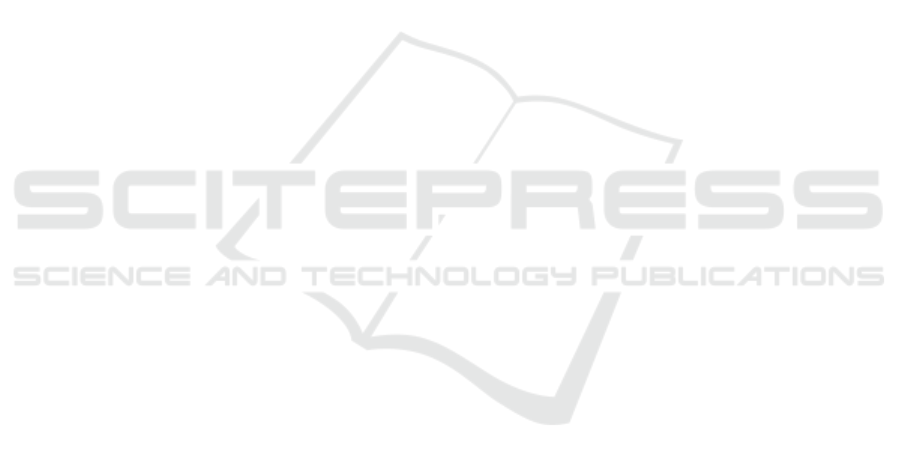
development for computational biology and bioinfor-
matics. Genome biology, 5(10):R80.
Hardcastle, T. J. and Kelly, K. A. (2010). bayseq: empir-
ical bayesian methods for identifying differential ex-
pression in sequence count data. BMC bioinformatics,
11(1):422.
Huber, W., Carey, V. J., Gentleman, R., Anders, S., Carlson,
M., Carvalho, B. S., Bravo, H. C., Davis, S., Gatto, L.,
Girke, T., et al. (2015). Orchestrating high-throughput
genomic analysis with bioconductor. Nature methods,
12(2):115–121.
Kim, D., Pertea, G., Trapnell, C., Pimentel, H., Kelley,
R., and Salzberg, S. L. (2013). Tophat2: accurate
alignment of transcriptomes in the presence of inser-
tions, deletions and gene fusions. Genome biology,
14(4):R36.
Lee, C.-Y., Chiu, Y.-C., Wang, L.-B., Kuo, Y.-L., Chuang,
E. Y., Lai, L.-C., and Tsai, M.-H. (2013). Common ap-
plications of next-generation sequencing technologies
in genomic research. Translational Cancer Research,
2(1):33–45.
Li, J., Witten, D. M., Johnstone, I. M., and Tibshirani, R.
(2012). Normalization, testing, and false discovery
rate estimation for rna-sequencing data. Biostatistics,
13(3):523–538.
Li, Y., Rao, X., Mattox, W. W., Amos, C. I., and Liu, B.
(2015). Rna-seq analysis of differential splice junc-
tion usage and intron retentions by dexseq. PloS one,
10(9):e0136653.
Love, M. I., Huber, W., and Anders, S. (2014). Moderated
estimation of fold change and dispersion for rna-seq
data with deseq2. Genome biology, 15(12):550.
Rajkumar, A. P., Qvist, P., Lazarus, R., Lescai, F., Ju, J.,
Nyegaard, M., Mors, O., Børglum, A. D., Li, Q., and
Christensen, J. H. (2015). Experimental validation of
methods for differential gene expression analysis and
sample pooling in rna-seq. BMC genomics, 16(1):548.
Rapaport, F., Khanin, R., Liang, Y., Pirun, M., Krek, A.,
Zumbo, P., Mason, C. E., Socci, N. D., and Betel, D.
(2013). Comprehensive evaluation of differential gene
expression analysis methods for rna-seq data. Genome
biology, 14(9):3158.
Robinson, M. D., McCarthy, D. J., and Smyth, G. K. (2010).
edger: a bioconductor package for differential expres-
sion analysis of digital gene expression data. Bioin-
formatics, 26(1):139–140.
Shi, L., Campbell, G., Jones, W., Campagne, F., Wen, Z.,
Walker, S., Su, Z., and Chu, T. Goodsaid, 373 fm,
pusztai, l., et al.(2010). the microarray quality control
(maqc)-ii study of common practices 374 for the de-
velopment and validation of microarray-based predic-
tive models. Nature Biotechnology, 375:28.
Shi, L., Reid, L., Jones, W., Shippy, R., Warrington, J.,
Baker, S., Collins, P., and de Longueville, F. (2006).
Es et al. kawasaki, and maqc consortium. the microar-
ray quality control (maqc) project shows inter-and in-
traplatform reproducibility of gene expression mea-
surements. Nat Biotechnol, 24:1151–61.
Smedley, D., Haider, S., Durinck, S., Pandini, L., Provero,
P., Allen, J., Arnaiz, O., Awedh, M. H., Baldock,
R., Barbiera, G., et al. (2015). The biomart com-
munity portal: an innovative alternative to large, cen-
tralized data repositories. Nucleic acids research,
43(W1):W589–W598.
Smyth, G. K. (2005). Limma: linear models for microar-
ray data. In Bioinformatics and computational biology
solutions using R and Bioconductor, pages 397–420.
Springer.
Trapnell, C., Hendrickson, D. G., Sauvageau, M., Goff, L.,
Rinn, J. L., and Pachter, L. (2013). Differential analy-
sis of gene regulation at transcript resolution with rna-
seq. Nature biotechnology, 31(1):46–53.
Young, M. D., McCarthy, D. J., Wakefield, M. J., Smyth,
G. K., Oshlack, A., and Robinson, M. D. (2012).
Differential expression for rna sequencing (rna-seq)
data: mapping, summarization, statistical analysis,
and experimental design. In Bioinformatics for High
Throughput Sequencing, pages 169–190. Springer.
geneEx - A Novel Tool to Assess Differential Expression from Gene and Exon Sequencing Data
181