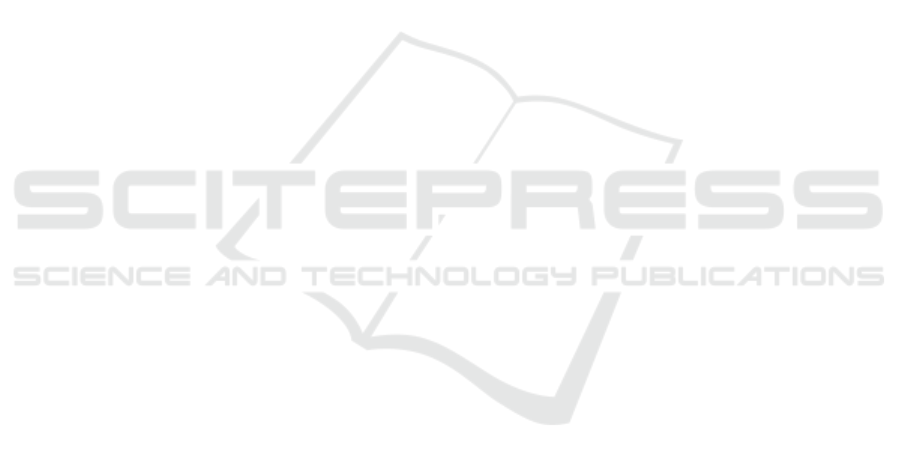
sifying the presence of carotid diseases using HRV
features. HRV analysis represents one of the main
approach able to estimate functionality of cardiovas-
cular system, in relationship with carotid diseases
as demonstrated by several studies existing in liter-
ature. We have considered several machine learning
methods including Support Vector Machine, Bayesian
Classifiers, Decision Tree, Multilayer Perceptron, Lo-
gistic Model Tree and Instance-based Learning algo-
rithms. The Support Vector Machine has proved to be
more effective than any of the other methods consid-
ered.
In future work, we will explore the possibility of
improving the classification obtained by using a hy-
brid system constructed by combining several ma-
chine learning techniques and methods for data reduc-
tion, such as for example the Principles Component
Analysis (PCA), necessary to reduce computational
complexity and execution times.
ACKNOWLEDGEMENTS
This work has received funding from the European
Unions Horizon 2020 Framework Programme for
Research and Innovation under grant agreement no
727528 (KONFIDO). The authors would like to ac-
knowledge Prof. Luigi Romano, of the Department
of Technology, University of Naples Parthenope for
his technical contribution.
REFERENCES
Aha, D. W., Kibler, D., and Albert, M. K. (1991).
Instance-based learning algorithms. Machine learn-
ing, 6(1):37–66.
Burges, C. J. (1998). A tutorial on support vector machines
for pattern recognition. Data mining and knowledge
discovery, 2(2):121–167.
Camm, A. J., Malik, M., Bigger, J., Breithardt, G., Cerutti,
S., Cohen, R. J., Coumel, P., Fallen, E. L., Kennedy,
H. L., Kleiger, R. E., et al. (1996). Heart rate vari-
ability: standards of measurement, physiological in-
terpretation and clinical use. task force of the euro-
pean society of cardiology and the north american so-
ciety of pacing and electrophysiology. Circulation,
93(5):1043–1065.
Chao, A., Chern, C., Kuo, T., Chou, C., Chuang, Y., Wong,
W., and Hu, H. (2003). Noninvasive assessment of
spontaneous baroreflex sensitivity and heart rate vari-
ability in patients with carotid stenosis. Cerebrovas-
cular Diseases, 16(2):151–157.
Cleary, J. G., Trigg, L. E., et al. (1995). K*: An instance-
based learner using an entropic distance measure. In
Proceedings of the 12th International Conference on
Machine learning, volume 5, pages 108–114.
Dirgenali, F. and Kara, S. (2006). Recognition of early
phase of atherosclerosis using principles component
analysis and artificial neural networks from carotid
artery doppler signals. Expert Systems with Applica-
tions, 31(3):643–651.
Fakhrzadeh, H., Yamini-Sharif, A., Sharifi, F., Tajal-
izadekhoob, Y., Mirarefin, M., Mohammadzadeh, M.,
Sadeghian, S., Badamchizadeh, Z., and Larijani, B.
(2012). Cardiac autonomic neuropathy measured
by heart rate variability and markers of subclinical
atherosclerosis in early type 2 diabetes. ISRN en-
docrinology, 2012.
Goldberger, A., Amaral, L., Glass, L., Hausdorff, J., Ivanov,
P. C., Mark, R., Mietus, J., Moody, G., Peng, C., and
Stanley, H. (2000). Physiobank, physiotoolkit, and
physionet: Components of a new research resource for
complex physiologic signals. circulation [online]. 101
(23), pp. e215–e220.
Gotts
¨
ater, A., Ahlgren,
˚
A. R., Taimour, S., and Sundkvist,
G. (2006). Decreased heart rate variability may pre-
dict the progression of carotid atherosclerosis in type
2 diabetes. Clinical Autonomic Research, 16(3):228–
234.
Grant, E. G., Benson, C. B., Moneta, G. L., Alexandrov,
A. V., Baker, J. D., Bluth, E. I., Carroll, B. A.,
Eliasziw, M., Gocke, J., Hertzberg, B. S., et al. (2003).
Carotid artery stenosis: gray-scale and doppler us di-
agnosissociety of radiologists in ultrasound consensus
conference. Radiology, 229(2):340–346.
H
¨
ogberg, D., Dellagrammaticas, D., Kragsterman, B.,
Bj
¨
orck, M., and Wanhainen, A. (2016). Simplified ul-
trasound protocol for the exclusion of clinically signif-
icant carotid artery stenosis. Upsala journal of medi-
cal sciences, 121(3):165–169.
Huikuri, H. V., M
¨
akikallio, T., Airaksinen, K. J., Mitrani,
R., Castellanos, A., and Myerburg, R. J. (1999). Mea-
surement of heart rate variability: a clinical tool or
a research toy? Journal of the American College of
Cardiology, 34(7):1878–1883.
John, G. H. and Langley, P. (1995). Estimating continuous
distributions in bayesian classifiers. In Proceedings
of the Eleventh conference on Uncertainty in artificial
intelligence, pages 338–345. Morgan Kaufmann Pub-
lishers Inc.
Kaufman, C. L., Kaiser, D. R., Steinberger, J., and Dengel,
D. R. (2007). Relationships between heart rate vari-
ability, vascular function, and adiposity in children.
Clinical Autonomic Research, 17(3):165–171.
Kim, H., Ishag, M. I. M., Piao, M., Kwon, T., and Ryu,
K. H. (2016). A data mining approach for cardiovas-
cular disease diagnosis using heart rate variability and
images of carotid arteries. Symmetry, 8(6):47.
Kim, W.-S., Jin, S.-H., Park, Y., and Choi, H.-M. (2007).
A study on development of multi-parametric mea-
sure of heart rate variability diagnosing cardiovascu-
lar disease. In World Congress on Medical Physics
and Biomedical Engineering 2006, pages 3480–3483.
Springer.