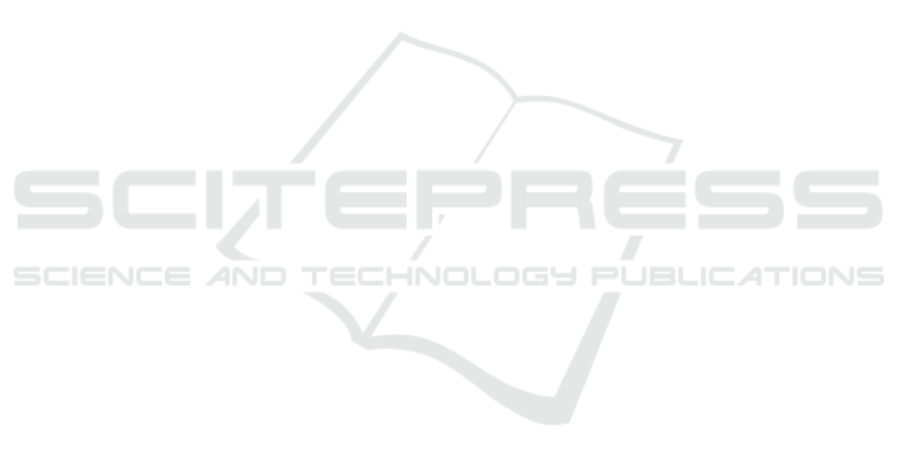
reliable solution with remarkable results. The pro-
posed approach requires a relatively small training ef-
fort, while it is able to achieve a state-of-the-art per-
formance on the ModelNet dataset. In the current ap-
proach, accuracies reported for volumetric and curva-
ture descriptors are slightly lower than those obtained
with depth data, future work will be devoted to im-
prove their performance as well. Furthermore, we
will explore the possibility of using more advanced
deep learning schemes and different approaches to
combine the multiple information sources.
REFERENCES
Bai, S., Bai, X., Zhou, Z., Zhang, Z., and Jan Latecki, L.
(2016). Gift: A real-time and scalable 3d shape search
engine. In Proceedings of CVPR, pages 5023–5032.
Brock, A., Lim, T., Ritchie, J. M., and Weston, N.
(2016). Generative and discriminative voxel modeling
with convolutional neural networks. arXiv preprint
arXiv:1608.04236.
Couprie, C., Farabet, C., Najman, L., and LeCun, Y. (2013).
Indoor semantic segmentation using depth informa-
tion. In Int. Conf. on Learning Representations.
Farabet, C., Couprie, C., Najman, L., and LeCun, Y.
(2013). Learning hierarchical features for scene la-
beling. IEEE Transactions on Pattern Analysis and
Machine Intelligence, 35(8):1915–1929.
Garcia-Garcia, A., Gomez-Donoso, F., Garcia-Rodriguez,
J., Orts-Escolano, S., Cazorla, M., and Azorin-Lopez,
J. (2016). Pointnet: A 3d convolutional neural net-
work for real-time object class recognition. In Pro-
ceedings of IJCNN, pages 1578–1584. IEEE.
Guo, Y., Bennamoun, M., Sohel, F., Lu, M., and Wan,
J. (2014). 3d object recognition in cluttered scenes
with local surface features: A survey. IEEE Trans-
actions on Pattern Analysis and Machine Intelligence,
36(11):2270–2287.
Hegde, V. and Zadeh, R. (2016). Fusionnet: 3d object clas-
sification using multiple data representations. arXiv
preprint arXiv:1607.05695.
Johns, E., Leutenegger, S., and Davison, A. J. (2016).
Pairwise decomposition of image sequences for ac-
tive multi-view recognition. In Proceedings of CVPR,
pages 3813–3822.
Klokov, R. and Lempitsky, V. (2017). Escape from cells:
Deep kd-networks for the recognition of 3d point
cloud models. arXiv preprint arXiv:1704.01222.
