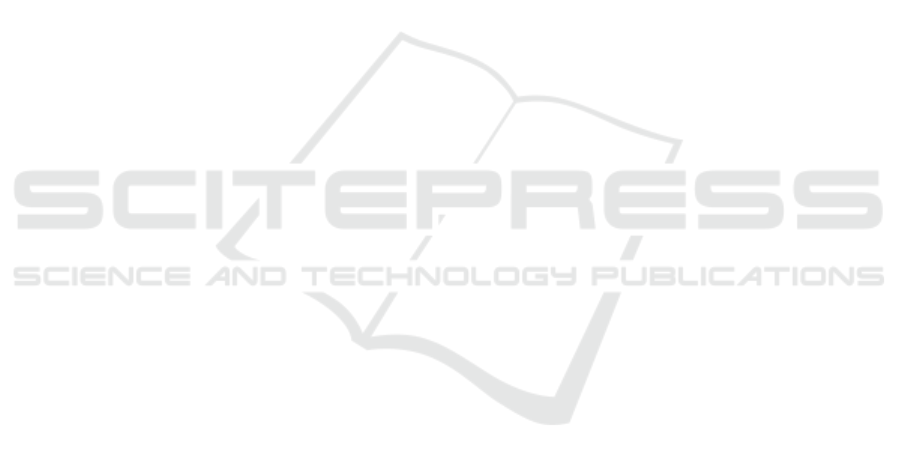
fore over segmentation to scenes that capture subtle
changes in the visual content. For keyframe selec-
tion, we found that motion descriptors are superior
over region features used in scene detection which
can be explained by their complementary informa-
tion. However, we also found that average perfor-
mance of keyframe selection methods is substantially
lower than with learning-based state-of-the-arts. We
also found that original frame rate provides good re-
sults. We introduced a GUI for fast (from 9 seconds to
less than two minutes) human-in-the-loop keyframe
selection which provides superior/on par performance
to state-of-the-art learning-based methods while re-
taining user control over personal preferences.
REFERENCES
Bian, J., Yang, Y., Zhang, H., and Chua, T.-S. (2015). Mul-
timedia summarization for social events in microblog
stream. IEEE Trans. on Multimedia, 17(2).
Chaudhry, R., Ravichandran, A., Hager, G., and Vidal, R.
(2009). Histograms of oriented optical flow and binet-
cauchy kernels on nonlinear dynamical systems for
the recognition of human actions. In The IEEE Con-
ference on Computer Vision and Pattern Recognition
(CVPR).
de Avila, S., Lopes, A., and et al. (2011a). VSUMM:
A mechanism designed to produce static video sum-
maries and a novel evaluation method. Pattern Recog-
nition Letters, 32(1):56–68.
de Avila, S. E. F., Lopes, A. P. B., da Luz Jr., A., and de Al-
buquerque Araajo, A. (2011b). VSUMM: A mecha-
nism designed to produce static video summaries and
a novel evaluation method. Pattern Recognition Let-
ters, 32(1).
Duan, X., Lin, L., and Chao, H. (2013). Discovering video
shot categories by unsupervised stochastic graph par-
tition. IEEE Trans. on Multimedia, 15(1).
Ejaz, N., Mehmood, I., and Baik, S. W. (2013). Efficient
visual attention based framework for extracting key
frames from videos. Image Commun., 28(1):34–44.
Fabro, M. D. and B
¨
osz
¨
ormenyi, L. (2013). State-of-the-
art and future challenges in video scene detection: a
survey. Multimedia Systems, 19:427–454.
Farneb
¨
ack, G. (2003). Two-frame motion estimation based
on polynomial expansion. In Proceedings of the 13th
Scandinavian Conference on Image Analysis (SCIA).
Gatica-Perez, D., Loui, A., and Sun, M.-T. (2003). Finding
structure in home videos by probabilistic hierarchical
clustering. IEEE Trans. on Circuits and Systems for
Video Technology, 13(6).
Gong, B., Chao, W.-L., Grauman, K., and Sha, F. (2014).
Diverse sequential subset selection for supervised
video summarization. In Conference on Neural In-
formation Processing Systems (NIPS).
Gu, Z., Mei, T., Hua, X.-S., Wu, X., and Li, S. (2007). Ems:
Energy minimization based video scene segmentation.
In ICME.
Guan, G., Wang, Z., Liu, S., Deng, J. D., and Feng, D.
(2013). Keypoint-based keyframe selection. IEEE
Trans. on Circuits and Systems for Video Technology,
24(4).
Gygli, M., Grabner, H., Riemenschneider, H., and
Van Gool, L. (2014). Creating summaries from user
videos. In European Conference on Computer Vision
(ECCV).
Gygli, M., Grabner, H., and Van Gool, L. (2015). Video
summarization by learning submodular mixtures of
objectives. In The IEEE Conference on Computer Vi-
sion and Pattern Recognition (CVPR).
Gygli, M., Song, Y., and Cao, L. (2016). Video2gif: Au-
tomatic generation of animated gifs from video. In
The IEEE Conference on Computer Vision and Pat-
tern Recognition (CVPR).
Han, B., Hamm, J., and Sim, J. (2010). Personalized video
summarization with human in the loop. In IEEE Work-
shop on Applications of Computer Vision(WACV).
Han, B. and Wu, W. (2011). Video scene segmentation us-
ing a novel boundary evaluation criterion and dynamic
programming. In ICME.
Herranz, L., Calic, J., Martinez, J., and Mrak, M. (2012).
Scalable comic-like video summaries and layout dis-
turbance. IEEE Trans. on Multimedia, 14(4).
Hong, R., Yuan, X.-T., Xu, M., and Wang, M. (2010).
Movie2comics: A feast of multimedia artwork. In
ACM Multimedia (ACMMM).
Jiang, Y.-G., Ye, G., Chang, S.-F., Ellis, D., and Loui, A.
(2011). Consumer video understanding: A benchmark
database and an evaluation of human and machine per-
formance. In ACM International Conference on Mul-
timedia Retrieval (ICMR).
Kyperountas, M., Kotropoulos, C., and Pitas, I. (2007).
Enhanced eigen-audioframes for audiovisual scene
change detection. IEEE Trans. on Multimedia, 9(4).
Lankinen, J. and K
¨
am
¨
ar
¨
ainen, J.-K. (2013). Video shot
boundary detection using visual bag-of-words. In Int.
Conf. on Computer Vision Theory and Applications
(VISAPP).
Lee, Y. and Grauman, K. (2015). Multimedia summariza-
tion for social events in microblog stream. Int J Com-
put Vis, 114:38–55.
Liu, W., Mei, T., Zhang, Y., Che, C., and Luo, J. (2015).
Multi-task deep visual-semantic embedding for video
thumbnail selection. In The IEEE Conference on
Computer Vision and Pattern Recognition (CVPR).
Lowe, D. (2004). Distinctive image features from scale-
invariant keypoints. Int J Comput Vis, 60(2):91–110.
Lu, S., Wang, Z., Mei, T., Guan, G., and Feng, D. (2014).
A bag-of-importance model with locality-constrained
coding based feature learning for video summariza-
tion. IEEE Trans. on Multimedia, 16(6).
Luo, J., Papin, C., and Costello, K. (2009). Towards ex-
tracting semantically meaningful key frames from per-
sonal video clips: from humans to computers. IEEE
Trans. on Circuits and Systems for Video Technology,
19(2):289–301.
Keyframe-based Video Summarization with Human in the Loop
295