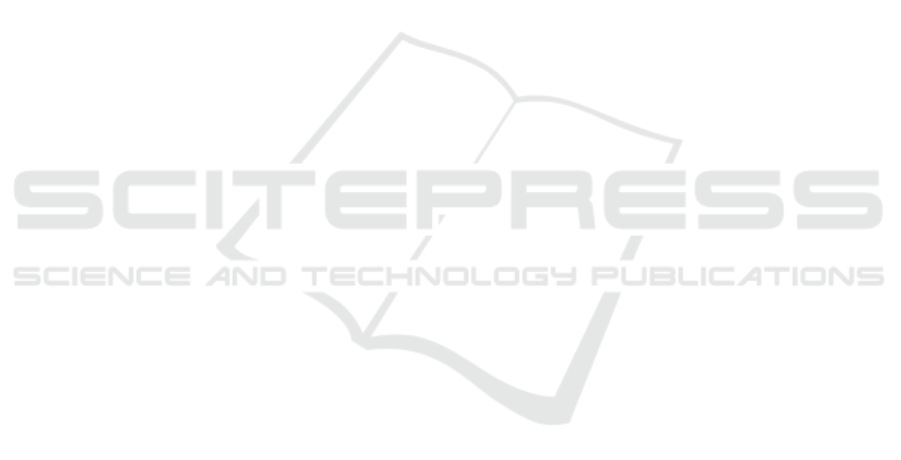
onal Conference of the IEEE Engineering in Medicine
and Biology Society (EMBC), pages 419–422.
Klonovs, J., Haque, M. A., Krueger, V., Nasrollahi, K.,
Andersen-Ranberg, K., Moeslund, T. B., and Spaich,
E. G. (2016). Monitoring Technology, pages 49–84.
Springer International Publishing, Cham.
Kotsia, I. and Pitas, I. (2007). Facial expression recognition
in image sequences using geometric deformation fea-
tures and support vector machines. IEEE Transactions
on Image Processing, 16(1):172–187.
Lajevardi, S. and Hussain, Z. (2010). Novel higher-order
local autocorrelation-like feature extraction methodo-
logy for facial expression recognition. IET Image Pro-
cessing, 4:114–119(5).
Lauterbach, M. D., Notarangelo, P. L., Nichols, S. J.,
Lane, K. S., and Koliatsos, V. E. (2015). Diagnos-
tic and treatment challenges in traumatic brain injury
patients with severe neuropsychiatric symptoms: in-
sights into psychiatric practice. Neuropsychiatr Dis
Treat, 11:1601–1607.
Li, F., Zhao, C., Xia, Z., Wang, Y., Zhou, X., and Li, G.-Z.
(2012). Computer-assisted lip diagnosis on traditio-
nal chinese medicine using multi-class support vector
machines. BMC Complementary and Alternative Me-
dicine, 12(1):127.
Li, S. Z. and Jain, A. K. (2011). Handbook of Face Recog-
nition. Springer Publishing Company, Incorporated,
2nd edition.
li Tian, Y. (2004). Evaluation of face resolution for expres-
sion analysis. In 2004 Conference on Computer Vision
and Pattern Recognition Workshop, pages 82–82.
Lyons, M. J., Budynek, J., and Akamatsu, S. (1999). Au-
tomatic classification of single facial images. IEEE
Trans. Pattern Anal. Mach. Intell., 21(12):1357–1362.
Mathias, M., Benenson, R., Pedersoli, M., and Van Gool,
L. (2014). Face Detection without Bells and Whistles,
pages 720–735. Springer International Publishing.
Mehrabian, A. (1968). Communication without words. Psy-
chology Today, 1.2(4):53–56.
Noroozi, F., Marjanovic, M., Njegus, A., Escalera, S., and
Anbarjafari, G. (2017). Audio-visual emotion recog-
nition in video clips. IEEE Transactions on Affective
Computing, (99):1–1.
