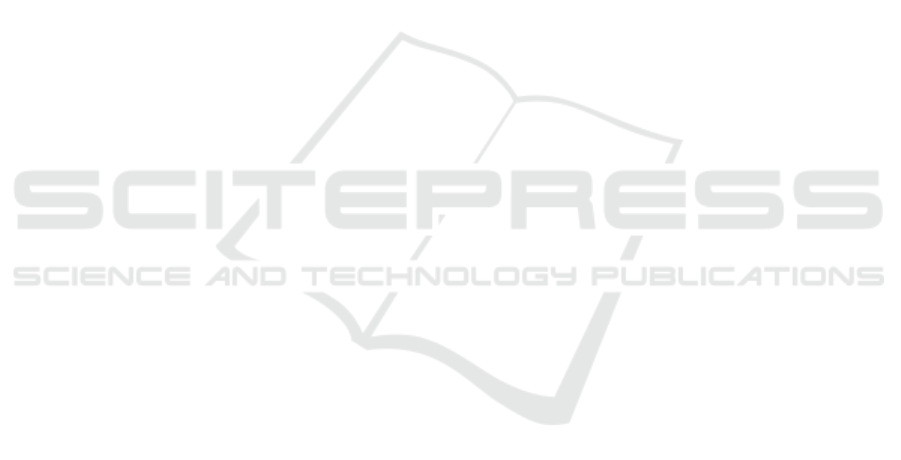
vity data reveal worldwide activity inequality. Nature,
547(7663):336–339.
Alves, A. J., Viana, J. L., Cavalcante, S. L., Oliveira, N. L.,
Duarte, J. A., Mota, J., Oliveira, J., and Ribeiro, F.
(2016). Physical activity in primary and secondary
prevention of cardiovascular disease: overview upda-
ted. World Journal of Cardiology, 8(10):575–583.
Biswas, A., Oh, P. I., Faulkner, G. E., Bajaj, R. R., Silver,
M. A., Mitchell, M. S., and Alter, D. A. (2015). Se-
dentary time and its association with risk for disease
incidence, mortality, and hospitalization in adults: a
systematic review and meta-analysis. Annals of Inter-
nal Medicine, 162(2):123–132.
Charitos, E. I., Stierle, U., Ziegler, P. D., Baldewig, M., Ro-
binson, D. R., Sievers, H.-H., and Hanke, T. (2012).
A comprehensive evaluation of rhythm monitoring
strategies for the detection of atrial fibrillation recur-
rence: insights from 647 continuously monitored pa-
tients and implications for monitoring after therapeu-
tic interventions. Circulation, 126(7):806–814.
Charitos, E. I., Ziegler, P. D., Stierle, U., Sievers, H.-H.,
Paarmann, H., and Hanke, T. (2013). Atrial fibril-
lation density: A novel measure of atrial fibrillation
temporal aggregation for the characterization of atrial
fibrillation recurrence pattern. Applied Cardiopulmo-
nary Pathophysiology, 17(1):3–10.
de Souto Barreto, P., Cesari, M., Andrieu, S., Vellas, B.,
and Rolland, Y. (2017). Physical activity and incident
chronic diseases: a longitudinal observational study in
16 European countries. American Journal of Preven-
tive Medicine, 52(3):373–378.
Gerber, Y., Myers, V., Goldbourt, U., Benyamini, Y., Schei-
nowitz, M., and Drory, Y. (2011). Long-term trajec-
tory of leisure time physical activity and survival after
first myocardial infarction: a population-based cohort
study. European Journal of Epidemiology, 26(2):109–
116.
Leininger, L. J., Cook, B. J., and Adams, K. J. (2016). Va-
lidation and accuracy of Fitbit Charge: A pilot study
in a university worksite walking program. Journal of
Fitness Research, 5(2):3–9.
Leth, S., Hansen, J., Nielsen, O. W., and Dinesen, B. (2017).
Evaluation of commercial self-monitoring devices for
clinical purposes: results from the future patient trial,
phase I. Sensors, 17(1):211.
Physical Activity Guidelines Advisory Committee Report
(2008). Washington, DC: US Department of Health
and Human Services, 2008:A1–H14.
Piwek, L., Ellis, D. A., Andrews, S., and Joinson, A. (2016).
The rise of consumer health wearables: promises and
barriers. PLoS Medicine, 13(2):e1001953.
Sattelmair, J., Pertman, J., Ding, E. L., Kohl, H. W., Has-
kell, W., and Lee, I.-M. (2011). Dose response bet-
ween physical activity and risk of coronary heart dise-
ase. Circulation, 124(7):784–791.
Warburton, D. E., Charlesworth, S., Ivey, A., Nettlefold,
L., and Bredin, S. S. (2010). A systematic review of
the evidence for Canada’s physical activity guidelines
for adults. The International Journal of Behavioral
Nutrition and Physical Activity, 7:39.
Wilmot, E. G., Edwardson, C. L., Achana, F. A., Davies,
M. J., Gorely, T., Gray, L. J., Khunti, K., Yates, T.,
and Biddle, S. J. (2012). Sedentary time in adults and
the association with diabetes, cardiovascular disease
and death: systematic review and meta-analysis. Dia-
betologia, 55(11):2895–2905.
BIOSIGNALS 2018 - 11th International Conference on Bio-inspired Systems and Signal Processing
194