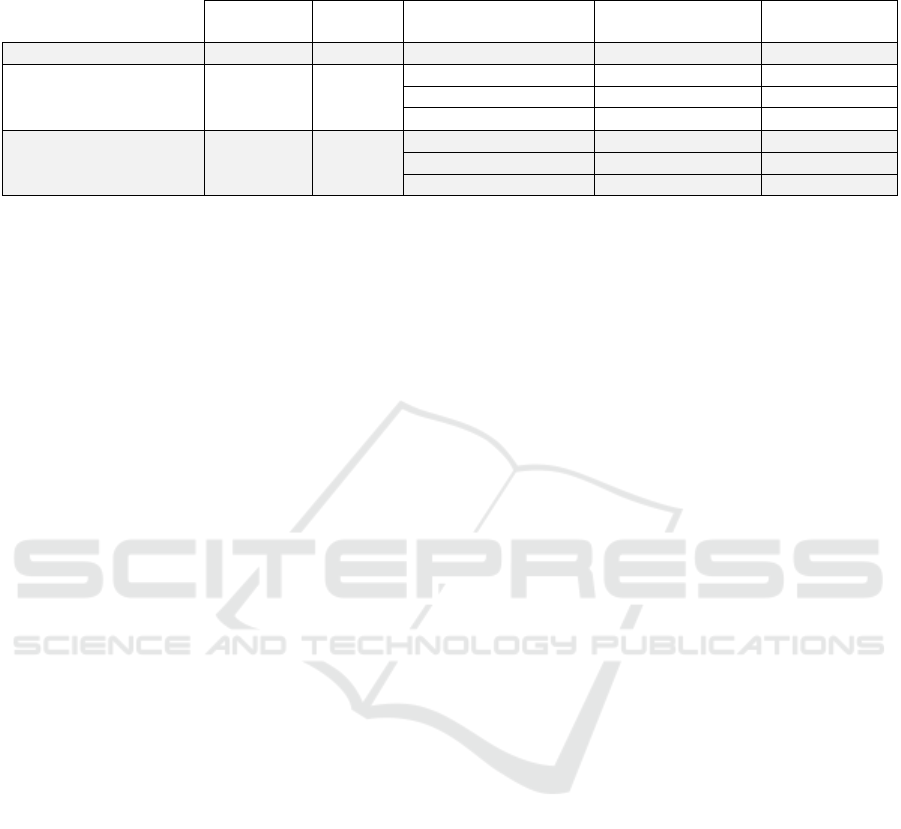
Table 3: Related work: accuracy and precision of the extraction of gait phase durations in older adults using inertial sensors.
NA: not available.
turning walking episodes, and (5) the extraction of
spatial gait parameters from the heel and toe
accelerations, taking advantage from the proposed
algorithm that enables splitting the gait cycle time
into small time intervals and thus the drift from
successive integration in these small intervals could
be minimized. In this context, i.e., the extraction of
spatial gait parameters from accelerometer data, we
obtained promising preliminary results reported in
(Boutaayamou et al., 2017b).
ACKNOWLEDGEMENTS
The authors would like to thank all the participants
who accepted to participate as volunteers to the
walking tests of the present study.
REFERENCES
Allali, G., Launay, C.P., Blumen, H.M., et al., (2017). Falls,
cognitive impairment, and gait performance: results
from the good initiative. J. of the American Medical
Directors Association, 18(4):335–40.
Boutaayamou, M., Schwartz, C., Stamatakis, J., et al.,
(2015). Development and validation of an
accelerometer-based method for quantifying gait
events. Medical Engineering & Physics, 37:226–232.
Boutaayamou, M., Denoël, V., Brüls, O., et al., (2017a).
Algorithm for temporal gait analysis using wireless
foot-mounted accelerometers. In: Fred A., Gamboa H.
(eds) Biomedical Engineering Systems and
Technologies. Communications in Computer and
Information Science, Springer, 690:236–254.
Boutaayamou, M., Schwartz, C., Denoël, V., Croisier, J.-
L., Verly, J.G., Garraux, G., and Brüls, O., (2017b). A
novel accelerometer-based method for stride length
estimation. In: 39
th
Annual International Conference of
IEEE Engineering in Medicine and Biology Society,
Jeju, South Korea.
Gillain, S., Dramé, M., Lekeu, F., et al., (2015). Gait speed
or gait variability, which one to use as a marker of risk
to develop Alzheimer disease? A pilot study. Aging
Clinical and Experimental Research, 28(2):249–255.
Gillain, S., Boutaayamou, M., Dardenne, N., et al., (2017).
Data set of healthy old people assessed for three
walking conditions using accelerometric and opto-
electronic methods. Aging Clinical and Experimental
Research, 1–9.
Hartmann, A., Luzi, S., Murer, K., de Bie, R.A., de Bruin,
E.D., (2009). Concurrent validity of a trunk tri-axial
accelerometer system for gait analysis in older adults.
Gait & Posture, 29(3):444–448.
Hausdorff, J.M., Rios, D.A., and Edelberg, H.K., (2001).
Gait variability and fall risk in community-living older
adults: a 1-year prospective study. Archives of Physical
Medicine and Rehabilitation, 82.
Micó-Amigo, M.E., Kingma, I., Ainsworth, E., et al.,
(2016). A novel accelerometry-based algorithm for the
detection of step durations over short episodes of gait in
healthy elderly. J. NeuroEngineering and
Rehabilitation, 13:38.
Moe-Nilssen, R., and Helbostad, J.L., (2004). Estimation of
gait cycle characteristics by trunk accelerometry.
Journal of Biomechanics, 37(1):121–126.
Rampp, A., Barth, J., Schülein, S., Gaßmann, et al. (2015).
Inertial sensor-based stride parameter calculation from
gait sequences in geriatric patients. IEEE Transactions
on Biomedical Engineering, 62(4):1089–1097.
Rueterbories, J., Spaich., E.G., Larsen, B., and Andersen
O.K., (2010). Methods for gait event detection and
analysis in ambulatory systems. Medical Engineering
and Physics, 32(6):545–552.
Trojaniello, D., Cereatti, A., Pelosin, et al., (2014).
Estimation of step-by-step spatio-temporal parameters
of normal and impaired gait using shank-mounted
magneto-inertial sensors: application to elderly,
hemiparetic, parkinsonian and choreic gait. Journal of
Neuroengineering & Rehabilitation, 11:152.
Validated Assessment of Gait Sub-Phase Durations in Older Adults using an Accelerometer-based Ambulatory System
255