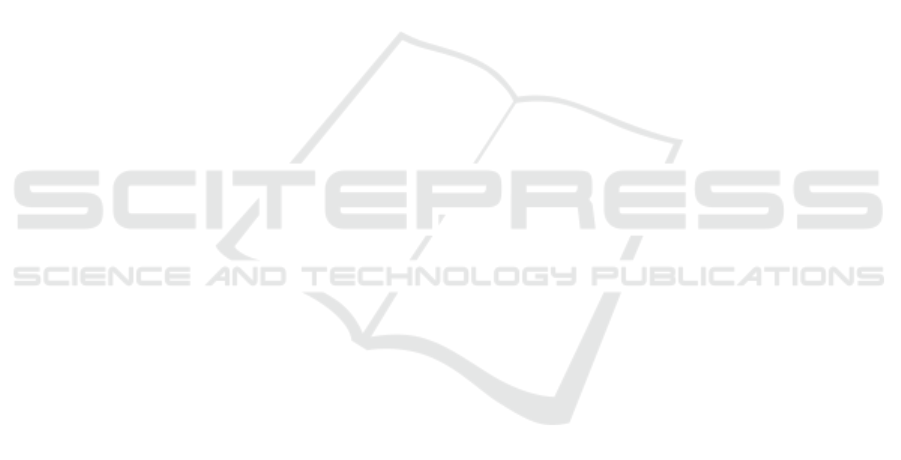
susci Mannequin (acting as the gold standard). This
is superior to the shoulder features with 4.9%, which
probably suffer from small dampening effects of the
elbow joint. The hand features are often occluded
even under near-optimal view situations and show a
higher variance of 7.7% variance. We will investigate
if the combined consideration of all three limb regions
will enhance the sensitivity furthermore.
While the results determining the CPR frequency
are already promising, the results for the compression
depth are not yet conclusive. The discrepancy to the
Resusci Anne results ranged between 5-39mm (15-
115%) with the hands features working best (mean
16mm). The high variance between the results made
it impossible to find a stable constant offset or factor
that may improve the accuracy. Here, more investi-
gations are needed, to determine why only singular
results are so far promising (see Figure 4).
Thus, our work represents an initial step towards a
more complete and precise modeling of the ERC CPR
using ambient sensors.
ACKNOWLEDGEMENTS
This work was supported by the funding initiative
Nieders
¨
achsisches Vorab of the Volkswagen Founda-
tion and the Ministry of Science and Culture of Lo-
wer Saxony as a part of the Interdisciplinary Research
Centre on Critical Systems Engineering for Socio-
Technical Systems II.
The authors would like to thank the anonymous
reviewers for their helpful comments.
REFERENCES
Higashi, E., Fukagawa, K., Kasimura, R., Kanamori, Y.,
Minazuki, A., and Hayashi, H. (2017). Development
and evaluation of a corrective feedback system using
augmented reality for the high-quality cardiopulmo-
nary resuscitation training.
Loconsole, C., Frisoli, A., Semeraro, F., Stroppa, F., Ma-
stronicola, N., Filippeschi, A., and Marchetti, L.
(2016). Relive: a markerless assistant for cpr trai-
ning. IEEE Transactions on Human-Machine Sys-
tems, 46(5):755–760.
Perkins, G., Handley, A., Koster, R., Castren, M., Smyth,
M., Olasveengen, T., Monsieurs, K., Raffay, V., Gras-
ner, J., Wenzel, V., et al. (2015). European resuscita-
tion council guidelines for resuscitation 2015. Resus-
citation, 95:81–99.
Schmidt, R. F., Lang, F., and Heckmann, F. (2011). Physio-
logie des Menschen. Springer Verlag.
Semeraro, F., Frisoli, A., Loconsole, C., Bann
`
o, F., Tam-
maro, G., Imbriaco, G., Marchetti, L., and Cerchiari,
E. L. (2013). Motion detection technology as a tool
for cardiopulmonary resuscitation (CPR) quality trai-
ning: A randomised crossover mannequin pilot study.
Resuscitation, 84(4):501–507.
Semeraro, F., Frisoli, A., Loconsole, C., Mastronicola, N.,
Stroppa, F., Ristagno, G., Scapigliati, A., Marchetti,
L., and Cerchiari, E. (2017). Kids (learn how to) save
lives in the school with the serious game Relive. Re-
suscitation, 116:27–32.
Soar, J., Nolan, J. P., B
¨
ottiger, B. W., Perkins, G. D., Lott,
C., Carli, P., Pellis, T., Sandroni, C., Skrifvars, M. B.,
Smith, G. B., et al. (2015). European resuscitation
council guidelines for resuscitation 2015. Resuscita-
tion, 95:100–147.
Storn, R. and Price, K. (1997). Differential Evolution A
Simple and Efficient Heuristic for global Optimization
over Continuous Spaces. Journal of Global Optimiza-
tion, 11(4):341–359.
Tian, Y., Raghuraman, S., Yang, Y., Guo, X., and Prabha-
karan, B. (2014). 3d immersive cardiopulmonary re-
suscitation (cpr) trainer. In Proceedings of the 22nd
ACM international conference on Multimedia, pages
749–750. ACM.
Wang, J.-C., Tsai, S.-H., Chen, Y.-H., Chen, Y.-L., Chu,
S.-J., and Liao, W.-I. (2017). Kinect-based real-time
audiovisual feedback device improves cardiopulmo-
nary resuscitation quality of lower-body-weight res-
cuers. The American Journal of Emergency Medicine.