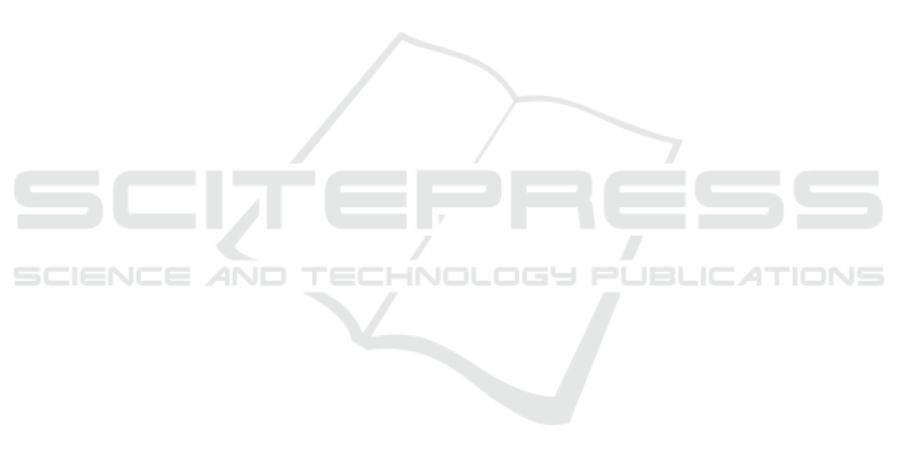
Farhadi, A., Endres, I., Hoiem, D., and Forsyth, D. (2009).
Describing objects by their attributes. In CVPR.
Felzenszwalb, P. F., Girshick, R. B., McAllester, D.,
and Ramanan, D. (2010). Object detection with
discriminatively trained part-based models. IEEE
Trans. on Pattern Analysis and Machine Intelligence,
32(9):1627–1645.
Gan, C., Yang, T., and Gong, B. (2016). Learning attributes
equals multi-source domain generalization. In CVPR.
He, K., Zhang, X., Ren, S., and Sun, J. (2016). Deep resi-
dual learning for image recognition. In CVPR.
Hillel, A. B. and Weinshall, D. (2007). Subordinate class
recognition using relational object models. In NIPS.
Huang, S., Xu, Z., Tao, D., and Zhang, Y. (2016). Part-
stacked CNN for fine-grained visual categorization. In
CVPR.
Juneja, M., Vedaldi, A., Jawahar, C. V., and Zisserman, A.
(2013). Blocks that shout: Distinctive parts for scene
classification. In CVPR.
Khosla, A., Jayadevaprakash, N., Yao, B., and Li, F.-F.
(2011). Novel dataset for fine-grained image catego-
rization: Stanford dogs. In CVPR Workshops.
Kovashka, A., Parikh, D., and Grauman, K. (2015). Whitt-
lesearch: Interactive image search with relative attri-
bute feedback. International Journal of Computer Vi-
sion, 115(2):185–210.
Kovashka, A., Vijayanarasimhan, S., and Grauman, K.
(2011). Actively selecting annotations among objects
and attributes. In ICCV.
Krizhevsky, A., Sutskever, I., and Hinton, G. E. (2012).
ImageNet classification with deep convolutional neu-
ral networks. In NIPS.
Kumar, N., Belhumeur, P. N., Biswas, A., Jacobs, D. W.,
Kress, W. J., Lopez, I. C., and Soares, J. V. (2012). Le-
afsnap: A computer vision system for automatic plant
species identification. In ECCV. Springer.
Lampert, C. H., Nickisch, H., and Harmeling, S. (2014).
Attribute-based classification for zero-shot visual ob-
ject categorization. IEEE Trans. on Pattern Analysis
and Machine Intelligence, 36(3):453–465.
Lin, D., Shen, X. Y., Lu, C. W., and Jia, J. Y. (2015). Deep
LAC: Deep localization, alignment and classification
for fine-grained recognition. In CVPR.
Maji, S., Rahtu, E., Kannala, J., Blaschko, M., and Vedaldi,
A. (2013). Fine-grained visual classification of air-
craft. Technical report.
Miller, G. A. (1995). WordNet: A lexical database for eng-
lish. Communications of ACM, 38(11):39–41.
