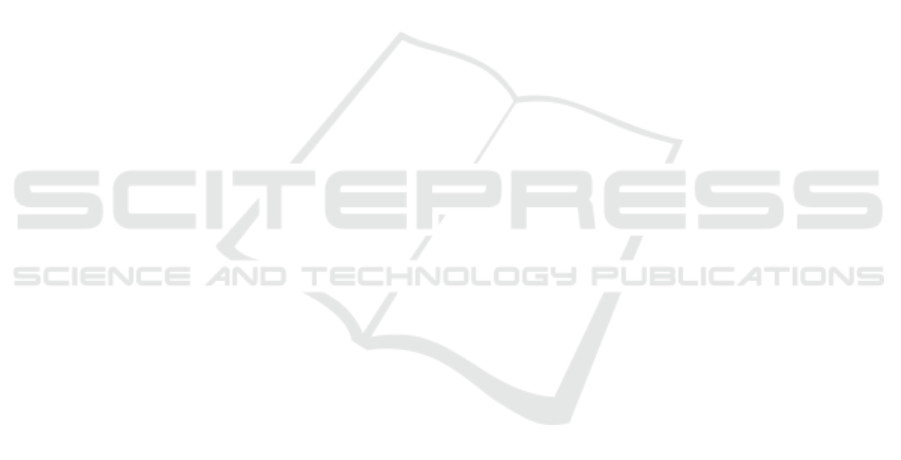
sequences in C.elegans. The novelty of this work
resides in the fact of the all helitron’s classification
using the energy of matrix contains the coefficient of
wavelet (time-frequencies presentation). These
energy-vector can characterize each helitrons by
specific frequencies that have energy around the
specific frequency.
REFERENCES
Amin, H. U., Malik, A. S., Ahmad, R. F., Badruddin, N.,
Kamel, N., Hussain, M., & Chooi, W. T. 2015. Feature
extraction and classification for EEG signals using
wavelet transform and machine learning
techniques. Australasian physical & engineering
sciences in medicine, 38(1), 139-149.
Chang, C. C., & Lin, C. J. 2011. LIBSVM: a library for
support vector machines. ACM transactions on
intelligent systems and technology (TIST), 2(3), 27.
Du, C., Fefelova, N., Caronna, J., He, L., & Dooner, H. K.
2009. The polychromatic Helitron landscape of the
maize genome. Proceedings of the National Academy
of Sciences, 106(47), 19916-19921.
Du, C., Caronna, J., He, L., & Dooner, H. K. 2008.
Computational prediction and molecular confirmation
of Helitron transposons in the maize genome. Bmc
Genomics, 9(1), 51.
Edgar, R. C. 2004. MUSCLE: multiple sequence alignment
with high accuracy and high throughput. Nucleic acids
research, 32(5), 1792-1797.
Norinder, U. 2003. Support vector machine models in drug
design: applications to drug transport processes and
QSAR using simplex optimisations and variable
selection. Neurocomputing, 55(1), 337-346.
Mateos, A., Dopazo, J., Jansen, R., Tu, Y., Gerstein, M., &
Stolovitzky, G. 2002. Systematic learning of gene
functional classes from DNA array expression data by
using multilayer perceptrons. In Genome
Research, 12(11), 1703-1715.
Öz, E., & Kaya, H. 2013. Support vector machines for
quality control of DNA sequencing. Journal of
Inequalities and Applications, 2013(1), 85.
Furey, T. S., Cristianini, N., Duffy, N., Bednarski, D. W.,
Schummer, M., & Haussler, D. 2000. Support vector
machine classification and validation of cancer tissue
samples using microarray expression data.
Bioinformatics, 16(10), 906-914.
Hsu, C. W., & Lin, C. J. 2002. A comparison of methods
for multiclass support vector machines. IEEE
transactions on Neural Networks, 13(2), 415-425.
Hsu,C.W., Chang, C.C. and Lin, C. J. 2009. A practical
guide to support vector classification,” Department of
Computer Science and Information Engineering
National Taiwan University, Taipei, Taiwan,
Available: www.csie.ntu.edu.tw/ cjlin/
Shawe-Taylor, J., Bartlett, P. L., Williamson, R. C., &
Anthony, M. 1998. Structural risk minimization over
data-dependent hierarchies. IEEE transactions on
Information Theory, 44(5), 1926-1940.
Vapnik, V.N., Vapnik,V. 1998. “Statistical learning
theory,” New York : Wiley,.
Vapnik, V., 1995. The nature of statistical learning theory,”
Springer Verlag New York, Inc. New York,
Gutschoven, B., & Verlinde, P. 2000. Multi-modal identity
verification using support vector machines (SVM).
In Information Fusion, 2000. FUSION 2000.
Proceedings of the Third International Conference
on (Vol. 2, pp. THB3-3). IEEE.
Poulter, R. T. M., & Goodwin, T. J. D. 2005. DIRS-1 and
the other tyrosine recombinase retrotransposons.
Cytogenetic and genome research, 110(1-4), 575-588.
Hood, M. E. 2005. Repetitive DNA in the automictic
fungus Microbotryum violaceum. Genetica, 124(1), 1-
10.
Kapitonov, V. V., & Jurka, J. 2001. Rolling-circle
transposons in eukaryotes. Proceedings of the National
Academy of Sciences, 98(15), 8714-8719.
Lai, J., Li, Y., Messing, J., & Dooner, H. K. 2005. Gene
movement by Helitron transposons contributes to the
haplotype variability of maize. Proceedings of the
National Academy of Sciences of the United States of
America, 102(25), 9068-9073.
Schnable, P. S., Ware, D., Fulton, R. S., Stein, J. C., Wei,
F., Pasternak, S., ... & Minx, P. 2009. The B73 maize
genome: complexity, diversity, and dynamics. Science,
326(5956), 1112-1115.
Tempel, S. 2007. Dynamique des hélitrons dans le genome
d'Arabidopsis thaliana: développement de nouvelles
stratégies d'analyse des éléments transposables
(Doctoral dissertation, Université Rennes 1).
Sweredoski, M., DeRose-Wilson, L., & Gaut, B. S. 2008.
A comparative computational analysis of
nonautonomous helitron elements between maize and
rice. BMC genomics, 9(1), 467.
Xiong, W., He, L., Lai, J., Dooner, H. K., & Du, C. 2014.
HelitronScanner uncovers a large overlooked cache of
Helitron transposons in many plant
genomes. Proceedings of the National Academy of
Sciences, 111(28), 10263-10268.
Yang, L., & Bennetzen, J. L. 2009. Structure-based
discovery and description of plant and animal
Helitrons. Proceedings of the National Academy of
Sciences, 106(31), 12832-12837.
Wicker, T., Sabot, F., Hua-Van, A., Bennetzen, J. L., Capy,
P., Chalhoub, B., ... & Paux, E. 2007. A unified
classification system for eukaryotic transposable
elements. Nature Reviews Genetics, 8(12), 973-982.
Fiser, A., Tusnady, G. E., & Simon, I. 1994. Chaos game
representation of protein structures. Journal of
molecular graphics, 12(4), 302-304.
Merry, R. J. E., & Steinbuch, M. 2005. Wavelet theory and
applications. literature study, Eindhoven university of
technology, Department of mechanical engineering,
Control systems technology group.
Morgante, M., Brunner, S., Pea, G., Fengler, K., Zuccolo,
A., & Rafalski, A. 2005. Gene duplication and exon
shuffling by helitron-like transposons generate
Classification of Helitron’s Types in the C.elegans Genome based on Features Extracted from Wavelet Transform and SVM Methods
133