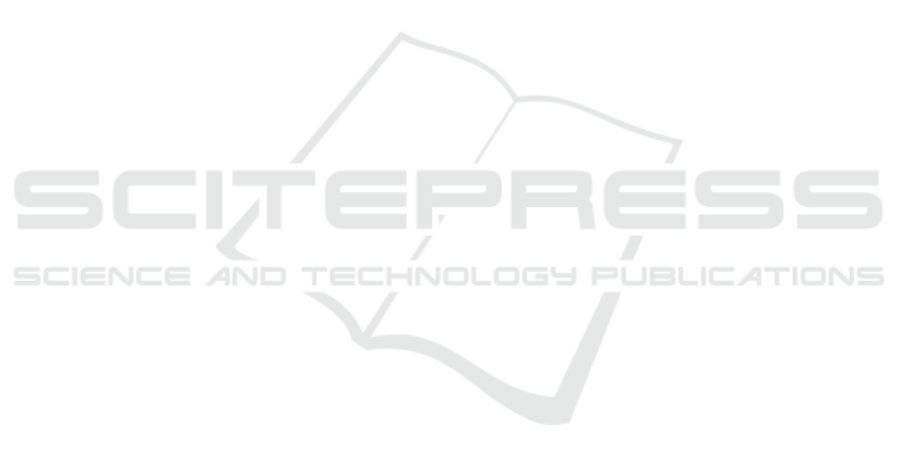
trics. EURASIP Journal on Image and Video Proces-
sing, 2008(1):246309.
Biswas, S. and Babu, R. V. (2013). H. 264 compressed
video classification using histogram of oriented mo-
tion vectors (homv). In Acoustics, Speech and Signal
Processing (ICASSP), 2013 IEEE International Con-
ference on, pages 2040–2044. IEEE.
Davis, J. W. and Keck, M. A. (2005). A two-stage tem-
plate approach to person detection in thermal ima-
gery. In Application of Computer Vision, 2005.
WACV/MOTIONS ’05 Volume 1. Seventh IEEE Works-
hops on, volume 1, pages 364–369.
Kantorov, V. and Laptev, I. (2014). Efficient feature ex-
traction, encoding and classification for action re-
cognition. In Proceedings of the IEEE Conference
on Computer Vision and Pattern Recognition, pages
2593–2600.
Karakasis, E., Bampis, L., Amanatiadis, A., Gasteratos, A.,
and Tsalides, P. (2014). Digital elevation model fusion
using spectral methods. In 2014 IEEE International
Conference on Imaging Systems and Techniques (IST)
Proceedings, pages 340–345. IEEE.
K
¨
as, C. and Nicolas, H. (2009). An approach to trajectory
estimation of moving objects in the h. 264 compressed
domain. Advances in Image and Video Technology,
pages 318–329.
Khosravi, M. and Hassanpour, H. (2017). A novel image
structural similarity index considering image content
detectability using maximally stable extremal region
descriptor. International Journal of Engineering-
Transactions B: Applications, 30(2):172.
Kumar, A. and Gupta, S. (2017). Detection and recognition
of text from image using contrast and edge enhanced
mser segmentation and ocr. IJOSCIENCE (INTERNA-
TIONAL JOURNAL ONLINE OF SCIENCE) Impact
Factor: 3.462, 3(3):07–07.
Ma, Y., Wu, X., Yu, G., Xu, Y., and Wang, Y. (2016). Pe-
destrian detection and tracking from low-resolution
unmanned aerial vehicle thermal imagery. Sensors,
16(4):446.
Matas, J., Chum, O., Urban, M., and Pajdla, T. (2004).
Robust wide-baseline stereo from maximally sta-
ble extremal regions. Image and vision computing,
22(10):761–767.
Park, S.-M. and Lee, J. (2003). Object tracking in mpeg
compressed video using mean-shift algorithm. In In-
formation, Communications and Signal Processing,
2003 and Fourth Pacific Rim Conference on Multime-
dia. Proceedings of the 2003 Joint Conference of the
Fourth International Conference on, volume 2, pages
748–752. IEEE.
Poularakis, S., Avgerinakis, K., Briassouli, A., and Kompat-
siaris, I. (2017). Efficient motion estimation methods
for fast recognition of activities of daily living. Signal
Processing: Image Communication, 53:1–12.
´
Sluzek, A. (2017). Mser and simser regions: A link be-
tween local features and image segmentation. In
Proceedings of the 2017 International Conference on
Computer Graphics and Digital Image Processing,
page 15. ACM.
Sun, X., Ding, J., Dalla Chiara, G., Cheah, L., and Cheung,
N.-M. (2017). A generic framework for monito-
ring local freight traffic movements using computer
vision-based techniques. In Models and Technologies
for Intelligent Transportation Systems (MT-ITS), 2017
5th IEEE International Conference on, pages 63–68.
IEEE.
Sundari, V. K. and Manikandan, M. (2017). Real time im-
plementation of surf based target tracking algorithm.
International Journal on Intelligent Electronics Sys-
tems, 11(1).
Torabi, A., Mass
´
e, G., and Bilodeau, G.-A. (2012). An ite-
rative integrated framework for thermal-visible image
registration, sensor fusion, and people tracking for vi-
deo surveillance applications. Comput. Vis. Image Un-
derst., 116(2):210–221.
Tun, W. N., Tyan, M., Kim, S., Nah, S.-H., and Lee, J.-
W. (2017). Marker tracking with ar. drone for visual-
based navigation using surf and mser algorithms. ,
pages 124–125.
Uemura, H., Ishikawa, S., and Mikolajczyk, K. (2008). Fe-
ature tracking and motion compensation for action re-
cognition. In BMVC, pages 1–10.
Wang, H. and Schmid, C. (2013). Action recognition with
improved trajectories. In Proceedings of the IEEE
International Conference on Computer Vision, pages
3551–3558.
Wu, S., Oreifej, O., and Shah, M. (2011). Action recogni-
tion in videos acquired by a moving camera using mo-
tion decomposition of lagrangian particle trajectories.
In Computer Vision (ICCV), 2011 IEEE International
Conference on, pages 1419–1426. IEEE.
Yeo, C., Ahammad, P., Ramchandran, K., and Sastry, S. S.
(2006). Compressed domain real-time action recogni-
tion. In Multimedia Signal Processing, 2006 IEEE 8th
Workshop on, pages 33–36. IEEE.
Zhang, B., Wang, L., Wang, Z., Qiao, Y., and Wang, H.
(2016). Real-time action recognition with enhanced
motion vector cnns. In Proceedings of the IEEE Con-
ference on Computer Vision and Pattern Recognition,
pages 2718–2726.
Zhang, S., Zhang, L., Gao, R., and Liu, C. (2017). Mobile
robot moving target detection and tracking system. In
Proceedings of the 2017 The 7th International Confe-
rence on Computer Engineering and Networks. 22-23
July, 2017 Shanghai, China (CENet2017).
Pedestrian Detection and Tracking in Thermal Images from Aerial MPEG Videos
495