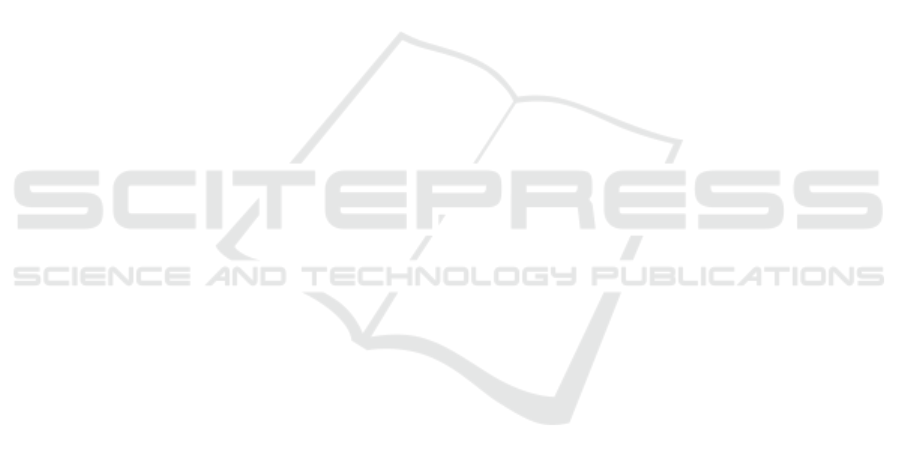
trieval on mobile devices where large flash drives
are becoming common but computational power and
main memory is still limited.
ACKNOWLEDGEMENTS
This work was supported by the German Ministry
of Education and Research (BMBF) (grant number
13N14028).
REFERENCES
Arandjelovi
´
c, R. and Zisserman, A. (2012). Three things
everyone should know to improve object retrieval. In
Computer Vision and Pattern Recognition (CVPR),
2012 IEEE Conference on, pages 2911–2918. IEEE.
Babenko, A. and Lempitsky, V. (2015). Aggregating local
deep features for image retrieval. In Proceedings of
the IEEE International Conference on Computer Vi-
sion, pages 1269–1277.
Goossaert, E. (2014). Coding for ssds.
http://codecapsule.com/2014/02/12/coding-for-
ssds-part-1-introduction-and-table-of-contents/.
Jegou, H., Douze, M., and Schmid, C. (2008). Hamming
embedding and weak geometric consistency for large
scale image search. In European conference on com-
puter vision, pages 304–317. Springer.
J
´
egou, H., Douze, M., Schmid, C., and P
´
erez, P. (2010). Ag-
gregating local descriptors into a compact image rep-
resentation. In Computer Vision and Pattern Recogni-
tion (CVPR), 2010 IEEE Conference on, pages 3304–
3311. IEEE.
Kalantidis, Y., Mellina, C., and Osindero, S. (2015). Cross-
dimensional weighting for aggregated deep convolu-
tional features. arXiv preprint arXiv:1512.04065.
Khan, F. S., Anwer, R. M., Van De Weijer, J., Bagdanov,
A. D., Vanrell, M., and Lopez, A. M. (2012). Color
attributes for object detection. In Computer Vision and
Pattern Recognition (CVPR), 2012 IEEE Conference
on, pages 3306–3313. IEEE.
Lowe, D. G. (2004). Distinctive image features from scale-
invariant keypoints. International journal of computer
vision, 60(2):91–110.
Manger, D. and Willersinn, D. (2017). Extending the bag-
of-words representation with neighboring local fea-
tures and deep convolutional features. In 2017 Irish
Machine Vision and Image Processing Conference
(IMVIP).
Mark J. Huiskes, B. T. and Lew, M. S. (2010). New
trends and ideas in visual concept detection: The mir
flickr retrieval evaluation initiative. In MIR ’10: Pro-
ceedings of the 2010 ACM International Conference
on Multimedia Information Retrieval, pages 527–536,
New York, NY, USA. ACM.
Perronnin, F. and Dance, C. (2007). Fisher kernels on visual
vocabularies for image categorization. In 2007 IEEE
Conference on Computer Vision and Pattern Recogni-
tion, pages 1–8. IEEE.
Philbin, J., Chum, O., Isard, M., Sivic, J., and Zisserman, A.
(2007). Object retrieval with large vocabularies and
fast spatial matching. In 2007 IEEE Conference on
Computer Vision and Pattern Recognition, pages 1–8.
IEEE.
Philbin, J., Chum, O., Isard, M., Sivic, J., and Zisserman,
A. (2008). Lost in quantization: Improving partic-
ular object retrieval in large scale image databases.
In Computer Vision and Pattern Recognition, 2008.
CVPR 2008. IEEE Conference on, pages 1–8. IEEE.
Razavian, A. S., Sullivan, J., Maki, A., and Carlsson,
S. (2014). A baseline for visual instance retrieval
with deep convolutional networks. arXiv preprint
arXiv:1412.6574.
Simonyan, K. and Zisserman, A. (2014). Very deep con-
volutional networks for large-scale image recognition.
arXiv preprint arXiv:1409.1556.
Sivic, J. and Zisserman, A. (2003). Video google: A text
retrieval approach to object matching in videos. In
Computer Vision, 2003. Proceedings. Ninth IEEE In-
ternational Conference on, pages 1470–1477. IEEE.
Tolias, G., Sicre, R., and J
´
egou, H. (2015). Particular object
retrieval with integral max-pooling of cnn activations.
arXiv preprint arXiv:1511.05879.
Zhang, S., Tian, Q., Huang, Q., Gao, W., and Rui, Y. (2013).
Multi-order visual phrase for scalable image search.
In Proceedings of the Fifth International Conference
on Internet Multimedia Computing and Service, pages
145–149. ACM.
Zheng, L., Wang, S., Liu, Z., and Tian, Q. (2014). Packing
and padding: Coupled multi-index for accurate im-
age retrieval. In Proceedings of the IEEE Conference
on Computer Vision and Pattern Recognition, pages
1939–1946.
VISAPP 2018 - International Conference on Computer Vision Theory and Applications
372