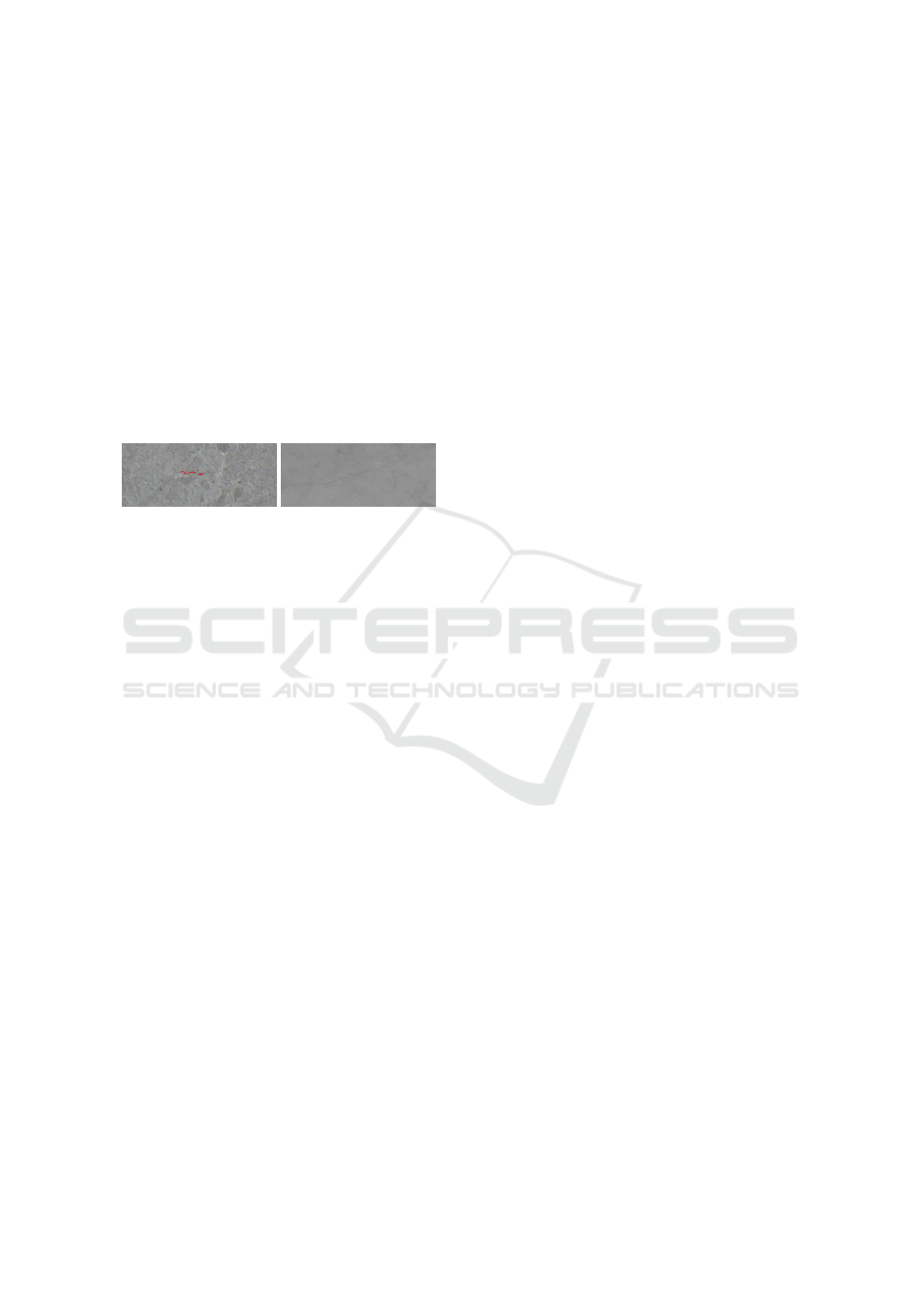
can easily give only the interested cracks thanks to our
direction computation.
We can finally mention that small numbers of false
positive are mainly due to the unsolved problem of
combining multiple interacting physical defects at the
same location or very low-contrasted cracks (see fig-
ure 5). In the literature, we can regret the lack of stan-
dardization and comprehensive representation of de-
fect information.
We can mention that in most of crack detection
works that are reported to be efficient, only few ex-
amples are used for the accuracy evaluation. We use
a much bigger database in our evaluations. The lack
of public available large datasets, that should leverage
crack detection methods, limits the potential of direct
comparisons between them, (Salman et al., 2013).
