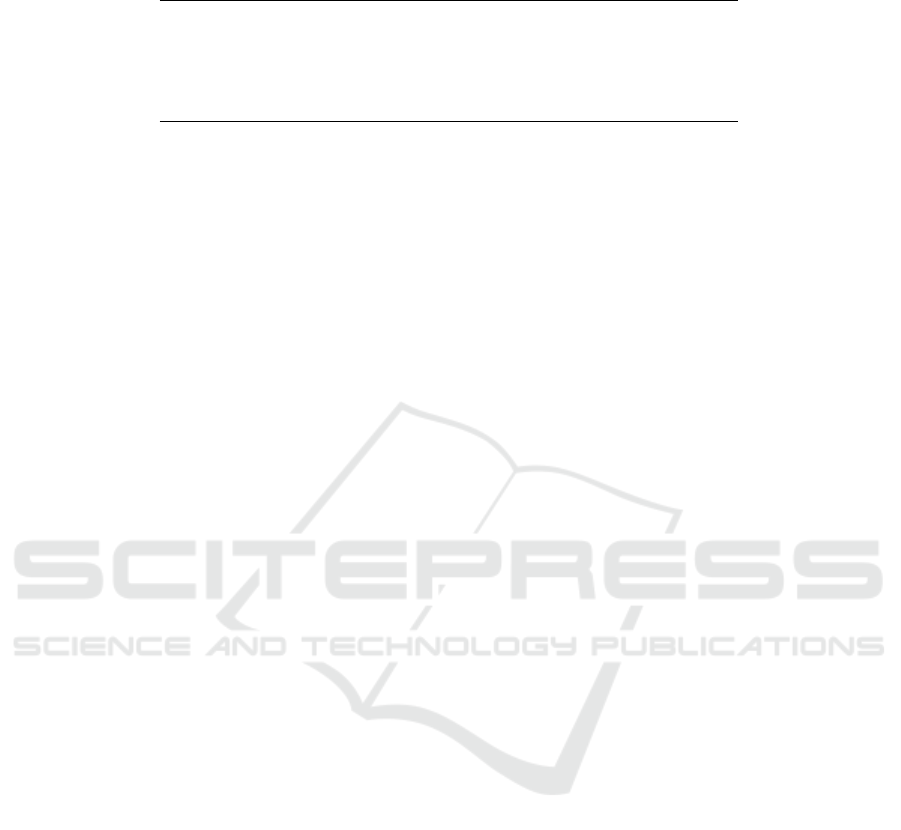
Table 4: The Percentage of Points Falling in the Different Zones of the Clarke Error Grid.
Zone GP Train GP Test IGBG Train IGBC Test S-R
opt
Train S-R
opt
Test
A 98.73% 95.28% 94.49% 90.57% 97.46% 93.40%
B 0.85% 4.72% 4.24% 8.49% 1.69% 6.60 %
A + B 99.58% 100% 98.73% 99.06% 99.15% 100%
C 0.00 % 0.00% 0,00% 0.0% 0% 0%
D 0.42% 0% 1.27% 0.94% 0.85% 0%
E 0.00% 0.00% 0.00% 0.00% 0.00% 0.00%
ues as if they were the exact measured values of
the BG, and against the state-of-the-art Steil-Rebrin
model with optimized parameters. The results have
shown its superiority in terms of lower RMSE, and
of better fitting in the Clarke Error Grid. The find-
ings obtained seem to imply that a unique model can
fit all of the subjects involved in this study, and the
artificial pancreas for all of them could be based on
evolutionary-devised model.
Future work implies the use of evolutionary-
devised model in a clinical trial to estimate the BG
values of the involved subjects, so as to further test its
effectiveness. In the positive case, this model could
be added to an under-development artificial pancreas
device for a real experimentation.
ACKNOWLEDGEMENTS
This publication was partially supported by the
project LO1506 of the Czech Ministry of Education,
Youth and Sports.
