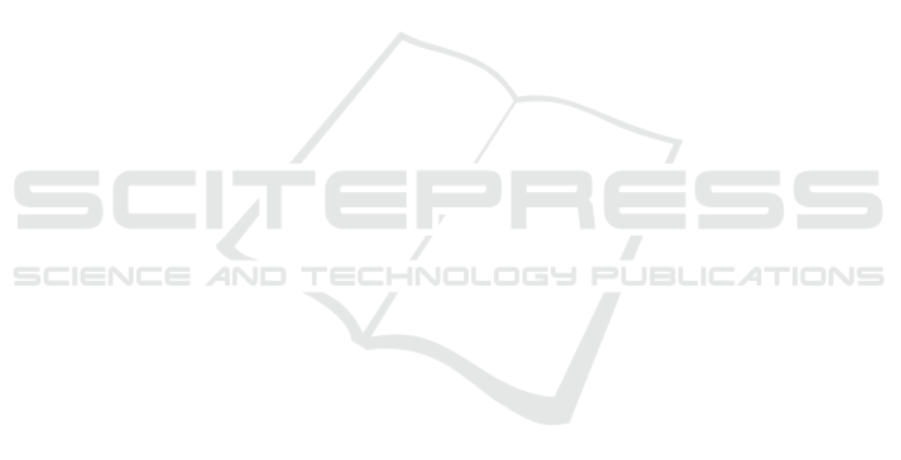
4 DISCUSSIONS AND
CONCLUSIONS
The present works shows results of the artificial
neural networks application in task of the arterial
hypertension diagnostics. The distinctive feature of
the present work is application of the heart rate
variability signals data recorded during tilt-test study.
The vector of 64 time-domain, frequency-domain and
non-linear features was used. The results, obtained in
this work will become foundation of the decision
support system in treatment of arterial hypertension.
Results of this study showed, that application of
Artificial Neural Networks reaches higher
classification accuracy results than such machine
learning classifiers as discriminant analysis, Naïve
Bayes, Decision Tree, Nearest Neighbors. Relatively
high values of accuracy were obtained – 86.8. We
want to point out that this work was the first step in
the neural networks application study. In the
following we are interested in continuing more
complex networks that could perform prior features
selection for accuracy improvement. One of the
possible ways of the future development is usage of
different networks architectures, like auto-encoding,
for dimension reduction (Hinton and Salakhutdinov,
2006).
The next step in the development of the decision
support system in treatment of arterial hypertension is
evaluation of the treatment efficiency. We are
planning to evaluate efficiency of the standard
pharmacological therapy and neuro-
electrostimulation by the ‘SYMPATHOCOR-01’
device. Such study will allow to estimate possibility
of the proposed system application for evaluation of
the treatment efficiency and prognosis of the
treatment process.
ACKNOWLEDGEMENTS
The work was supported by Act 211 Government of
the Russian Federation, contract № 02.A03.21.0006.
REFERENCES
Baevskiy, R.M., 2001. Аnaliz variabelnosti serdechnogo
ritma pri ispolzovanii razlichnykh
ehlektrokardiograficheskikh sistem (metodicheskie
rekomendatsii) [Analysis of heart rate variability using
different electrocardiographic systems (guidelines)].
Vestn. Aritmologii Her. Arhythmology 65–87.
Belova, N.Y., Mihaylov, S.V., Piryova, B.G., 2007.
Wavelet transform: A better approach for the evaluation
of instantaneous changes in heart rate variability.
Auton. Neurosci. 131, 107–122.
Chattipakorn, N., Incharoen, T., Kanlop, N., Chattipakorn,
S., 2007. Heart rate variability in myocardial infarction
and heart failure. Int. J. Cardiol. 120, 289–296.
De Boor, C.A., 1978. Practical Guide to Splines. Springer-
Verlag.
Demuth, H.B., Beale, M.H., De Jess, O., Hagan, M.T.,
2014. Neural network design. Martin Hagan.
Egorova, D.D., Kazakov, Y.E., Kublanov, V.S., 2014.
Principal Components Method for Heart Rate
Variability Analysis. Biomed. Eng. 48, 37–41.
Feng, X.L., Pang, M., Beard, J., 2014. Health system
strengthening and hypertension awareness, treatment
and control: Data from the China health and retirement
longitudinal study. Bull. World Health Organ. 92, 29–
41.
Hinton, G.E., Salakhutdinov, R.R., 2006. Reducing the
dimensionality of data with neural networks. science
313, 504–507.
Hornik, K., Stinchcombe, M., White, H., 1989. Multilayer
feedforward networks are universal approximators.
Neural Netw. 2, 359–366.
Kleiger, R.E., Stein, P.K., Bigger, J.T., 2005. Heart rate
variability: measurement and clinical utility. Ann.
Noninvasive Electrocardiol. 10, 88–101.
Kseneva, S.I., Borodulina, E.V., Trifonova, O.Y., Udut,
V.V., 2016. Cardiac Autonomic Drive during Arterial
Hypertension and Metabolic Disturbances. Bull. Exp.
Biol. Med. 161, 237–240.
Kublanov, V.S., Dolganov, A.Y., Belo, D., Gamboa, H.,
2017. Comparison of Machine Learning Methods for
the Arterial Hypertension Diagnostics. Appl. Bionics
Biomech. 2017.
Kublanov, V.S., Shmirev, V.I., Shershever, A.S., Kazakov,
J.E., 2010. About Innovative Possibilities of Device
“SIMPATOCOR-01” in Management of Functional
Disorders of Vegetative and Central Nervous System in
Neurology, Kremljovskaya Medicine. Clin. Vestn. 4,
60–64.
Lee, C.K., Yoo, S.K., Park, Y., Kim, N., Jeong, K., Lee, B.,
2005. Using Neural Network to Recognize Human
Emotions from Heart Rate Variability and Skin
Resistance. In: 2005 IEEE Engineering in Medicine and
Biology 27th Annual Conference. Presented at the 2005
IEEE Engineering in Medicine and Biology 27th
Annual Conference, pp. 5523–5525.
Malik, M., 1996. Heart rate variability: Standards of
measurement, physiological interpretation, and clinical
use. Circulation 93, 1043–1065.
Mallat, S., 2009. A Wavelet Tour of Signal Processing, A
Wavelet Tour of Signal Processing.
Mancia, G., Fagard, R., Narkiewicz, K., Redon, J.,
Zanchetti, A., Böhm, M., Christiaens, T., Cifkova, R.,
Backer, G.D., Dominiczak, A., Galderisi, M., Grobbee,
D.E., Jaarsma, T., Kirchhof, P., Kjeldsen, S.E., Laurent,
S., Manolis, A.J., Nilsson, P.M., Ruilope, L.M.,
Schmieder, R.E., Sirnes, P.A., Sleight, P., Viigimaa,
NENT 2018 - Special Session on Neuro-electrostimulation in Neurorehabilitation Tasks
330