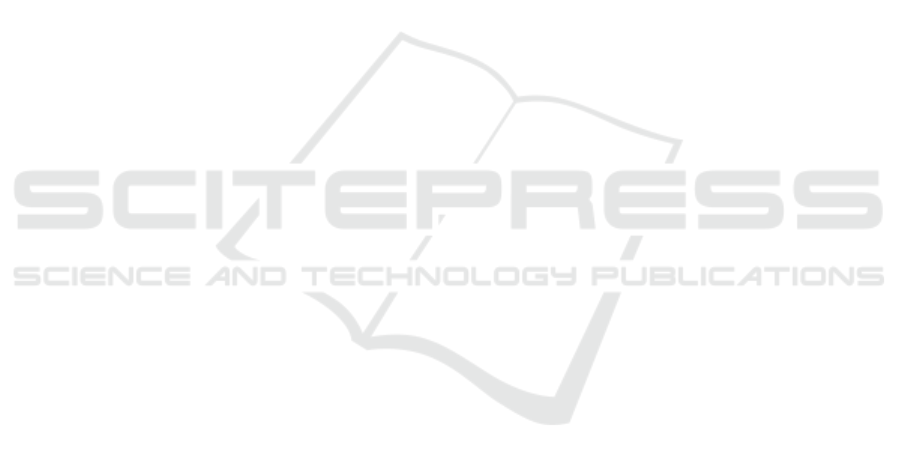
Effective results of application of the decisive
rule and high accuracy of the mathematical
algorithm for the classification of epithelial
neoplasms and non-tumor lesions of the stomach
show the fundamental possibility of formalizing the
microendoscopic structure of the formations, and
hence the possibility of developing their objective
clinical classification and decision support system
for the doctor.
The decision support system with automatic
image identification soon will become an
indispensable part of endoscopic video systems
A method for processing narrow–band
endoscopic images using a highly productive
distributed intellectual analytic decision–making
system was presented. This method allows
improving accuracy and helps avoiding subjectivity
in real-time classification of endoscopic images. It
possesses the following distinctive features:
1. Key points are selected in real time due to
application of FFPT algorithm.
2. Use of SIFT descriptors allows real-time
selection and vectorization of local image
features invariant to scale, shift, rotation and
illumination.
3. Application of the “Bag of visual words”
approach enables processing the whole image
of a mucosal neoplasm (and not just the part
of it examined using histological methods).
4. Use of SVM method for building
classification space.
Examination of a suggested computer algorithm
using 182 endoscopic images todetermine the
neoplasia demonstrated that the accuracy of correct
recognition reaches 85%.
That allows formalization of pit and vessel
pattern descriptors for gastric epithelial neoplasms,
and, in turn, development of a precise and objective
clinical classification. Algorithm efficiency can be
improved by breaking image sets into a larger
number of subgroups according to histological data.
That requires processing wide image set for each
subgroup. It is important that the algorithm is fast
and efficient. That allows using it for processing
video streams and endoscopic images in real time.
ACKNOWLEDGEMENTS
The work was done within the framework of the
project performed by SIAMS Ltd, and supported by
the Ministry of Education and Science of the
Russian Federation (Grant agreement 14.576.21.
0018 dated June 27, 2014. Applied research (project)
UID: RFMEFI57614X0018).
REFERENCES
Buntseva, O. A., Plakhov, R. V., Galkova, Z. V., Fedorov,
E. D., 2014. Modern endoscopic methods of diagnosis
and treatment of precancerous changes and early
gastric cancer.Polyclinic, 2(2), pp. 56-64.
Canny, J., 1986. A computational approach to edge
detection. IEEE Transactions on pattern analysis and
machine intelligence, 6, pp. 679-698.
Dixon, M. F., 2002. Gastrointestinal epithelial neoplasia:
Vienna revisited. Gut, 51(1), pp. 130-131.
Geusebroek, J–M., Smeulders, A. W. M., van de Weijer,
J., 2003. Fast anisotropic Gauss Filtering. IEEE
Transactions on image processing (TIP 2003),
pp. 938–943.
Gubler, E. V., 1978. Computational methods of analysis
and recognition of pathological processes, Medicine.
Leningrad.
Guo, Z., Hall, R. W., 1992. Fast fully parallel thinning
algorithms.CVGIP: Image Understanding, 55(3),
pp. 317–328.
Japanese Gastric Cancer Association, 1998. Japanese
classification of gastric carcinoma - 2nd English
edition. Gastric Cancer, 1, pp.10-24.
Kaprin, A. D., Starinsky, V. V., Petrova, G. V. (ed.), 2017.
Malignant neoplasms in Russia in 2015 (morbidity
and mortality), MSRI named after P.A. Herzen - FGBI
branch of NMRRC of Russian Health Ministry,
Moscow.
Lee, T-C., Lin Y-H., Uedo, N., Wang, H-P., Chang, H-T.,
Hung C-W., 2013. Computer-aided diagnosis in
endoscopy: A novel application toward automatic
detection ofabnormal lesions on magnifying narrow-
band imagingendoscopy in the stomach.In Proc. 35th
IEEE AnnualInt. Conf. of the Engineering in Medicine
and Biology Society (EMBC), pp. 4430–4433.
Liedlgruber, M., Uhl, A., 2011. Computer-aided decision
support systems for endoscopy in the gastrointestinal
tract: a review. IEEE reviews in biomedical
engineering, 4, pp 73-88.
Lingua, A., Marenchino, D., Nex, F., 2009.Performance
Analysis of the SIFT Operator for Automatic Feature
Extractionand Matching in Photogrammetric
Applications. Sensors, 9(5), pp. 3745–3766.
Lowe, D. G. 2004. Distinctive image features from scale-
invariant keypoints. International journal of computer
vision, 60(2), pp. 91-110.
Miyaki, R., Yoshida, S., Tanaka, S., Kominami, Y.,
Sanomura, Y., Matsuo, T., Oka, S., Raytchev, B.,
Tamaki, T., Koide, T., Kaneda, K., Yoshihara, M.,
Chayama, K., 2013. Quantitative identification of
mucosal gastric cancer under magnifying endoscopy
withflexible spectral imaging color enhancement.J.
Gastroenterol. Hepatology, 28(5), pp. 841–847.
Osawa, H., Yamamoto, H., Miura, Y., Ajibe, H., Shinhata,
The Computer-aided Diagnostics of Gastric Lesions by using High Definition Narrow-band Imaging Endoscopy and Real-time Pattern
Recognition System
619