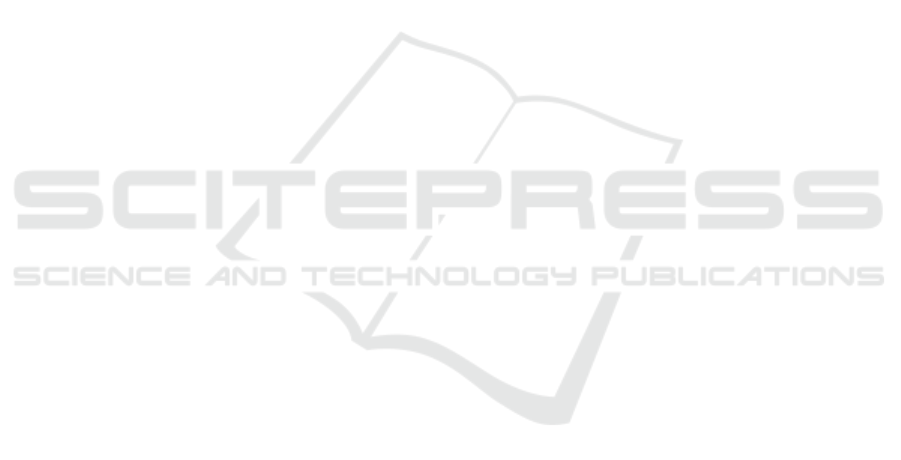
4 CONCLUSION AND
DISCUSSION
We first compared eye and mouse data with the sa-
liency metric PCC. We did not find significant con-
sistency between participants’ eyes and mouse positi-
ons (inter) and between participants’ eyes (intra). Ho-
wever, results showed that participants behaved in a
more similar way when they had the same task with
the same location (reading task).
Then, we got deeper with dynamic analyses. We
showed that using distance and correlation, we were
able to highlight more interesting coordinations bet-
ween eyes and mouse. We had better results on Y axis
than X axis and succeed to demonstrate behaviour dif-
ferences between tasks. In addition, scroll analyses,
clearly showed a relation between eyes position and
scroll speed while browsing and amplitude before the
scroll.
Finally, we made a model for each task able to
predict the area around the mouse’s cursor in which
the eyes had 70% chances to be located in. Howe-
ver, eyes location uncertainty compared to mouse po-
sition remained high, even if we succeed to enhance
the model during target finding task by observing bru-
tal changes on X axis.
In this paper, we presented results of a prelimi-
nary study, used as a validation to conduct a bigger
experiment, including more participants. This will al-
low us to analyse the impact of participants’ age on
their mouse movements. Moreover, we did not use
scroll events analyses to enhance our models. In fu-
ture work, we think that doing so, could boost the
precision of the model by reducing the area around
the mouse’s cursor. We could also investigate new re-
lations between the scroll and the eyes by analysing
scroll in 2D. Then, we could use machine learning
models to integrate new features and more user beha-
viours such as mouse patterns. Finally, our main ob-
jective is to propose the most accurate model in order
to use it in real time to predict web user behaviours.
ACKNOWLEDGEMENT
We thank French Research and Technology Associa-
tion (ANRT) and Sublime Skinz for supporting this
work.
REFERENCES
Bejan, A. (2009). The goldenratio predicted: Vision, cog-
nition and locomotion as a single design in nature. In-
ternational Journal of Design & Nature and Ecodyn-
amics, 4(2):97–104.
Boi, P., Fenu, G., Davide Spano, L., and Vargiu, V. (2016).
Reconstructing User’s Attention on the Web through
Mouse Movements and Perception-Based Content
Identification. Transactions on Applied Perception,
13(3):1–21.
Castelhano, M. S., Mack, M. L., and Henderson, J. M.
(2009). Viewing task influences eye movement cont-
rol during active scene perception. Journal of Vision,
9(3):1–15.
Chen, M.-c. (2001). What can a mouse cursor tell us more?
Correlation of eye / mouse movements on web brow-
sing. In Conference on Human Factors in Computing
Systems, pages 281–282.
Cooke, L. (2006). Is the Mouse a” Poor Man’s Eye Trac-
ker”? Annual Conference-Society for Technical Com-
munication, 53:252 – 255.
Deng, S., Chang, J., Kirkby, J. A., and Zhang, J. J. (2016).
Gaze–mouse coordinated movements and dependency
with coordination demands in tracing. Behaviour &
Information Technology, 35(8):665–679.
Guo, Q. and Agichtein, E. (2010). Towards predicting web
searcher gaze position from mouse movements. In Ex-
tended Abstracts of Conference on Human Factors in
Computing Systems, pages 3601–3606.
Huang, J. and White, R. (2012). User See, User Point: Gaze
and Cursor Alignment in Web Search. In Special Inte-
rest Group of Conference on Human Factors in Com-
puting Systems, pages 1341–1350.
Le Meur, O., Le Callet, P., and Barba, D. (2007). Predicting
visual fixations on video based on low-level visual fe-
atures. Vision Research, 47(19):2483–2498.
Mills, M., Hollingworth, A., and Dodd, M. D. (2011). Ex-
amining the influence of task set on eye movements
and fi xations. Journal of Vision, 11(8):1–15.
Mueller, F. and Lockerd, A. (2001). Cheese: tracking
mouse movement activity on websites, a tool for user
modeling. In Extended Abstracts of Conference on
Human Factors in Computing Systems, pages 279–
280.
Navalpakkam, V., Jentzsch, L. L., Sayres, R., Ravi, S.,
Ahmed, A., and Smola, A. (2013). Measurement and
modeling of eye-mouse behavior in the presence of
nonlinear page layouts. In International Conference
on World Wide Web, pages 953–964.
Ouerhani, N., Wartburg, R. V., and Heinz, H. (2004). Empi-
rical Validation of the Saliency-based Model of Visual
Attention. Electronic Letters on Computer Vision and
Image Analysis, 3(1):13–24.
Rodden, K. and Fu, X. (2007). Exploring how mouse mo-
vements relate to eye movements on web search re-
sults pages. In Special Interest Group on Information
Retrieval Workshop on Web Information Seeking and
Interaction, pages 29–32.
Rodden, K., Fu, X., Aula, A., and Spiro, I. (2008). Eye-
mouse coordination patterns on web search results pa-
ges. In Extended Abstracts of Conference on Human
Factors in Computing Systems, pages 2997–3002.
HUCAPP 2018 - International Conference on Human Computer Interaction Theory and Applications
92