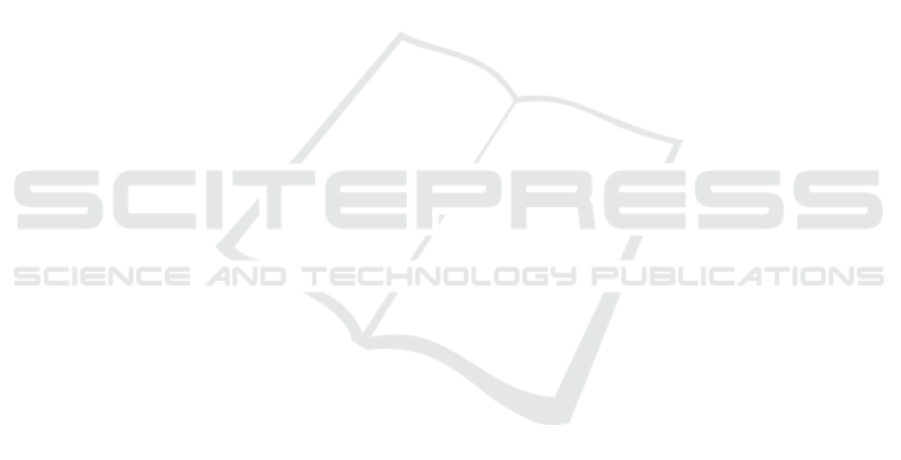
nal features. In IEEE Int. Conf.on Electro/Information
Technology (EIT), pages 1–6.
Alqassim, S., Ganesh, M., Khoja, S., Zaidi, M., Aloul, F.,
and Sagahyroon, A. (2012). Sleep apnea monitor-
ing using mobile phones. In IEEE 14th Int. Conf.
on e-Health Networking, Applications and Services
(Healthcom), pages 443–446.
Angeline, P. J., Saunders, G. M., and Pollack, J. B. (1994).
An evolutionary algorithm that constructs recurrent
neural networks. IEEE transactions on Neural Net-
works, 5(1):54–65.
B
¨
ack, T., Fogel, D., and Michalewicz, Z. (1997). Handbook
of evolutionary computation. Release, 97(1):B1.
Cuomo, S., De Pietro, G., Farina, R., Galletti, A., and San-
nino, G. (2016). A revised scheme for real time ecg
signal denoising based on recursive filtering. Biomed-
ical Signal Processing and Control, 27:134–144.
De Falco, I., De Pietro, G., and Sannino, G. (2015). On find-
ing explicit rules for personalized forecasting of ob-
structive sleep apnea episodes. In IEEE Int. Conf. on
Information Reuse and Integration (IRI), pages 326–
333.
De Falco, I., Della Cioppa, A., Maisto, D., Scafuri, U.,
and Tarantino, E. (2014). An adaptive invasion-based
model for distributed differential evolution. Informa-
tion Sciences, 278:653–672.
De Falco, I., Della Cioppa, A., Scafuri, U., and Tarantino,
E. (2017). Exploiting diversity in an asynchronous
migration model for distributed differential evolution.
In Genetic and Evolutionary Computation Conf. Com-
panion (GECCO), pages 1880–1887.
De Falco, I., Iazzetta, A., Natale, P., and Tarantino, E.
(1998). Evolutionary neural networks for nonlinear
dynamics modeling. In Parallel Problem Solving from
Nature (PPSN), pages 593–602.
Edlund, J. A., Chaumont, N., Hintze, A., Koch, C., Tononi,
G., and Adami, C. (2011). Integrated information in-
creases with fitness in the evolution of animats. PLoS
computational biology, 7(10):e1002236.
Gruau, F. et al. (1994). Neural network synthesis using cel-
lular encoding and the genetic algorithm.
Hertz, J. A., Krogh, A. S., and Palmer, R. G. (1991). Intro-
duction to the theory of neural computation, volume 1.
Kassahun, Y. and Sommer, G. (2005). Efficient rein-
forcement learning through evolutionary acquisition
of neural topologies. In ESANN, pages 259–266.
LeCun, Y., Bengio, Y., and Hinton, G. (2015). Deep learn-
ing. Nature, 521(7553):436–444.
McNicholas, W. and Levy, P. (2000). Sleep-related breath-
ing disorders: definitions and measurements.
Miikkulainen, R., Liang, J., Meyerson, E., Rawal, A., Fink,
D., Francon, O., Raju, B., Navruzyan, A., Duffy, N.,
and Hodjat, B. (2017). Evolving deep neural net-
works. arXiv.
Najafabadi, M. M., Villanustre, F., Khoshgoftaar, T. M.,
Seliya, N., Wald, R., and Muharemagic, E. (2015).
Deep learning applications and challenges in big data
analytics. Journal of Big Data, 2(1):1.
Niskanen, J.-P., Tarvainen, M. P., Ranta-Aho, P. O., and
Karjalainen, P. A. (2004). Software for advanced
hrv analysis. Computer methods and programs in
biomedicine, 76(1):73–81.
of the European Society of Cardiology, T. F. et al. (1996).
Heart rate variability: standards of measurement,
physiological interpretation, and clinical use. Circu-
lation, 93:1043–1065.
Penzel, T., Moody, G. B., Mark, R. G., Goldberger, A. L.,
and Peter, J. H. (2000). The apnea-ecg database. In
Computers in cardiology 2000, pages 255–258.
Price, K., Storn, R. M., and Lampinen, J. A. (2006). Differ-
ential evolution: a practical approach to global opti-
mization.
Real, E., Moore, S., Selle, A., Saxena, S., Suematsu, Y. L.,
Le, Q., and Kurakin, A. (2017). Large-scale evolution
of image classifiers. arXiv.
Ronald, E. and Schoenauer, M. (1994). Genetic lander: An
experiment in accurate neuro-genetic control. In Int.
Conf. on Parallel Problem Solving from Nature, pages
452–461.
Rumelhart, D. E., Hinton, G. E., and Williams, R. J. (1985).
Learning internal representations by error propaga-
tion. Technical report, California Univ San Diego La
Jolla Inst for Cognitive Science.
Sannino, G., De Falco, I., and De Pietro, G. (2014). An
automatic rules extraction approach to support osa
events detection in an mhealth system. IEEE jour-
nal of biomedical and health informatics, 18(5):1518–
1524.
Sher, G. I. (2012). Handbook of neuroevolution through
Erlang.
Shokoueinejad, M., Fernandez, C., Carroll, E., Wang, F.,
Levin, J., Rusk, S., Glattard, N., Mulchrone, A.,
Zhang, X., Xie, A., et al. (2017). Sleep apnea: a re-
view of diagnostic sensors, algorithms, and therapies.
Physiological measurement, 38(9):R204.
Siebel, N. T. and Sommer, G. (2007). Evolutionary re-
inforcement learning of artificial neural networks.
International Journal of Hybrid Intelligent Systems,
4(3):171–183.
Stanley, K. O. and Miikkulainen, R. (2002). Evolving neu-
ral networks through augmenting topologies. Evolu-
tionary computation, 10(2):99–127.
Tomassini, M. (2006). Spatially structured evolutionary al-
gorithms: artificial evolution in space and time.
Vargas, D. V. and Murata, J. (2017). Spectrum-diverse
neuroevolution with unified neural models. IEEE
transactions on neural networks and learning systems,
28(8):1759–1773.
Yao, X. and Liu, Y. (1997). A new evolutionary system
for evolving artificial neural networks. IEEE trans. on
neural networks, 8(3):694–713.