Li, B., Lu, Y., Li, C., Godil, A., Schreck, T., Aono, M.,
Burtscher, M., Chen, Q., Chowdhury, N. K., Fang,
B., et al. (2015). A comparison of 3d shape retrieval
methods based on a large-scale benchmark supporting
multimodal queries. Computer Vision and Image Un-
derstanding, 131:1–27.
Li, Y., Pirk, S., Su, H., Qi, C. R., and Guibas, L. J. (2016).
Fpnn: Field probing neural networks for 3d data. In
Advances in Neural Information Processing Systems.
Maturana, D. and Scherer, S. (2015). Voxnet: A 3d convolu-
tional neural network for real-time object recognition.
In International Conference on Intelligent Robots and
Systems (IROS), pages 922–928. IEEE.
Minto, L., Pagnutti, G., and Zanuttigh, P. (2016). Scene seg-
mentation driven by deep learning and surface fitting.
In Geometry Meets Deep Learning ECCV Workshop.
Pagnutti, G. and Zanuttigh, P. (2016). Joint color and depth
segmentation based on region merging and surface fit-
ting. In Proceedings of VISAPP.
Qi, C. R., Su, H., Mo, K., and Guibas, L. J. (2017). Pointnet:
Deep learning on point sets for 3d classification and
segmentation. In Proceedings of CVPR.
Qi, C. R., Su, H., Nießner, M., Dai, A., Yan, M., and
Guibas, L. J. (2016). Volumetric and multi-view cnns
for object classification on 3d data. In Proceedings of
CVPR, pages 5648–5656.
Sfikas, K., Theoharis, T., and Pratikakis, I. (2017). Ex-
ploiting the PANORAMA Representation for Convo-
lutional Neural Network Classification and Retrieval.
In Eurographics Workshop on 3D Object Retrieval.
Shi, B., Bai, S., Zhou, Z., and Bai, X. (2015). Deeppano:
Deep panoramic representation for 3-d shape recogni-
tion. IEEE Signal Processing Letters, 22(12):2339–
2343.
Simonovsky, M. and Komodakis, N. (2017). Dynamic
edge-conditioned filters in convolutional neural net-
works on graphs. In Proceedings of CVPR.
Sinha, A., Bai, J., and Ramani, K. (2016). Deep learning 3d
shape surfaces using geometry images. In Proceed-
ings of ECCV, pages 223–240. Springer.
Su, H., Maji, S., Kalogerakis, E., and Learned-Miller, E.
(2015). Multi-view convolutional neural networks for
3d shape recognition. In Proceedings of ICCV, pages
945–953.
Tangelder, J. W. and Veltkamp, R. C. (2004). A survey of
content based 3d shape retrieval methods. In Proceed-
ings of IEEE Int. Conference on Shape Modeling Ap-
plications, pages 145–156.
Theano Development Team (2016). Theano: A Python
framework for fast computation of mathematical ex-
pressions. arXiv e-prints, abs/1605.02688.
Wu, J., Zhang, C., Xue, T., Freeman, B., and Tenenbaum,
J. (2016). Learning a probabilistic latent space of ob-
ject shapes via 3d generative-adversarial modeling. In
Advances in Neural Information Processing Systems.
Wu, Z., Song, S., Khosla, A., Yu, F., Zhang, L., Tang, X.,
and Xiao, J. (2015). 3d shapenets: A deep representa-
tion for volumetric shapes. In Proceedings of CVPR,
pages 1912–1920.
Xu, X. and Todorovic, S. (2016). Beam search for learning
a deep convolutional neural network of 3d shapes. In
Proceedings of ICPR, pages 3506–3511. IEEE.
Zanuttigh, P. and Minto, L. (2017). Deep learning for 3d
shape classification from multiple depth maps. In Pro-
ceedings of ICIP.
Zhi, S., Liu, Y., Li, X., and Guo, Y. (2017). Lightnet: A
lightweight 3d convolutional neural network for real-
time 3d object recognition. In Eurographics Workshop
on 3D Object Retrieval.
VISAPP 2018 - International Conference on Computer Vision Theory and Applications
324