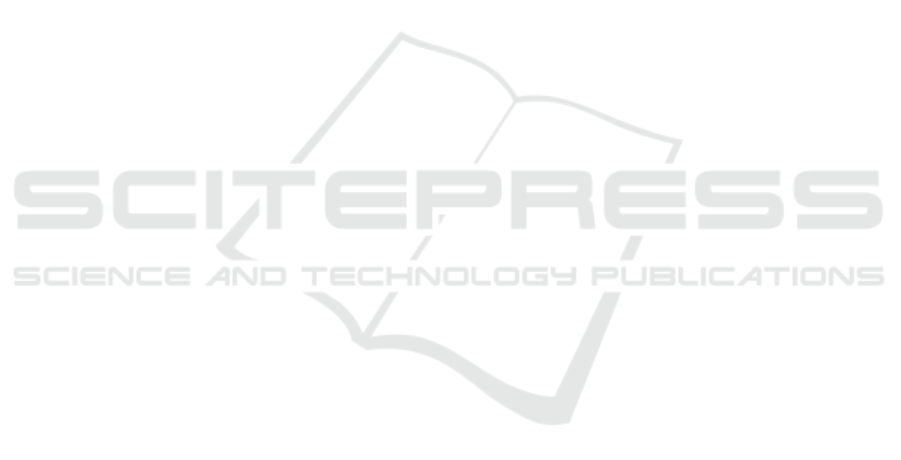
wearable healthcare will drastically improve in-home
care for a variety of patients. Collected data processed
using big-data techniques or advanced signal process-
ing could also have great predictive values for the
evolution of chronic diseases and could be used to
provide better and earlier care for patients.
However, the personal nature of collected health
data mandates strong security mechanism, which were
not explored in our contribution. Indeed, at the mo-
ment our system only features basic data encryption
in compliance with the BLE standard, and the MQTT-
based solution does not use any security mechanism.
Security of our overall system needs to be improved,
more particularly in terms of access control (i.e., the
patients of physicians must be able to know and con-
trol who accesses their medical data) and identity
management, and recently developed decentralized
blockchain-based solutions (Zhu et al., 2017) can be
explored in order to provide comprehensive werable
healthcare system security.
5 CONCLUSION
In this paper, we presented a multiparametric, car-
diorespiratory wearable sensor. In order to answer
healthcare requirements, more particularly in terms of
the ability to integrate wearable sensors into wider-
scale frameworks, we considered an IoT-based ap-
proach. Indeed, we equipped our sensor with remote
configuration capabilities while preserving quality-of-
data and real-time streaming capabilities, which are
key requirements of wearable healthcare systems. This
sensor was implemented using carefully selected hard-
ware, and it was comprehensively characterized in
terms of energy consumption, which is another major
concern of wearable healthcare devices. Indeed, be-
cause battery charging usually implies the sensor is
not collecting physiological data, potentially relevant
data can be lost, and the charging time to battery life
ration must thus be as big as possible. The data col-
lection capabilities of our sensor were also extensively
tested on both synthetic ECG signals and in real-life
ambulatory conditions. Successful testing and inte-
gration to Internet-based framework proved that our
sensor can be used in a wide-scale wearable healthcare
framework.
ACKNOWLEDGMENT
The authors would like to thank the COOPERA fund-
ing program of R
´
egion Auvergne Rh
ˆ
one-Alpes for
their generous financial support.
REFERENCES
Altini, M., Polito, S., Penders, J., Kim, H., Van Helleputte,
N., Kim, S., and Yazicioglu, F. (2011). An ECG patch
combining a customized ultra-low-power ECG SoC
with bluetooth low energy for long term ambulatory
monitoring. In Proceedings of the 2nd Conference
on Wireless Health, pages 15:1–15:2, New York, NY,
USA. ACM.
Fernandez, F. and Pallis, G. C. (2014). Opportunities and
challenges of the internet of things for healthcare: Sys-
tems engineering perspective. In Proceedings of the
EAI International Conference on Wireless Mobile Com-
munication and Healthcare, pages 263–266.
Gubbi, J., Buyya, R., Marusic, S., and Palaniswami, M.
(2013). Internet of things (IoT): A vision, architectural
elements, and future directions. Future Generation
Computer Systems, 29(7):1645 – 1660.
Harris III, A. F., Khanna, V., Tuncay, G., Want, R., and
Kravets, R. (2016). Bluetooth low energy in dense
IoT environments. IEEE Communications Magazine,
54(12):30–36.
Izumi, S., Yamashita, K., Nakano, M., Kawaguchi, H.,
Kimura, H., Marumoto, K., Fuchikami, T., Fujimori,
Y., Nakajima, H., Shiga, T., and Yoshimoto, M. (2015).
A wearable healthcare system with a 13.7
µ
A noise tol-
erant ecg processor. IEEE Transactions on Biomedical
Circuits and Systems, 9(5):733–742.
Khayatzadeh, M., Zhang, X., Tan, J., Liew, W. S., and Lian,
Y. (2013). A 0.7-V 17.4-
µ
W 3-lead wireless ECG
SoC. IEEE Transactions on Biomedical Circuits and
Systems, 7(5):583–592.
Lomb, N. R. (1976). Least-squares frequency analysis of
unequally spaced data. Astrophysics and Space Science,
39(2):447–462.
Magno, M., Spagnol, C., Benini, L., and Popovici, E.
(2014). A low power wireless node for contact and
contactless heart monitoring. Microelectronics Journal,
45(12):1656–1664.
Massot, B., Noury, N., Gehin, C., and McAdams, E. (2013).
On designing an ubiquitous sensor network for health
monitoring. In Proceedings of the International Con-
ference on e-Health Networking, Applications and Ser-
vices, pages 310–314.
Massot, B., Risset, T., Michelet, G., and McAdams, E.
(2015). A wireless, low-power, smart sensor of cardiac
activity for clinical remote monitoring. In Proceedings
of the International Conference on E-health Network-
ing, Application Services, pages 488–494.
Massot, B., Risset, T., Michelet, G., and McAdams, E.
(2016). Mixed hardware and software embedded sig-
nal processing methods for in-situ analysis of cardiac
activity. In Proceedings of the 9th International Joint
Conference on Biomedical Engineering Systems and
Technologies, pages 303–310.
Nunan, D., Sandercock, G. R., and Brodie, D. A. (2010).
A quantitative systematic review of normal values
for short-term heart rate variability in healthy adults.
Pacing and Clinical Electrophysiology, 33(11):1407–
1417.
Building IoT-Enabled Wearable Medical Devices: An Application to a Wearable, Multiparametric, Cardiorespiratory Sensor
117