Palestra, G., Pettinicchio, A., Del Coco, M., Carcagn
`
ı, P.,
Leo, M., and Distante, C. (2015). Improved Perfor-
mance in Facial Expression Recognition Using 32 Ge-
ometric Features, pages 518–528. Springer Internati-
onal Publishing, Cham.
Pantic, M. and Patras, I. (2006). Dynamics of facial expres-
sion: recognition of facial actions and their temporal
segments from face profile image sequences. IEEE
Transactions on Systems, Man, and Cybernetics, Part
B (Cybernetics), 36(2):433–449.
Parkhi, O. M., Vedaldi, A., and Zisserman, A. (2015). Deep
face recognition. In British Machine Vision Confe-
rence.
Poursaberi, A., Noubari, H. A., Gavrilova, M., and Ya-
nushkevich, S. N. (2012). Gauss–laguerre wavelet tex-
tural feature fusion with geometrical information for
facial expression identification. EURASIP Journal on
Image and Video Processing, 2012(1):17.
Ravichander, A., Vijay, S., Ramaseshan, V., and Natarajan,
S. (2016). Automated Human Facial Expression Re-
cognition Using Extreme Learning Machines, pages
209–222. Springer International Publishing, Cham.
Rodriguez, P., Cucurull, G., Gonzlez, J., Gonfaus, J. M.,
Nasrollahi, K., Moeslund, T. B., and Roca, F. X.
(2017). Deep pain: Exploiting long short-term me-
mory networks for facial expression classification.
IEEE Transactions on Cybernetics, PP(99):1–11.
Tian, Y. I., Kanade, T., and Cohn, J. F. (2001). Recogni-
zing action units for facial expression analysis. IEEE
Transactions on Pattern Analysis and Machine Intel-
ligence, 23(2):97–115.
Triantafyllidou, D. and Tefas, A. (2016). Face detection ba-
sed on deep convolutional neural networks exploiting
incremental facial part learning. In 2016 23rd Inter-
national Conference on Pattern Recognition (ICPR),
pages 3560–3565.
Uddin, M. Z. and Hassan, M. M. (2015). A depth video-
based facial expression recognition system using ra-
don transform, generalized discriminant analysis, and
hidden markov model. Multimedia Tools and Appli-
cations, 74(11):3675–3690.
Uddin, M. Z., Hassan, M. M., Almogren, A., Alamri, A.,
Alrubaian, M., and Fortino, G. (2017). Facial ex-
pression recognition utilizing local direction-based ro-
bust features and deep belief network. IEEE Access,
5:4525–4536.
Viola, P. and Jones, M. (2001). Rapid object detection
using a boosted cascade of simple features. In Procee-
dings of the 2001 IEEE Computer Society Conference
on Computer Vision and Pattern Recognition. CVPR
2001, volume 1, pages I–511–I–518 vol.1.
Xiong, X. and la Torre, F. D. (2013). Supervised descent
method and its applications to face alignment. In 2013
IEEE Conference on Computer Vision and Pattern Re-
cognition, pages 532–539.
Yan, J., Zhang, X., Lei, Z., and Li, S. Z. (2014). Face de-
tection by structural models. Image and Vision Com-
puting, 32(10):790 – 799. Best of Automatic Face and
Gesture Recognition 2013.
Yoshihara, H., Seo, M., Ngo, T. H., Matsushiro, N., and
Chen, Y. W. (2016). Automatic feature point de-
tection using deep convolutional networks for quan-
titative evaluation of facial paralysis. In 2016 9th
International Congress on Image and Signal Proces-
sing, BioMedical Engineering and Informatics (CISP-
BMEI), pages 811–814.
Zhao, X. and Zhang, S. (2011). Facial expression recogni-
tion based on local binary patterns and kernel discri-
minant isomap. Sensors, 11(10):9573–9588.
VISAPP 2018 - International Conference on Computer Vision Theory and Applications
530