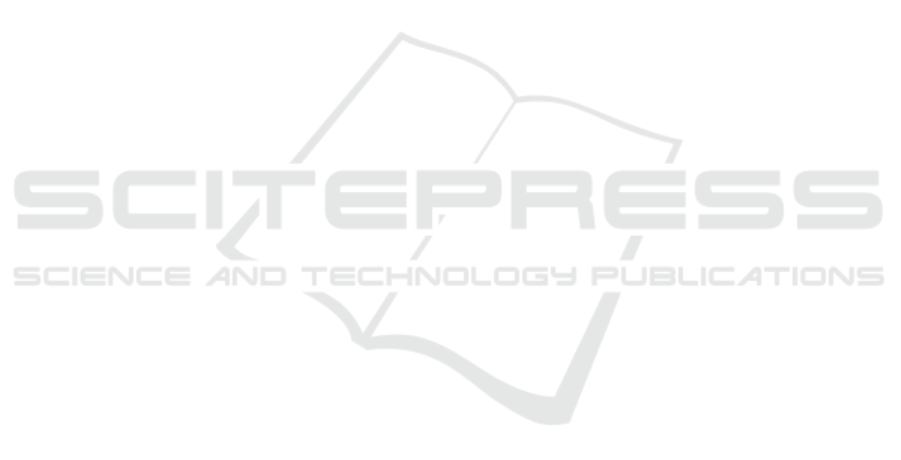
ory for warping. In European Conference on Compu-
ter Vision (ECCV), volume 3024, pages 25–36. Lec-
ture Notes in Computer Science.
Butler, D. J., Wulff, J., Stanley, G. B., and Black, M. J.
(2012). A naturalistic open source movie for optical
flow evaluation. In A. Fitzgibbon et al. (Eds.), editor,
European Conf. on Computer Vision (ECCV), Part IV,
LNCS 7577, pages 611–625. Springer-Verlag.
Chen, Z., Jin, H., Lin, Z., Cohen, S., and Wu, Y. (2013).
Large displacement optical flow from nearest neig-
hbor fields. In IEEE Conference on Computer Vision
and Pattern Recognition (CVPR), pages 2443–2450.
Fortun, D., Bouthemy, P., and Kervrann, C. (2016). Aggre-
gation of local parametric candidates with exemplar-
based occlusion hangling for optical flow. Computer
Vision and Image Undestanding, pages 81–94.
Horn, B. K. and Schunck, B. H. (1981). Determining optical
flow. Artificial Intelligence, 17:185–203.
Ince, S. and Konrad., J. (2008). Occlusion-aware optical
flow estimation. IEEE Transactions on Image Proces-
sing, 17(8):1443–1451.
Kennedy, R. and Taylor, C. J. (2015). Optical flow with geo-
metric occlusion estimation and fusion of multiple fra-
mes. In EMMCVPR 2015, Hong Kong, China, Janu-
ary 13-16, 2015. Proceedings, pages 364–377. Sprin-
ger International Publishing.
Kr
¨
ahenb
¨
uhl, P. and Koltun., V. (2012). Efficient nonlocal
regularization for optical flow. In European Confe-
rence on Computer Vision (ECCV), pages 356–369.
Springer.
Nagel, H.-H. and Ekelman, W. (1986). An investigation
of smoothness constraints for the estimation of displa-
cement vector fields from image sequences. Pattern
Analysis and Machine Intelligence, 6(5):565–593.
Palomares, R. P., Haro, G., and Ballester, C. (2015).
A rotation-invariant regularization term for optical
flow related problems. Lectures Notes in Computer
Science, 9007:304–319.
Palomares, R. P., Meinhardt-Llopis, E., Ballester, C., and
Haro, G. (2017). Faldoi: A new minimization strategy
for large displacement variational optical flow. Jour-
nal of Mathematical Imaging and Vision, 58(1):27–
46.
Ranftl, R., Bredies, K., and Pock, T. (2014). Non-local total
generalized variation for optical flow estimation. In
Computer Vision–ECCV, pages 439–454. Springer.
S
´
anchez, J., Salgado, A., and Monz
´
on, N. (2014). Preser-
ving accurate motion contours with reliable parameter
selection. In IEEE International Conference on Image
Processing (ICIP), pages 209–213.
Scharstein, S. and Szeliski, R. (2002). A taxonomy and
evaluation of dense two-frame stereo correspondence
algorithms. International journal of computer vision,
47 :7–42.
Steinbruecker, F. and Pock, T. (2009). Large displacement
optical flow computation without warping. In Interna-
tional Conference on Computer Vision, pages 1609–
1614.
Stoll, M., Volz, S., and Bruhn., A. (2012). Adaptive in-
tegration of features matches into variational optical
flow methods. In Proc. of the Asian Conference in
Computer Vision (ACCV), pages 1–14.
Strekalovskiy, E., Chambolle, A., and Cremers, D. (2014).
Convex relaxation of vectorial problems with coupled
regularization. SIAM J. Imaging Sciences, 7(1 ):294–
336.
Sun, D., Roth, S., and Black, M. J. (2010). Secrets of opti-
cal flow estimation and their principles. In IEEE Con-
ference on Computer Vision and Pattern Recognition,
pages 2432–2439.
Sun, D., Roth, S., and Black, M. J. (2014). A quantitative
analysis of current practices in optical flow estimation
and the principles behind them. International Journal
of Computer Vision, 2 (106):115–137.
Timofte, R. and Van Gool, L. (2015). Sparse flow: Sparse
matching for small to large displacement optical flow.
IEEE WACV, 00:1100–1106.
Weinzaepfel, P., Revaud, J., Harchaoui, Z., and Schmid, C.
(2013). Deepflow: Large displacement optical flow
with deep matching. In IEEE International Confe-
rence on Computer Vision, Sydney, Australia, pages
1385–1392.
Werlberger, M., Pock, T., and Bischof, H. (2010). Mo-
tion estimation with non-local total variation regula-
rization. In IEEE Conference on Computer Vision and
Pattern Recognition(CVPR), pages 2464–2471.
Werlberger, M., Trobin, W., Pock, T., Wedel, A., Cremers,
D., and Bischof, H. (2009). Anisotropic huber-l1 op-
tical flow. In Proceedings of the BMVC.
Xu, L., Jia, J., and Matsushita, Y. (2012). Motion detail
preserving optical flow estimation. In Pattern Analy-
sis and Machine Intelligence, IEEE Transactions on,
volume 34, pages 1744–1757.
Zach, C., Pock, T., and Bischof, H. (2007). A duality
based approach for realtime tv-l1 optical flow. In
Proceedings of the 29th DAGM Conference on Pat-
tern Recognition, pages 214–223. Berlin, Heidelberg,
Springer-Verlag.
Zimmer, H., Bruhn, A., and J., W. (2011). Optic flow in
harmony. International Journal of Computer Vision,
93(3):368–388.
Jointly Optical Flow and Occlusion Estimation for Images with Large Displacements
595