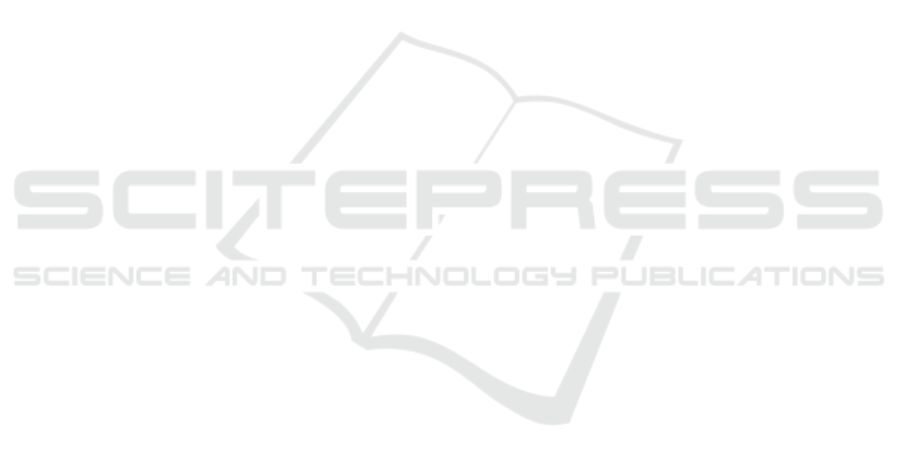
We used a database containing sound samples
that were recorded from a mobile phone or a laptop
computer. These samples contain the same common
20-words sentence in Portuguese. For the severity of
ALS disease, a short sentence is an important
requisite of our methodology, to make it valid for
these patients’ context. In fact, as speech becomes
difficult to produce, the more complex is the sample
collection, and the more dropouts will take place.
The results described in previous sections
demonstrate that the KLD from a healthy control is
sensitive to neurodegeneration progression in ALS.
For all ALS subjects, except one, the AKV model
expressed progression of neurodegenerative
symptoms in speech, by increase of KLD, for both
the younger and the older models. The exception
was observed for the youngest subject with ALS (38
years old). In fact, we can hypothesize that the
samples from this patient will fit better to the
younger control model, due to the age proximities.
The LLR distance for the younger model expresses a
continuous increase and correlation to the ALSFRS-
B values attributed to this subject.
In general, the results described in this work
confirm the hypothesis that we can model the speech
dysarthria in ALS as a “freezing” of the articulation
process: the probability of AKV close to 0 increases
as diseases progresses.
Our objective and quantitative measures are
according to the qualitatively assessed clinical rating
for bulbar involvement that is based on clinical
experienced observation. The apparent regression in
neurodegeneration from our results can be
confirmed by the experienced clinical observation of
stabilization of symptoms within assessments. But,
our quantitative measure may have implicit
information that is not observable and needs further
insight on its meaning. By hypothesis, our measures
may be sensible to different therapies that cause
variations observed within assessments. A new
dataset of samples that are collected in shorter time
intervals, from home mobile devices, is needed to
obtain a continuous observation of articulation
measures. A continuous observation will support a
novel insight on progression behaviour.
Our study has some limitations. One is the
heterogeneity of our sample, since some subjects
have 2 and others have 5 assessments. For this
reason, it is not possible to have a solid
demonstration of the progression along time.
Another limitation is that we are using samples
containing the same phonetic material. In all
samples, subjects use the same sentence.
For future work, a larger database containing
spontaneous speech from subjects with ALS will be
used to further test our model and study progression
from symptoms measured in speech signal.
ACKNOWLEDGEMENTS
This work was supported by Calouste Gulbenkian
Foundation and the Portuguese Association of ALS
(APELA), as well as by grant TEC2016-77791-C4-
4-R (Plan Nacional de I+D+i, Ministry of Economic
Affairs and Competitiveness of Spain).
REFERENCES
Abad, A. et al., 2013. Automatic word naming recognition
for an on-line aphasia treatment system. Computer
Speech and Language, 27(6), pp.1235–1248.
Andersen, P.M. et al., 2012. EFNS guidelines on the
Clinical Management of Amyotrophic Lateral
Sclerosis (MALS) - revised report of an EFNS task
force. European Journal of Neurology, 19(3), pp.360–
375.
de Carvalho, M., Costa, J. & Swash, M., 2005. Clinical
trials in ALS: a review of the role of clinical and
neurophysiological measurements. Amyotrophic
Lateral Sclerosis & Other Motor Neuron Disorders,
6(4), pp.202–212. Available at:
http://search.ebscohost.com/login.aspx?direct=true&d
b=a2h&AN=19020138&loginpage=Login.asp&site=e
host-live.
Cedarbaum, J.M. & Stambler, N., 1997. Performance of
the amyotrophic lateral sclerosis functional rating
scale (ALSFRS) in multicenter clinical trials. In
Journal of the Neurological Sciences.
Deller, J.R., Proakis, J.G. & Hansen, J.H.L., 2000.
Discrete-Time Processing of Speech Signals,
Available at:
http://www.library.wisc.edu/selectedtocs/bd429.pdf
Gómez-vilda, P. et al., 2013. Characterization of Speech
from Amyotrophic Lateral Sclerosis by Neuromorphic
Processing. In IWINAC 2013. pp. 212–224.
Gómez-Vilda, P. et al., 2015. Monitoring amyotrophic
lateral sclerosis by biomechanical modeling of speech
production. Neurocomputing, 151(1), pp.130–138.
Londral, A. et al., 2015. Quality of life in ALS patients
and caregivers: impact of assistive communication
from early stages. Muscle and Nerve.
P.Gomez, P. et al., 2017. Articulation acoustic kinematics
in ALS speech. In 2017 International Conference and
Workshop on Bioinspired Intelligence (IWOBI). IEEE,
pp. 1–6. Available at:
http://ieeexplore.ieee.org/document/7985522/.
Sapir, S. et al., 2011. Acoustic metrics of vowel
articulation in Parkinson’s Disease: Vowel Space Area
(VSA) vs. vowel articulation index (VAI). In C.
Manfredi, ed. Proceedings of the MAVEBA 2011.
Florence: Florence University Press, pp. 173–175.
Tomik, B. & Guiloff, R.J., 2010. Dysarthria in
amyotrophic lateral sclerosis: A review. Amyotrophic
Lateral Sclerosis, 11(1–2), pp.4–15.
BIOSIGNALS 2018 - 11th International Conference on Bio-inspired Systems and Signal Processing
274