Modolo, D. and Ferrari, V. (2017). Learning semantic part-
based models from google images. IEEE Trans. on
Pattern Analysis and Machine Intelligence, PP(99):1–
1.
Movshovitz-Attias, Y., Yu, Q., Stumpe, M. C., Shet, V., Ar-
noud, S., and Yatziv, L. (2015). Ontological supervi-
sion for fine grained classification of street view sto-
refronts. In CVPR.
Ouyang, W., Li, H., Zeng, X., and Wang, X. (2015). Le-
arning deep representation with large-scale attributes.
In ICCV.
Porello, D., Setti, F., Ferrario, R., and Cristani, M. (2013).
Multiagent socio-technical systems. an ontological
approach. In COIN Workshop, in conj. with AAMAS.
Qiao, R., Liu, L., Shen, C., and van den Hengel, A. (2016).
Less is more: zero-shot learning from online textual
documents with noise suppression. In CVPR.
Rejeb Sfar, A., Boujemaa, N., and Geman, D. (2013). Van-
tage feature frames for fine-grained categorization. In
CVPR.
Rohrbach, M., Stark, M., and Schiele, B. (2011). Evaluating
knowledge transfer and zero-shot learning in a large-
scale setting. In CVPR. IEEE.
Romera-Paredes, B. and Torr, P. (2015). An embarrassingly
simple approach to zero-shot learning. In ICML.
Salakhutdinov, R., Tenenbaum, J. B., and Torralba, A.
(2013). Learning with hierarchical-deep models.
IEEE Trans. on Pattern Analysis and Machine Intel-
ligence, 35(8):1958–1971.
Setti, F., Cheng, D.-S., Abdulhak, S. A., Ferrario, R., and
Cristani, M. (2013). Ontology-assisted object de-
tection: Towards the automatic learning with internet.
In ICIAP. Springer.
Sharmanska, V. and Quadrianto, N. (2017). In the era of
deep convolutional features: Are attributes still useful
privileged data? In Visual Attributes, pages 31–48.
Springer.
Sharmanska, V., Quadrianto, N., and Lampert, C. H. (2013).
Learning to rank using privileged information. In
ICCV.
Simonyan, K. and Zisserman, A. (2014). Very deep con-
volutional networks for large-scale image recognition.
ICLR.
Szegedy, C., Liu, W., Jia, Y., Sermanet, P., Reed, S., Angue-
lov, D., Erhan, D., Vanhoucke, V., and Rabinovich, A.
(2015). Going deeper with convolutions. In CVPR.
Town, C. (2006). Ontological inference for image and video
analysis. Machine Vision and Applications, 17(2):94–
115.
Vapnik, V. and Vashist, A. (2009). A new learning para-
digm: Learning using privileged information. Neural
networks, 22(5):544–557.
Wang, D., Shen, Z., Shao, J., Zhang, W., Xue, X., and
Zhang, Z. (2015a). Multiple granularity descriptors
for fine-grained categorization. In ICCV.
Wang, Z., Wang, X., and Wang, G. (2015b). Learning fine-
grained features via a cnn tree for large-scale classifi-
cation. arXiv preprint arXiv:1511.04534.
Xu, Z., Liu, Y., Mei, L., Hu, C., and Chen, L. (2015). Se-
mantic based representing and organizing surveillance
big data using video structural description technology.
Journal of Systems and Software, 102:217–225.
Yu, A. and Grauman, K. (2017). Fine-grained comparisons
with attributes. In Visual Attributes, pages 119–154.
Springer.
Zhang, N., Donahue, J., Girshick, R., and Darrell, T.
(2014). Part-based R-CNNs for fine-grained category
detection. In ECCV.
Zhang, N., Farrell, R., Iandola, F., and Darrell, T. (2013).
Deformable part descriptors for fine-grained recogni-
tion and attribute prediction. In ICCV.
Zhao, B., Feng, J., Wu, X., and Yan, S. (2017). A survey on
deep learning-based fine-grained object classification
and semantic segmentation. International Journal of
Automation and Computing, pages 1–17.
VISAPP 2018 - International Conference on Computer Vision Theory and Applications
392