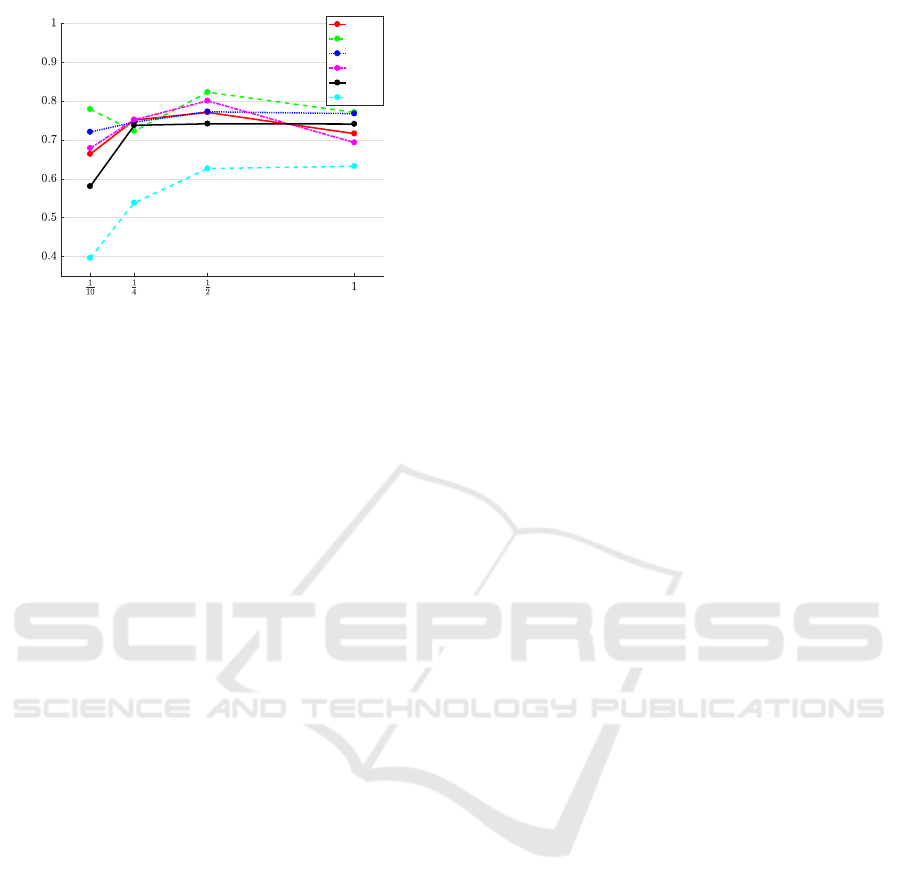
Percentages p of the action
Average Detection (%)
b = 2
b = 3
b = 4
b = 5
b = 7
b = 9
Figure 6: Average detection for the different subsequences
with length p of the template action for τ = 2.
5 CONCLUSIONS
In this paper, we proposed an online method to detect
suspicious actions with low latency. This method is
based on an adaptive sliding window which efficiently
rejects irrelevant data during streaming. We explored
the feature representation of a subsequence using the
spatial and temporal information of the video stream.
Furthermore, we evaluated the relationship between
the size of the template action and latency, where we
conclude that using half of the action as a template
action, the detection accuracy and the time needed to
detect the action achieve competitive and promising
results compared to using the full action as a tem-
plate. We also observed that tuning the parameters,
the method can be used for different setups of video
surveillance. Next, we intend to use real surveillance
videos coupled with a robust human pose detection
approach, e.g. (Pishchulin et al., 2016).
ACKNOWLEDGEMENTS
This work has been partially funded by the Natio-
nal Research Fund (FNR), Luxembourg, under the
CORE project C15/IS 10415355/3D-ACT/Bj
¨
orn Ot-
tersten. This work was also supported by the Euro-
pean Union‘s Horizon 2020 research and innovation
project STARR under grant agreement No.689947.
REFERENCES
Antunes, M., Baptista, R., Demisse, G., Aouada, D., and
Ottersten, B. (2016). Visual and human-interpretable
feedback for assisting physical activity. In European
Conference on Computer Vision (ECCV) Workshop on
Assistive Computer Vision and Robotics Amsterdam,.
Baptista, R., Antunes, M., Aouada, D., and Ottersten, B.
(2017a). Video-based feedback for assisting physical
activity. In International Joint Conference on Compu-
ter Vision, Imaging and Computer Graphics Theory
and Applications (VISAPP).
Baptista, R., Antunes, M., Shabayek, A. E. R., Aouada, D.,
and Ottersten, B. (2017b). Flexible feedback system
for posture monitoring and correction. In IEEE In-
ternational Conference on Image Information Proces-
sing (ICIIP).
Bilen, H., Fernando, B., Gavves, E., Vedaldi, A., and Gould,
S. (2016). Dynamic image networks for action recog-
nition. In The IEEE Conference on Computer Vision
and Pattern Recognition (CVPR).
Bilinski, P. and Bremond, F. (2016). Human violence re-
cognition and detection in surveillance videos. In Ad-
vanced Video and Signal Based Surveillance (AVSS),
2016 13th IEEE International Conference on, pages
30–36. IEEE.
Chu, W.-S., Zhou, F., and De la Torre, F. (2012). Unsu-
pervised temporal commonality discovery. In Euro-
pean Conference on Computer Vision, pages 373–387.
Springer Berlin Heidelberg.
Datta, A., Shah, M., and Da Vitoria Lobo, N. (2002).
Person-on-person violence detection in video data. In
Proceedings of the 16 th International Conference on
Pattern Recognition (ICPR’02) Volume 1 - Volume
1, ICPR ’02, pages 10433–, Washington, DC, USA.
IEEE Computer Society.
Du, Y., Wang, W., and Wang, L. (2015). Hierarchical recur-
rent neural network for skeleton based action recog-
nition. In The IEEE Conference on Computer Vision
and Pattern Recognition (CVPR).
Fernando, B., Gavves, E., Oramas, J., Ghodrati, A., and
Tuytelaars, T. (2016). Rank pooling for action recog-
nition. IEEE Transactions on Pattern Analysis and
Machine Intelligence.
Gaidon, A., Harchaoui, Z., and Schmid, C. (2011). Actom
Sequence Models for Efficient Action Detection. In
CVPR 2011 - IEEE Conference on Computer Vision
& Pattern Recognition, pages 3201–3208, Colorado
Springs, United States. IEEE.
Geest, R. D., Gavves, E., Ghodrati, A., Li, Z., Snoek, C.,
and Tuytelaars, T. (2016). Online action detection.
CoRR, abs/1604.06506.
Gkioxari, G. and Malik, J. (2015). Finding action tubes.
Han, F., Reily, B., Hoff, W., and Zhang, H. (2017). Space-
time representation of people based on 3d skeletal
data: A review. Computer Vision and Image Under-
standing, pages –.
Hoai, M. and De la Torre, F. (2012). Max-margin early
event detectors. In Proceedings of IEEE Conference
on Computer Vision and Pattern Recognition.
Hoai, M. and De la Torre, F. (2014). Max-margin early
event detectors. International Journal of Computer
Vision, 107(2):191–202.
Jain, M., van Gemert, J. C., J
´
egou, H., Bouthemy, P., and
Snoek, C. G. M. (2014). Action localization by tube-
Anticipating Suspicious Actions using a Small Dataset of Action Templates
385