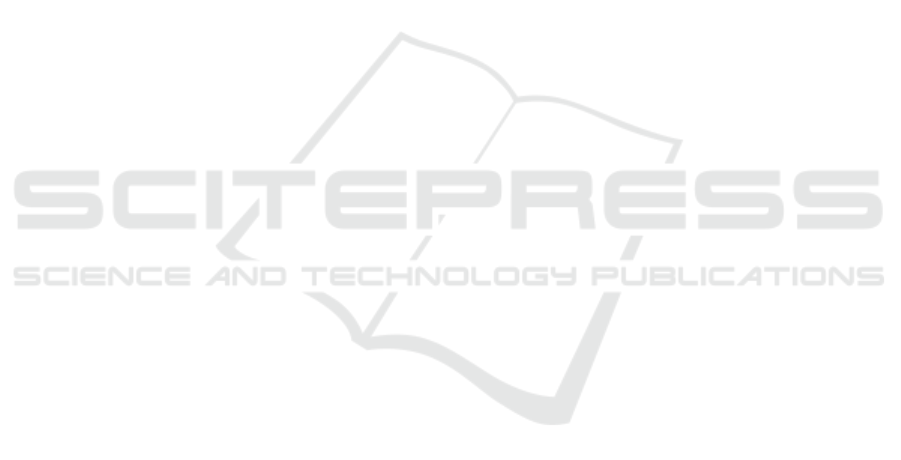
5 CONCLUSIONS
We presented a subtle motion analysis and spot-
ting method based on the Riesz pyramid. Our
method adapted the quaternionic representation of the
Riesz monogenic signal by proposing a new filtering
scheme. We were also able to mask regions of inter-
est where subtle motion might take place in order to
reduce the effect of noise using the image amplitude.
Furthermore, we illustrated the power of our subtle
motion analysis method by briefly presenting a couple
of potential real-life applications. After testing our
method using our own database under different levels
of Gaussian additive noise and salt and peper noise,
we can conclude that our method surpasses other state
of the art methods.
Due to the unavailability of a public labeled subtle
motion database we had to test our experiments in a
rather limited dataset. Further tests will require us to
create or find a more complete database in order to
obtain more statistically significant results.
The quaternionic representation of phase and ori-
entation from the Riesz monogenic signal is a power-
ful tool that could potentially be exploited in the fu-
ture for more focused applications like modal analy-
sis, biomedical signals processing, and facial micro-
expression spotting and recognition.
REFERENCES
Balakrishnan, G., Durand, F., and Guttag, J. (2013). Detect-
ing Pulse from Head Motions in Video. In 2013 IEEE
Conference on Computer Vision and Pattern Recogni-
tion, pages 3430–3437.
Barron, J. L., Fleet, D. J., and Beauchemin, S. S. (1994).
Performance of optical flow techniques. International
Journal of Computer Vision, 12(1):43–77.
Bharadwaj, S., Dhamecha, T. I., Vatsa, M., and Singh, R.
(2013). Computationally Efficient Face Spoofing De-
tection with Motion Magnification. In 2013 IEEE
Conference on Computer Vision and Pattern Recog-
nition Workshops, pages 105–110.
Chen, J. G., Wadhwa, N., Cha, Y.-J., Durand, F., Freeman,
W. T., and Buyukozturk, O. (2015). Modal identifica-
tion of simple structures with high-speed video using
motion magnification. Journal of Sound and Vibra-
tion, 345:58–71.
Dalal, N. and Triggs, B. (2005). Histograms of oriented gra-
dients for human detection. In 2005 IEEE Computer
Society Conference on Computer Vision and Pattern
Recognition (CVPR’05), volume 1, pages 886–893
vol. 1.
Irani, R., Nasrollahi, K., and Moeslund, T. B. (2014). Im-
proved Pulse Detection from Head Motions Using
DCT. Institute for Systems and Technologies of In-
formation, Control and Communication.
Kamate, S. and Yilmazer, N. (2015). Application of Object
Detection and Tracking Techniques for Unmanned
Aerial Vehicles. Procedia Computer Science, 61:436–
441.
Lee, J. and Shin, S. Y. (2002). General construction of time-
domain filters for orientation data. IEEE Transactions
on Visualization and Computer Graphics, 8(2):119–
128.
Li, X., Hong, X., Moilanen, A., Huang, X., Pfister, T.,
Zhao, G., and Pietikainen, M. (2017). Towards Read-
ing Hidden Emotions: A Comparative Study of Spon-
taneous Micro-expression Spotting and Recognition
Methods. IEEE Transactions on Affective Computing,
PP(99):1–1.
Liu, C., Torralba, A., Freeman, W. T., Durand, F., and Adel-
son, E. H. (2005). Motion Magnification. In ACM
SIGGRAPH 2005 Papers, SIGGRAPH ’05, pages
519–526, New York, NY, USA. ACM.
McLeod, A. J., Baxter, J. S. H., de Ribaupierre, S., and
Peters, T. M. (2014). Motion magnification for en-
doscopic surgery. volume 9036, pages 90360C–
90360C–8.
Ojala, T., Pietikainen, M., and Maenpaa, T. (2002). Mul-
tiresolution gray-scale and rotation invariant texture
classification with local binary patterns. IEEE Trans-
actions on Pattern Analysis and Machine Intelligence,
24(7):971–987.
Park, S. and Kim, D. (2009). Subtle facial expression recog-
nition using motion magnification. Pattern Recogni-
tion Letters, 30(7):708–716.
Raghavendra, R., Avinash, M., Marcel, S., and Busch, C.
(2015). Finger vein liveness detection using motion
magnification. In 2015 IEEE 7th International Con-
ference on Biometrics Theory, Applications and Sys-
tems (BTAS), pages 1–7.
Rassem, T. H. and Khoo, B. E. (2014). Completed local
ternary pattern for rotation invariant texture classifica-
tion. The Scientific World Journal, 2014.
Unser, M., Sage, D., and Ville, D. V. D. (2009). Multires-
olution Monogenic Signal Analysis Using the Riesz-
Laplace Wavelet Transform. IEEE Transactions on
Image Processing, 18(11):2402–2418.
Wadhwa, N., Rubinstein, M., Durand, F., and Freeman, W.
(2014a). Riesz pyramids for fast phase-based video
magnification. In 2014 IEEE International Confer-
ence on Computational Photography (ICCP), pages
1–10.
Wadhwa, N., Rubinstein, M., Durand, F., and Freeman,
W. T. (2013). Phase-based Video Motion Processing.
ACM Trans. Graph., 32(4):80:1–80:10.
Wadhwa, N., Rubinstein, M., Durand, F., and Freeman,
W. T. (2014b). Quaternionic representation of the
riesz pyramid for video magnification. Technical re-
port.
Wu, H.-Y., Rubinstein, M., Shih, E., Guttag, J., Durand, F.,
and Freeman, W. (2012). Eulerian Video Magnifica-
tion for Revealing Subtle Changes in the World. ACM
Trans. Graph., 31(4):65:1–65:8.
VISAPP 2018 - International Conference on Computer Vision Theory and Applications
454