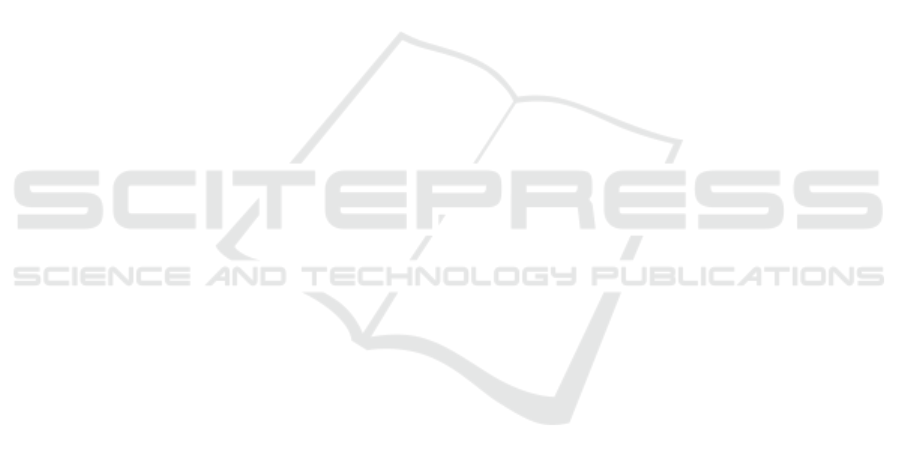
Bulashevska, A. and Eils, R. (2008). Using Bayesian multi-
nomial classifier to predict whether a given protein se-
quence is intrinsically disordered. Journal of theoret-
ical biology, 254(4):799–803.
Cortes, C. and Vapnik, V. (1995). Support-vector networks.
Machine Learning, 20(3):273–297.
DeForte, S. and Uversky, V. N. (2016). Order, Disorder, and
Everything in Between. Molecules, 21(8):1090.
Deng, X., Eickholt, J., and Cheng, J. (2012). A compre-
hensive overview of computational protein disorder
prediction methods. Molecular BioSystems, 8(1):114–
121.
Dosztnyi, Z., Csizmok, V., Tompa, P., and Simon, I. (2005).
IUPred: web server for the prediction of intrinsically
unstructured regions of proteins based on estimated
energy content. Bioinformatics, 21(16):3433–3434.
Faraggi, E., Zhang, T., Yang, Y., Kurgan, L., and Zhou, Y.
(2012). SPINE X: improving protein secondary struc-
ture prediction by multistep learning coupled with
prediction of solvent accessible surface area and back-
bone torsion angles. Journal of computational chem-
istry, 33(3):259–267.
He, B., Wang, K., Liu, Y., Xue, B., Uversky, V. N., and
Dunker, A. K. (2009). Predicting intrinsic disorder in
proteins: an overview. Cell research, 19(8):929–949.
Hollingsworth, S. A. and Karplus, P. A. (2010). A fresh look
at the Ramachandran plot and the occurrence of stan-
dard structures in proteins. Biomolecular concepts,
1(3-4):271–283.
Hollingsworth, S. A., Lewis, M. C., Berkholz, D. S., Wong,
W.-K., and Karplus, P. A. (2012). ($\phi$, $\psi$)
2 motifs: a purely conformation-based fine-grained
enumeration of protein parts at the two-residue level.
Journal of molecular biology, 416(1):78–93.
Jones, D. T. and Cozzetto, D. (2014). DISOPRED3: precise
disordered region predictions with annotated protein-
binding activity. Bioinformatics, page btu744.
Kalmankar, N. V., Ramakrishnan, C., and Balaram, P.
(2014). Sparsely populated residue conformations
in protein structures: Revisiting experimental Ra-
machandran maps. Proteins: Structure, Function, and
Bioinformatics, 82(7):1101–1112.
Kawashima, S. and Kanehisa, M. (2000). AAindex:
amino acid index database. Nucleic acids research,
28(1):374–374.
Kozlowski, L. P. and Bujnicki, J. M. (2012). MetaDisorder:
a meta-server for the prediction of intrinsic disorder in
proteins. BMC bioinformatics, 13(1):111.
