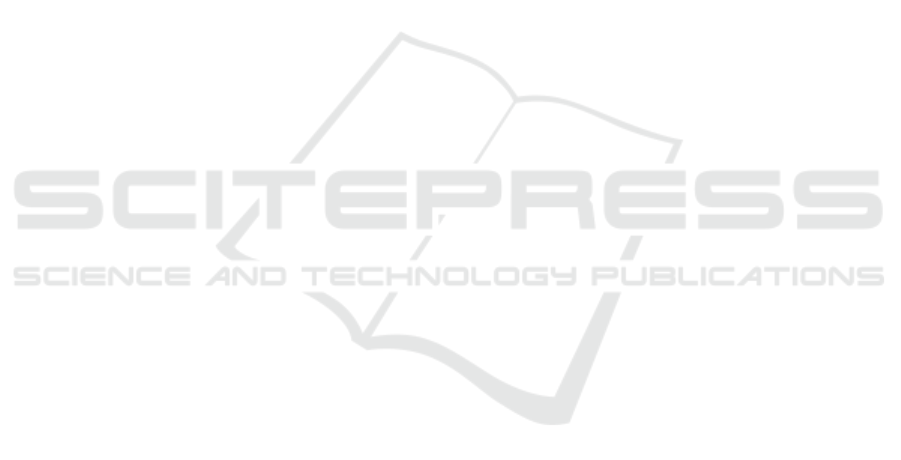
influence the determination of stress and relax states
in this case.
On the other hand, the use of the interpolation by
moving window gives us more flexibility. Compared
to the interpolation by splitting the interval, we can
experiment with the delta parameter and achieve a
better fit of the linear regression method to improve
the identification of stress dichotomy. We are fully
aware of this matter, but the confirmation of such
hypothesis can be achieved only with a bigger data
sample in the following research.
5.2 Further Research
The presented approach and the preliminary results
themselves are good starting points for further
research.
It is evident that the method itself has several
problems. For example, it describes the situation
related to acute stress within the time window that is
given by the sentiment interpolation window in which
the linear regression applied to the HR data was
performed. Since the stress state is supposed to be
present in a time window around its occurrence, we
can experiment with the length of the interpolation
window.
Moreover, when using the simple linear
regression, the regression fit was not calculated. This
needs to be considered in the next work. Besides
using the simple linear regression, we can also
process the HR data by using the method of simple
moving average (SMA).
Furthermore, the current study focuses on fairly
simple signal pre-processing steps rather than using
arguably more appropriate methods such as dynamic
Bayesian networks (DBN) (Dagum P., 1992; Dagum
P., 1995) and Hidden Markov Models (HMM)
(Wahlström J., 2017).
About the related work on the topic of fusing
social media and sensor data, we would also take into
account existing research in similar fields such as
(Farseev A., 2017) and (Choudhury M. De, 2017).
5.3 Further Data Collection
With a bigger and more rigorous experimental setup
(multiple subjects of both genders and various age
categories) would be possible to validate the
presented results and make more generalizable
conclusions.
The current approach of data collection and pre-
processing is described in (Salamon J., 2017)
including the description of heart rate devices
accuracy. The accuracy of the used measurement
devices and uncertainty is sufficient to determine the
slope of the heart rate used in this paper.
Nevertheless, other experiments validated one of
the devices (Fitbit Charge HR) with various results
(Montoye A. H. K., 2017), (Dooley E. E., 2017) and
(Boudreaux B. D., 2017). It leads to the conclusion
the uncertainty modeling for more complex methods
(such as DBN or HMM) needs to be considered for
further research.
ACKNOWLEDGEMENTS
The work is supported by the UWB grant SGS-2016-
018 Data and Software Engineering for Advanced
Applications.
REFERENCES
Choi J., Gutierrez-Osuna R., 2009. Using Heart Rate
Monitors to Detect Mental Stress. In Proceedings of the
2009 Sixth International Workshop on Wearable and
Implantable Body Sensor Networks (BSN ’09). IEEE
Computer Society, Washington, DC, USA, 219–223.
https://doi.org/10.1109/BSN.2009.13.
Selye H.,1975. Confusion and Controversy in the Stress
Field. Journal of Human Stress 1, 2 (1975), 37–44.
https://doi.org/10.1080/0097840X.1975.9940406.
Blangsted A. K. et al., Hjortskov N., Rissen D., 2004. The
effect of mental stress on heart rate variability and
blood pressure during computer work. Eur J Appl
Physiol (2004). https://doi.org/10.1007/s00421-004-1055-z.
Spaepen A., Taelman J., Vandeput S. and Hu S. V., 2009.
Influence of mental stress on heart rate and heart rate
variability. In Proceedings 4th European Conference of
the International Federation for Medical and
Biological Engineering (IFMBE ’09), 1366–1369.
Joulin A., Grave E., Bojanowski P., and Mikolov T., 2016.
Bag of Tricks for Efficient Text Classification. (2016).
http://arxiv.org/abs/1607.01759.
Vassanyi I., Salai M., and Kosa I., 2016. Stress Detection
Using Low Cost Heart Rate Sensors. Journal of
Healthcare Engineering 2016 (2016). https://
doi.org/10.1155/2016/5136705.
Mcduff D., Gontarek S., and Picard R.. 2014. Remote
Measurement of Cognitive Stress via Heart Rate
Variability. Annual International Conference of the
IEEE Engineering in Medicine and Biology Society.
Mitra A., 2008. Diabetes and stress: a review.
Ethnomedicine 2 (2008), 131–135.
Schubert C., Lambertz M., Nelesen R.A., Bardwell W, Choi
J. B., and Dimsdale J.E., 2009. Effects of stress on heart
rate complexity — A comparison between short-term
and chronic stress. Biological psychology 80, 3 (03
2009),325–332.
https://doi.org/10.1016/j.biopsycho.2008.11.005.
Stress Dichotomy using Heart Rate and Tweet Sentiment
531