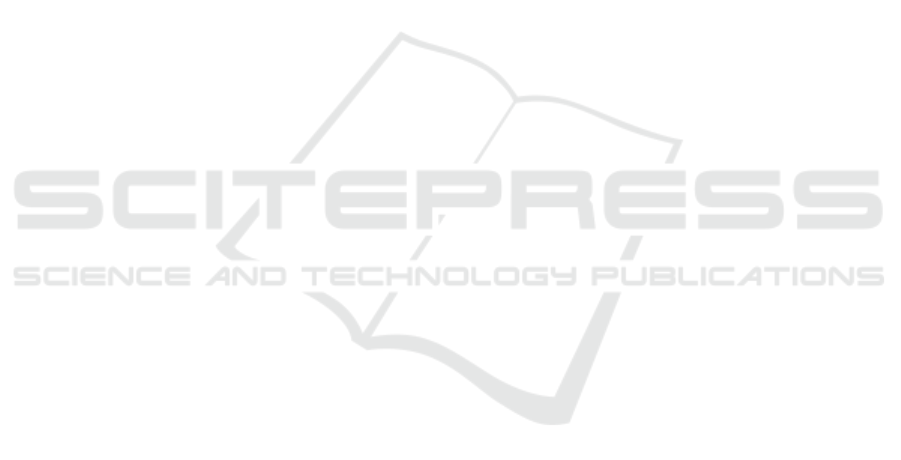
Charles, J., Pfister, T., Everingham, M., and Zisserman, A.
(2013). Automatic and efficient human pose estima-
tion for sign language videos. International Journal
of Computer Vision (IJCV).
Charles, J., Pfister, T., Magee, D., Hogg, D., and Zisser-
man, A. (2016). Personalizing video pose estimation.
In IEEE Conference on Computer Vision and Pattern
Recognition (CVPR).
Dantone, M., Gall, J., Leistner, C., and van Gool, L. (2013).
Human pose estimation using body parts dependent
joint regressors. In IEEE Conference on Computer
Vision and Pattern Recognition (CVPR), pages 3041–
3048, Portland, OR, USA.
Felzenszwalb, P., McAllester, D., and Ramanan, D. (2008).
A discriminatively trained, multiscale, deformable
part model. In IEEE Conference on Computer Vision
and Pattern Recognition (CVPR), pages 1–8.
Glorot, X. and Bengio, Y. (2010). Understanding the dif-
ficulty of training deep feedforward neural networks.
In International Conference on Artificial Intelligence
and Statistics (AISTATS’10).
Insafutdinov, E., Pishchulin, L., Andres, B., Andriluka, M.,
and Schiele, B. (2016). Deepercut: A deeper, stronger,
and faster multi-person pose estimation model. arXiv
preprint arXiv:1605.03170.
Jain, A., Tompson, J., Andriluka, M., Taylor, G. W., and
Bregler, C. (2013). Learning human pose estimation
features with convolutional networks. arXiv preprint
arXiv:1312.7302.
Jain, A., Tompson, J., LeCun, Y., and Bregler, C. (2014).
Modeep: A deep learning framework using motion
features for human pose estimation. In Asian Con-
ference on Computer Vision (ACCV), pages 302–315.
Jia, Y., Shelhamer, E., Donahue, J., Karayev, S., Long, J.,
Girshick, R., Guadarrama, S., and Darrell, T. (2014).
Caffe: Convolutional architecture for fast feature em-
bedding. arXiv preprint arXiv:1408.5093.
Johnson, S. and Everingham, M. (2010). Clustered pose and
nonlinear appearance models for human pose estima-
tion. In British Machine Vision Conference (BMVC).
doi:10.5244/C.24.12.
Krizhevsky, A., Sutskever, I., and Hinton, G. E. (2012).
Imagenet classification with deep convolutional neu-
ral networks. In Advances in Neural Information Pro-
cessing Systems (NIPS), pages 1097–1105.
Lifshitz, I., Fetaya, E., and Ullman, S. (2016). Human pose
estimation using deep consensus voting. arXiv pre-
print arXiv:1603.08212.
Newell, A., Yang, K., and Deng, J. (2016). Stacked hour-
glass networks for human pose estimation. arXiv pre-
print arXiv:1603.06937.
Pfister, T., Charles, J., and Zisserman, A. (2015). Flowing
convnets for human pose estimation in videos. In In-
ternational Conference on Computer Vision (ICCV).
Pfister, T., Simonyan, K., Charles, J., and Zisserman, A.
(2014). Deep convolutional neural networks for effi-
cient pose estimation in gesture videos. In Asian Con-
ference on Computer Vision (ACCV).
Pishchulin, L., Insafutdinov, E., Tang, S., Andres, B.,
Andriluka, M., Gehler, P., and Schiele, B. (2015).
Deepcut: Joint subset partition and labeling for
multi person pose estimation. arXiv preprint
arXiv:1511.06645.
Pishchulin, L., Jain, A., Andriluka, M., Thorm
¨
ahlen, T., and
Schiele, B. (2012). Articulated people detection and
pose estimation: Reshaping the future. In IEEE Con-
ference on Computer Vision and Pattern Recognition
(CVPR), pages 3178–3185.
Ren, S., He, K., Girshick, R., and Sun, J. (2015). Faster
r-cnn: Towards real-time object detection with region
proposal networks. arXiv preprint arXiv:1506.01497.
Sapp, B. and Taskar, B. (2013). Modec: Multimodal de-
composable models for human pose estimation. In
IEEE Conference on Computer Vision and Pattern Re-
cognition (CVPR), pages 3674–3681.
Shotton, J., Sharp, T., Kipman, A., Fitzgibbon, A., Fi-
nocchio, M., Blake, A., Cook, M., and Moore, R.
(2013). Real-time human pose recognition in parts
from single depth images. Communications of the
ACM, 56(1):116–124.
Tompson, J., Goroshin, R., Jain, A., LeCun, Y., and Bregler,
C. (2015). Efficient object localization using convolu-
tional networks. In IEEE Conference on Computer
Vision and Pattern Recognition (CVPR), pages 648–
656.
Toshev, A. and Szegedy, C. (2014). Deeppose: Human pose
estimation via deep neural networks. In IEEE Con-
ference on Computer Vision and Pattern Recognition
(CVPR).
Wei, S.-E., Ramakrishna, V., Kanade, T., and Sheikh, Y.
(2016). Convolutional pose machines. arXiv preprint
arXiv:1602.00134.
Yang, Y. and Ramanan, D. (2011). Articulated pose esti-
mation with flexible mixtures-of-parts. In IEEE Con-
ference on Computer Vision and Pattern Recognition
(CVPR), pages 1385–1392.
VISAPP 2018 - International Conference on Computer Vision Theory and Applications
342