Lafferty, J., McCallum, A., and Pereira, F. (2001). Con-
ditional random fields: Probabilistic models for seg-
menting and labeling sequence data. In Proceedings
of the eighteenth international conference on machine
learning, ICML, volume 1, pages 282–289.
Lieutaud, P., Canard, B., and Longhi, S. (2008). MeDor: a
metaserver for predicting protein disorder. BMC ge-
nomics, 9(Suppl 2):S25.
Mizianty, M. J., Stach, W., Chen, K., Kedarisetti, K. D.,
Disfani, F. M., and Kurgan, L. (2010). Improved
sequence-based prediction of disordered regions with
multilayer fusion of multiple information sources.
Bioinformatics, 26(18):i489–i496.
Morency, L.-P. (2015). HCRF library (including CRF and
LDCRF).
Oates, M. E., Romero, P., Ishida, T., Ghalwash, M.,
Mizianty, M. J., Xue, B., Dosztnyi, Z., Uversky, V. N.,
Obradovic, Z., Kurgan, L., and others (2013). D2p2:
database of disordered protein predictions. Nucleic
acids research, 41(D1):D508–D516.
Peng, Z., Yan, J., Fan, X., Mizianty, M. J., Xue, B., Wang,
K., Hu, G., Uversky, V. N., and Kurgan, L. (2015).
Exceptionally abundant exceptions: comprehensive
characterization of intrinsic disorder in all domains of
life. Cellular and Molecular Life Sciences, 72(1):137–
151.
Potenza, E., Di Domenico, T., Walsh, I., and Tosatto, S. C.
(2015). MobiDB 2.0: an improved database of intrin-
sically disordered and mobile proteins. Nucleic acids
research, 43(D1):D315–D320.
Schlessinger, A., Punta, M., and Rost, B. (2007). Natively
unstructured regions in proteins identified from con-
tact predictions. Bioinformatics, 23(18):2376–2384.
Sickmeier, M., Hamilton, J. A., LeGall, T., Vacic, V.,
Cortese, M. S., Tantos, A., Szabo, B., Tompa, P.,
Chen, J., Uversky, V. N., and others (2007). DisProt:
the database of disordered proteins. Nucleic acids re-
search, 35(suppl 1):D786–D793.
Sirota, F. L., Ooi, H.-S., Gattermayer, T., Schneider, G.,
Eisenhaber, F., and Maurer-Stroh, S. (2010). Parame-
terization of disorder predictors for large-scale appli-
cations requiring high specificity by using an extended
benchmark dataset. BMC genomics, 11(Suppl 1):S15.
Tibshirani, R. (1996). Regression shrinkage and selection
via the lasso. Journal of the Royal Statistical Society.
Series B (Methodological), pages 267–288.
Tsuruoka, Y., Tsujii, J., and Ananiadou, S. (2009). Stochas-
tic Gradient Descent Training for L1-regularized Log-
linear Models with Cumulative Penalty. In Pro-
ceedings of the Joint Conference of the 47th Annual
Meeting of the ACL and the 4th International Joint
Conference on Natural Language Processing of the
AFNLP: Volume 1 - Volume 1, ACL ’09, pages 477–
485, Stroudsburg, PA, USA. Association for Compu-
tational Linguistics.
Uribe, J. A., Arias-Londo
˜
no, J. D., and Perera-Lluna, A.
(2017). Protein Disorder Prediction using Informa-
tion Theory Measures on the Distribution of the Dihe-
dral Torsion Angles from Ramachandran Plots:. pages
43–51. SCITEPRESS - Science and Technology Pub-
lications.
Uversky, V. N., Oldfield, C. J., and Dunker, A. K. (2008).
Intrinsically disordered proteins in human diseases:
introducing the D2 concept. Annu. Rev. Biophys.,
37:215–246.
Varadi, M., Vranken, W., Guharoy, M., and Tompa, P.
(2015). Computational approaches for inferring the
functions of intrinsically disordered proteins. Fron-
tiers in molecular biosciences, 2.
Walsh, I., Martin, A. J., Di Domenico, T., and Tosatto, S. C.
Protein Disorder Prediction using Jumping Motifs from Torsion Angles Dynamics in Ramachandran Plots
47