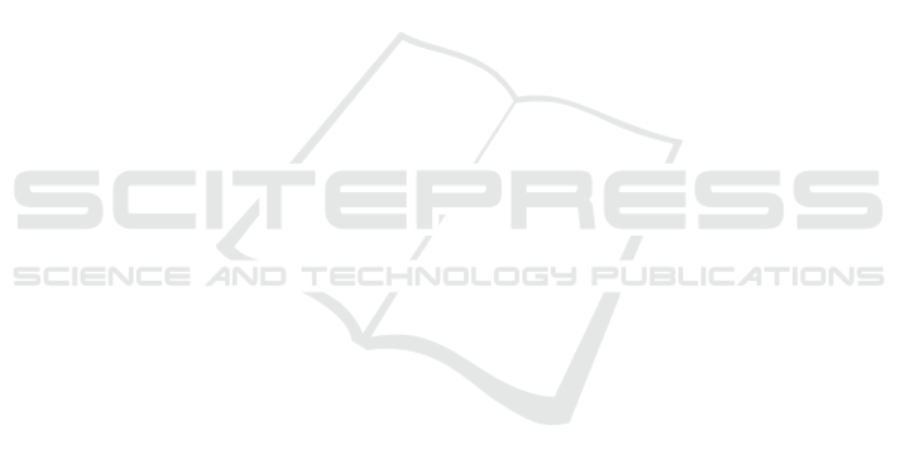
expect a possibility of the discrimination between rel-
evant and non-relevant cases.
Making good use of existing mappings in the clin-
ical domain (e.g., SNOMED-CT and other ontolo-
gies) is also important. The benefit from existing
mappings includes detailed evaluations and character-
ization of mappings. In other words, it is feasible to
generalize the mapping knowledge from a gold stan-
dard and apply the knowledge on different mapping
tasks.
It is possible to compare all items of MDS and
ICF exhaustedly. However, for larger ontologies such
as SNOMED-CT, such approach will be obstructed
by computational issues. An investigation on candi-
date generation for clinical ontology mapping is also
a need. It is also worthy to find a mapping method
leveraging structural information when two ontolo-
gies are both well-structured.
As clinical ontology mapping requires curation
by domain experts, utilizing checking results by live-
feedback mechanisms is useful. A semi-supervised
mapping method will help to improve the mapping
quality.
Lastly, multiple ontologies are preferable because
it increase the coverage of assessment sheets. How-
ever, such advantage comes with challenges, includ-
ing ontology merging and deduplication. Further-
more, even when using more ontologies, it is not guar-
anteed a 100% coverage because assessment sheets
are customized for a local society. Such problem hap-
pen when we work on other health care systems as
well. Therefore, it is important to study how to define
new items with sufficient logical relations.
4 CONCLUSION
We described an ongoing research project in care
plan recommendation system in Japan and its prelim-
inary step, which is the mapping of clinical ontolo-
gies. We proposed a straightforward yet reasonable
method for mapping MDS and ICF ontologies. Al-
though there are still many challenges to overcome
as discussed, we envision an optimistic form of the
direction. We hope our study could contribute to im-
proving the quality of healthcare in different societies.
ACKNOWLEDGMENTS
This research is funded by Welmo inc.
REFERENCES
Carbonell, J. and Goldstein, J. (1998). The Use of MMR,
Diversity-based Reranking for Reordering Documents
and Producing Summaries. In Annual International
ACM SIGIR Conference on Research and Develop-
ment in Information Retrieval, pages 335–336.
Choi, Y., Chiu, C. Y.-I., and Sontag, D. (2016). Learning
Low-Dimensional Representations of Medical Con-
cepts. AMIA Summits on Translational Science,
2016:41–50.
Deng, L., Yu, D., et al. (2014). Deep learning: Methods
and applications. Foundations and Trends
R
in Signal
Processing, 7(3–4):197–387.
Fung, K. W. and Bodenreider, O. (2005). Utilizing the
UMLS for Semantic Mapping Between Terminolo-
gies. In AMIA Annual Symposium, volume 2005,
pages 266–270.
Kenter, T. and De Rijke, M. (2015). Short Text Similar-
ity with Word Embeddings. In ACM International on
Conference on Information and Knowledge Manage-
ment, pages 1411–1420.
LeCun, Y., Bengio, Y., and Hinton, G. (2015). Deep Learn-
ing. Nature, 521(7553):436–444.
Lomax, J. and McCray, A. T. (2004). Mapping the Gene
Ontology into the Unified Medical Language System.
Comparative and Functional Genomics, 5(4):354–
361.
Mikolov, T., Chen, K., Corrado, G., and Dean, J. (2013). Ef-
ficient Estimation of Word Representations in Vector
Space. arXiv preprint arXiv:1301.3781.
Pyysalo, S., Ginter, F., Moen, H., Salakoski, T., and Anani-
adou, S. (2013). Distributional Semantics Resources
for Biomedical Text Processing. In International Sym-
posium on Languages in Biology and Medicine, pages
39–43.
Shvaiko, P. and Euzenat, J. (2013). Ontology Match-
ing: State of the art and Future Challenges. IEEE
Transactions on Knowledge and Data Engineering,
25(1):158–176.
Tu, S. W., Nyulas, C. I., Tudorache, T., and Musen, M. A.
(2015). A Method to Compare ICF and SNOMED CT
for Coverage of US Social Security Administrations
Disability Listing Criteria. In AMIA Annual Sympo-
sium, volume 2015, pages 1224–1233.
Tuttle, M. S., Weida, T., White, T., and Harvell, J. (2007).
Standardizing the MDS with LOINC
R
and vocabu-
lary matches. Report, at U.S. Department of Health
and Human Services.
Wu, Z. and Palmer, M. (1994). Verbs Semantics and Lex-
ical Selection. In Annual meeting on Association for
Computational Linguistics, pages 133–138.