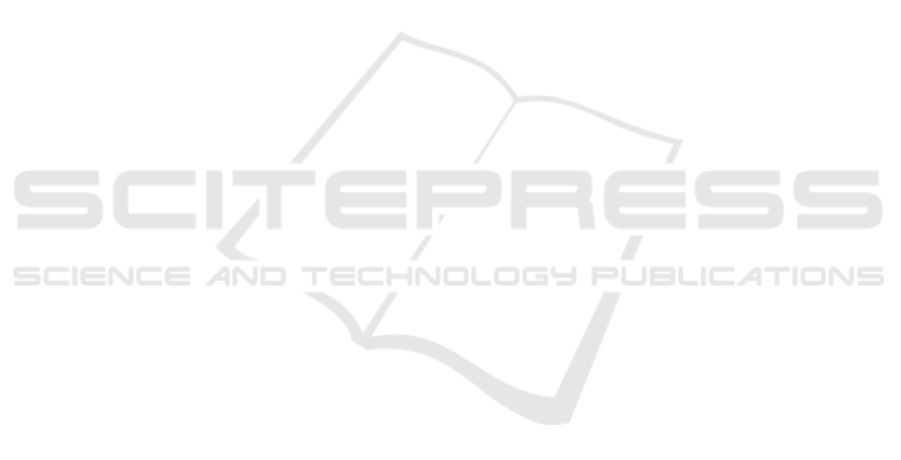
high as compared to resting, and it also stayed high
in whole stress session, shown in figure 3 and 4.
5 CONCLUSIONS AND FUTURE
PERSPECTIVE
User’s psychophysiological state was measured and
predicted in real time and autonomy is provided to
the system to improve its interface dynamically with
respect to the mental workload and stress level on
the user. A PAUI was created, that performs
dynamic updations to its interface and helps in
decelerating the effects of workload and stress.
Moreover, the classification findings are quite
impressive. We have explored different aspects of
psychophysiology and combined them with external
emotional and attentional clues. Getting 88.37% of
accuracy in Person specific data and 84.75%
accuracy in Task specific data with this small
amount of training samples gives a valid indication
of having huge potential of improvement.
Current findings clearly suggest that the use of
Deep learning techniques could be a promising
measure to achieve higher degree of accuracy in
emotion classification.
Future aspects of this research are with the
improvements in emotion classification techniques
with current state of the art classifiers. One
important field to scrutinize is with Recurrent Neural
Networks that could be helpful in understanding the
changing patterns of the data and make prediction on
them. And to introduce more emotional states for
classification which helps in bring more dynamicity
and understandability to PAUI.
ACKNOWLEDGEMENTS
This work was supported from Fundação para a
Ciência e a Tecnologia (FCT, Portugal), through
project UID/EEA/50009/2013.
REFERENCES
Affectiva. 2017. SDK & API. [online] Available at:
https://www.affectiva.com/product/emotion-sdk/
[Accessed 7 Nov. 2017].
Bitalino, 2017. EEG Sensor | Biomedical Sensors |
BITalino Board Kit. [online] Bitalino.com. Available
at: http://bitalino.com/en/plugged-kit-bt [Accessed 7
Nov. 2017].
Bulling, A., 2016. Pervasive Attentive User
Interfaces. IEEE Computer, 49(1), pp.94-98.
Caproni, M., Orihuela-Espina, F., James, D.R., Menciassi,
A., Dario, P., Darzi, A.W. and Yang, G.Z., 2009,
October. An analysis framework for near infrared
spectroscopy based brain-computer interface and
prospective application to robotic surgery.
In Intelligent Robots and Systems, 2009. IROS 2009.
IEEE/RSJ International Conference on(pp. 2143-
2148). IEEE.
Chapin, J.K., Moxon, K.A., Markowitz, R.S. and
Nicolelis, M.A., 1999. Real-time control of a robot
arm using simultaneously recorded neurons in the
motor cortex. Nature neuroscience, 2(7), pp.664-670.
Chen, D., 2006. The Physiologically Attentive User
Interface Towards A Physiological Model of
Interruptability (pp. 2836-2836). Queen's University.
Chen, D. and Vertegaal, R., 2004, April. Using mental
load for managing interruptions in physiologically
attentive user interfaces. In CHI'04 extended abstracts
on Human factors in computing systems (pp. 1513-
1516). ACM.
Dirican, A.C. and Gokturk, M., 2009. Attentive
computing. In Human-Computer Interaction. InTech.
Dirican, A.C. and Göktürk, M., 2011. Psychophysiological
measures of human cognitive states applied in human
computer interaction. Procedia Computer Science, 3,
pp.1361-1367.
McMahan, T., Parberry, I. and Parsons, T.D., 2015.
Evaluating player task engagement and arousal using
electroencephalography. Procedia Manufacturing, 3,
pp.2303-2310.
Němcová, A., Maršánová, L. and Smíšek, R., 2016
RECOMMENDATIONS FOR ECG ACQUISITION
USING BITALINO.
Rigsofrods.org. 2017. Rigs of Rods Soft Body Physics
Simulator. [online] Available at: https://rigsofrods.org/
[Accessed 7 Nov. 2017].
Sapa, M., 2011. Psychological state estimation from
physiological recordings during robot-assisted gait
rehabilitation. Journal of rehabilitation research and
development, 48(4), p.367.
Siewiorek, D.P., Smailagic, A., Furukawa, J., Krause, A.,
Moraveji, N., Reiger, K., Shaffer, J. and Wong, F.L.,
2003, October. SenSay: A Context-Aware Mobile
Phone. In ISWC (Vol. 3, p. 248).
Silva, J.L., Ornelas, J.D. and Silva, J.C., 2016, June. Make
it ISI: interactive systems integration tool.
In Proceedings of the 8th ACM SIGCHI Symposium
on Engineering Interactive Computing Systems (pp.
245-250). ACM.
Tobii Technologies. 2017. Get your Tobii Eye Tracker 4C.
[online] Available at: https://tobiigaming.com/eye-
tracker-4c/ [Accessed 7 Nov. 2017].
Vertegaal, R., 2003. Attentive user
interfaces. Communications of the ACM, 46(3),
pp.30-33.
Vertegaal, R., Shell, J.S., Chen, D. and Mamuji, A., 2006.
Designing for augmented attention: Towards a
Physiologically Attentive User Interface for Robot Teleoperation - Real Time Emotional State Estimation and Interface Modification using
Physiology, Facial Expressions and Eye Movements
301