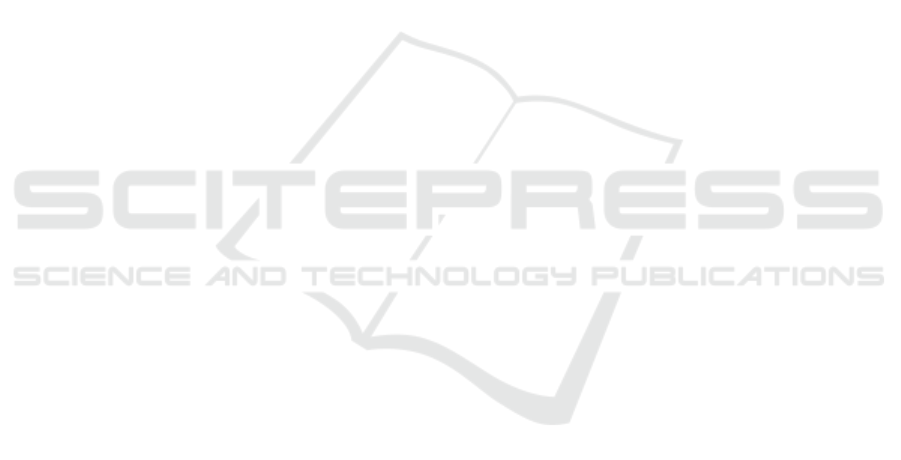
4 DISCUSSION
The ability of a UAV crew to conduct effective
operations is based to a large extent on their training.
The role of the SO is critical in determining the
success of a mission and rapid decision making must
often be made in a timely manner, and sometimes
calls upon greater demand of attentional resources.
This is particularly true of missions that require both
rapid response to a changing environment, and also
missions where vigilance may result in mental fatigue
(and increased likelihood of human error). It is
essential therefore that training regimes for operators
takes these factors into account and in many cases this
will often rely upon high fidelity simulation and
scenario-based mission tasks. However, the
evaluation techniques currently being used to assess
such training tends to focus on behaviour markers
related to task, rather than the cognitive ability of the
operator. This study builds on the knowledge that we
have already gathered by using other functional
neurological imaging techniques (such as MRI, EEG)
and harnessed the utilisation of fNIRS within a field-
based study to demonstrate the benefits this form of
measurement may have when assessing operator
cognitive state. Further, there are vast amounts of
research reported fNIRS studies in the aerospace
domain. The training effect and expertise
development for manned aircraft pilots was studied
with the fNIRS and reported oxygenation decrease on
PFC (Hernandez, et al., 2015). Cognitive workload of
air traffic controllers was measured by using fNIRS
and explored assessment of working memory from
the PFC (Ayaz, et al., 2012). Further, we studied
UAV pilots on landing and approach task while
measuring the expertise development via fNIRS
(Izzetoglu, et al., 2014; Ayaz, et al., 2012).
The current study identified that as participants
acquired knowledge and gained new skills we are
able to observe how they draw on oxygenation to
increase their cognitive effort associated with
different elements localised within the PFC. On
closer inspection this localised oxygenation change is
associated with parts of the PFC closely aligned with
the middle frontal gyrus of the right hemisphere
associated with attention.
The experimental protocol reported here was very
complex task. Although these results, behavioural
and neuro-physiological measures, are in line with
previous reports, one should conduct further analysis
for all the sub-tasks for the payload operators and
investigate fNIRS measures acquired from all the
PFC regions for each sub-task and overall task
performance.
This study has demonstrated the benefit of
utilising fNIRS as a biomarker for cognitive function
of participants employed in conducting UAV sensor
operator tasks. While it has highlighted the nature of
cognitive function within the PFC, it also can be used
as an evaluation of expertise during multiple training
sessions.
ACKNOWLEDGEMENTS
The authors express their gratitude to Shahar Kosti
for his valuable review and support for scenario
design, UAV simulator setup and behavioral data.
The authors also would like to thank Simlat, Inc for
providing access to their UAV training simulator.
REFERENCES
Ayaz H, Shewokis PA, Bunce S, Izzetoglu K, Willems B,
Onaral B. (2012). Optical brain monitoring for operator
training and mental workload assessment. Neuroimage,
59(1):36-47. PMID: 21722738.
Cooke, N. J., & Shope, S. M. (2004). Designing a synthetic
task environment. In Schiflett, S. G.
Cope, M., and Delpy, D. T. (1988). System for long-term
measurement of cerebral blood flow and tissue
oxygenation on newborn infants by infra-red
transillumination. Med. Biol. Eng. Comput., 26: 289–
294.
Hernandez-Meza, G., Slason, L., Ayaz, H., Craven, P.,
Oden, K., & Izzetoglu, K. (2015). Investigation of
Functional Near Infrared Spectroscopy in Evaluation
of Pilot Expertise Acquisition. In D. D. Schmorrow &
C. M. Fidopiastis (Eds.), Foundations of Augmented
Cognition (Vol. 9183, pp. 232-243): Springer
International Publishing.
Izzetoglu, K., Bunce, S., Onaral, B., Pourrezaei, K.,
Chance, B (2004) Functional optical brain imaging
using near-infrared during cognitive tasks.
International Journal of Human-Computer Interaction
17, 211-227 (2004)
Izzetoglu, K., Ayaz, H., Hing, J., Shewokis, P., Bunce, S.,
Oh, P., & Onaral, B. (2014). UAV Operators Workload
Assessment by Optical Brain Imaging Technology
(fNIR). In K. P. Valavanis & G. J. Vachtsevanos (Eds.),
Handbook of Unmanned Aerial Vehicles (pp. 2475-
2500): Springer Netherlands
Izzetoglu M, Bunce S, Izzetoglu K, Onaral B, Pourrezaei K
(2007). Functional Brain Imaging Using Near Infrared
Technology for Augmented Cognition. IEEE Eng. in
Med. and Biol. Mag., Special issue on The Role of
Optical Imaging in Augmented Cognition, 26(4):38 –
46.
Real 2018 - Special Session on Assessing Human Cognitive State in Real-World Environments
292