Figure 5: (Left)Example of false detection. (Right)Example
of missed detection.
6 CONCLUSION
In this article, we propose a new line detector called
FLASH and applied here in concrete structures for
crack detection. The detected micro-lines are very
stable and efficient to straight line detection. The pro-
cess for graph construction is cheap in time. Some
informations about the graph and orientation can be
used to describe an object. The experimental results
show better reliability for crack detection than the
existing algorithms. We can use it in an embedded
system like a UAV to monitor automatically a con-
crete structure. We will present more examples at
liris.cnrs.fr/∼yfaula/.
ACKNOWLEDGEMENTS
This work is supported by Smart Aerial Machines and
SNCF R
´
eseau. Thanks to Jean Delzers and Alain
Morice for their suggestions.
REFERENCES
Anwar, S. A. and Abdullah, M. Z. (2014). Micro-crack
detection of multicrystalline solar cells featuring an
improved anisotropic diffusion filter and image seg-
mentation technique. EURASIP Journal on Image and
Video Processing, 2014(1):15.
Bay, H., Ess, A., Tuytelaars, T., and Van Gool, L. (2008).
Speeded-Up Robust Features (SURF). Comput. Vis.
Image Underst., 110(3):346–359.
Duda, R. O. and Hart, P. E. (1972). Use of the Hough
Transformation to Detect Lines and Curves in Pic-
tures. Commun. ACM, 15(1):11–15.
Fujita, Y. and Hamamoto, Y. (2010). A robust automatic
crack detection method from noisy concrete surfaces.
Machine Vision and Applications, 22(2):245–254.
Gioi, R. G. v., Jakubowicz, J., Morel, J.-M., and Randall,
G. (2012). LSD: a Line Segment Detector. Image
Processing On Line, 2:35–55.
Jahanshahi, M. R., Masri, S. F., Padgett, C. W., and
Sukhatme, G. S. (2011). An innovative methodology
for detection and quantification of cracks through in-
corporation of depth perception. Machine Vision and
Applications, 24(2):227–241.
Koch, C., Georgieva, K., Kasireddy, V., Akinci, B., and
Fieguth, P. (2015). A review on computer vision based
defect detection and condition assessment of concrete
and asphalt civil infrastructure. Advanced Engineer-
ing Informatics, 29(2):196–210.
Leutenegger, S., Chli, M., and Siegwart, R. Y. (2011).
BRISK: Binary Robust invariant scalable keypoints.
In 2011 International Conference on Computer Vi-
sion, pages 2548–2555.
Lowe, D. G. (2004). Distinctive Image Features from Scale-
Invariant Keypoints. Int. J. Comput. Vision, 60(2):91–
110.
Mikolajczyk, K. and Schmid, C. (2005). A perfor-
mance evaluation of local descriptors. IEEE Trans-
actions on Pattern Analysis and Machine Intelligence,
27(10):1615–1630.
Mohan, A. and Poobal, S. (2017). Crack detection us-
ing image processing: A critical review and analysis.
Alexandria Engineering Journal.
Nguyen, H.-N., Kam, T.-Y., and Cheng, P.-Y. (2014). An
Automatic Approach for Accurate Edge Detection
of Concrete Crack Utilizing 2d Geometric Features
of Crack. Journal of Signal Processing Systems,
77(3):221–240.
Pereira, F. C. and Pereira, C. E. (2015). Embedded
Image Processing Systems for Automatic Recogni-
tion of Cracks using {UAVs}. IFAC-PapersOnLine,
48(10):16 – 21. 2nd {IFAC} Conference on Embed-
ded Systems, Computer Intelligence and Telematics
{CESCIT} 2015Maribor, Slovenia, 22-24 June 2015.
Rey-Otero, I., Delbracio, M., and Morel, J.-M. (2014).
Comparing Feature Detectors: A bias in the repeata-
bility criteria, and how to correct it. arXiv:1409.2465
[cs]. arXiv: 1409.2465.
Rosten, E., Porter, R., and Drummond, T. (2010). Faster
and Better: A Machine Learning Approach to Corner
Detection. IEEE Transactions on Pattern Analysis and
Machine Intelligence, 32(1):105–119.
Rublee, E., Rabaud, V., Konolige, K., and Bradski, G.
(2011). ORB: An efficient alternative to SIFT or
SURF. In 2011 International Conference on Com-
puter Vision, pages 2564–2571.
FLASH: A New Key Structure Extraction used for Line or Crack Detection
451