REFERENCES
Boyne, M., Silver, D. M., Kaplan, J., and Saudek, C. D.
(2003). Timing of changes in interstitial and venous
blood glucose measured with a continuous subcuta-
neous glucose sensor. Diabetes, 52:2790–2794.
Clarke, W. L., Cox, D., Gonder-Frederick, L., Carter, W.,
and Pohl, S. (1987). Evaluating clinical accuracy of
systems for self-monitoring of blood glucose. Dia-
betes Care, 10:622–628.
De Falco, I., Della Cioppa, A., Scafuri, U., and Tarantino,
E. (2017). Accurate estimate of blood glucose through
interstitial glucose by genetic programming. In Pro-
ceedings of the International Symposium on Comput-
ers and Communications, pages 284–289. IEEE Press.
De Falco, I., Della Cioppa, A., Tarantino, E., and
Fontanella, F. (2005). A novel grammar-based genetic
programming approach to clustering. In Proceedings
of the ACM Symposium on Applied Computing, vol-
ume 2, pages 928–932. ACM Computing.
De Falco, I., Della Cioppa, A., Tarantino, E., and
Fontanella, F. (2006). An innovative approach to ge-
netic programming-based clustering, volume 34 of
Advances in Soft Computing, pages 55–64. Springer.
Del Favero, S., Facchinetti, A., Sparacino, G., and Co-
belli, C. (2014). Improving accuracy and precision of
glucose sensor profiles: retrospective fitting by con-
strained deconvolution. IEEE Trans. Biomed. Eng.,
61(4):1044–1053.
Koutny, T. (2014). Blood glucose level reconstruction as a
function of transcapillary glucose transport. Comput.
Biol. Med., 53:171–178.
Koutny, T. (2016). Using meta-differential evolution to
enhance a calculation of a continuous blood glu-
cose level. Computer methods and programs in
biomedicine, 133:45–54.
Koza, J. (1992). Genetic programming: on the program-
ming of computers by means of natural selection, vol-
ume 1. MIT Press.
Makroglou, A., Li, J., and Kuang, Y. (2006). Mathemati-
cal models and software tools for the glucose-insulin
regulatory system and diabetes: an overview. Applied
Numerical Mathematics, 56:559–573.
P
´
erez-Gand
´
ıa, C., Facchinetti, A., Sparacino, G., Cobelli,
C., G
´
omez, E., Rigla, M., de Lieiva, A., and Her-
nando, M. E. (2010). Artificial neural network al-
gorithm for online glucose prediction from contin-
uous glucose monitoring. Diabetes Technol. Ther.,
12(1):81–88.
Price, K. and Storn, R. (1997). Differential evolution. Dr.
Dobb’s Journal, 22(4):18–24.
Rebrin, K., Steil, G., van Antwerp, W., and Mastrototaro,
J. (1999). Subcutaneous glucose predicts plasma glu-
cose independent of insulin: implications for continu-
ous monitoring. Am. J. Physiol. 277, 277:E561–E571.
Rossetti, P., Bondia, J., Veh
´
ı, J., and Fanelli, C. (2010). Es-
timating plasma glucose from interstitial glucose: the
issue of calibration algorithms in commercial contin-
uous glucose monitoring devices. Sensors, 10:10936–
10952.
Searson, D. (2009). GPTIPS: Genetic program-
ming and symbolic regression for MATLAB.
http://gptips.sourceforge.net., 2009.
Steil, G., Bernaba, B., Saad, M., Mastrototaro, J., and Re-
brin, K. (2000). Accurate determination of plasma
glucose during hyper- and hypoglycemia with a sub-
cutaneous glucose sensor. Diabetes, 49 (Suppl.
1):A126.
Vashist, S. K. (2013). Continuous glucose monitoring sys-
tems: a review. Diagnostics, 3(4):385–412.
World Health Organization (2013). Diabetes fact sheet n.
312.