-
works. In IEEE Transactions on Pattern Analy-
sis and Machine Intelligence, 38(2), 295-307. IEEE.
doi:10.1109/TPAMI.2015.2439281.
Elad, M. and Aharon, M. (2006). Image denoising via
learned dictionaries and sparse representation. In
IEEE Computer Society Conference on Computer Vi-
sion and Pattern Recognition (CVPR), 1, 895-900.
IEEE. doi:10.1109/CVPR.2006.142.
Glorot, X. and Bengio, Y. (2010). Understanding the
difficulty of training deep feedforward neural net-
works. In Proceedings of the Thirteenth Inter-
national Conference on Artificial Intelligence and
Statistics, 9, 249-256. JMLR. Retrieved from
http://proceedings.mlr.press/v9/glorot10a.html.
Goldstein, T. and Osher, S. (2009). The split breg-
man method for L1 regularized problems. In SIAM
Journal on Imaging Sciences, 2(2), 323-343. Society
for Industrial and Applied Mathematics Philadelphia.
doi:10.1137/080725891.
He, K., Zhang, X., Ren, S., and Sun, J. (2016). Deep
residual learning for image recognition. In IEEE Con-
ference on Computer Vision and Pattern Recognition
(CVPR), 770-778. IEEE. doi:10.1109/CVPR.2016.90.
Jain, V. and Seung, S. (2009). Natural image de-
noising with convolutional networks. In Ad-
vances in Neural Information Processing Systems
21, 769-776. Curran Associates, Inc. Retrieved
from http://papers.nips.cc/paper/3506-natural-image-
denoising-with-convolutional-networks.pdf.
J.Xu, L.Zhang, Zuo, W., Zhang, D., and Feng, X. (2015).
Patch group based nonlocal self-similarity prior learn-
ing for image denoising. In IEEE International Con-
ference on Computer Vision (ICCV), 244-252. IEEE.
doi:10.1109/ICCV.2015.36.
Kim, J., Lee, J. K., and Lee, K. M. (2016). Accurate
image super-resolution using very deep convolutional
networks. In IEEE Computer Society Conference on
Computer Vision and Pattern Recognition (CVPR),
1646-1654. IEEE. doi:10.1109/CVPR.2016.182.
Kingma, D. P. and Ba, J. (2014). Adam: A method for
stochastic optimization. In 3rd International Confer-
ence for Learning Representations. CoRR. Retrieved
from http://arxiv.org/abs/1412.6980.
Micchelli, C. A., Shen, L., and Y.Xu (2011). Prox-
imity algorithms for image models: denoising.
In Inverse Problems, 27(4). Retrieved from
http://stacks.iop.org/0266-5611/27/i=4/a=045009.
Pan, T., Zhongliang, F., Lili, W., and Kai, Z. (2016).
Perceptual loss with fully convolutional for image
residual denoising. In T. Tan et al. (eds.) Chinese
Conference on Pattern Recognition (CCPR), 122-132.
Springer. Retrieved from https://doi.org/10.1007/978-
981-10-3005-5 11.
Vedaldi, A. and Lenc, K. (2015). Matconvnet: Con-
volutional neural networks for matlab. In Pro-
ceedings of the 23rd ACM international confer-
ence on Multimedia. CoRR. Retrieved from
http://arxiv.org/abs/1412.4564.
Zhang, K., Zuo, W., Chen, Y., Meng, D., and Zhang, L.
(2017). Beyond a gaussian denoiser: Residual learn-
ing of deep cnn for image denoising. In IEEE Transac-
tions on Image Processing, 26(7), 3142-3155. IEEE.
doi:10.1109/TIP.2017.2662206.
Zhang, Z. M., Tsai, B. K., and G.Machin (2010). Radiomet-
ric Temperature Measurements. Elsevier, 1st edition.
Zhou, Z., Cao, J., and Liu, W. (2009). Contourlet-
based image denoising algorithm using adaptive win-
dows. In 4th IEEE Conference on Industrial Elec-
tronics and Applications (ICIEA), 3654-3657. IEEE.
doi:10.1109/ICIEA.2009.5138888.
VISAPP 2018 - International Conference on Computer Vision Theory and Applications
46