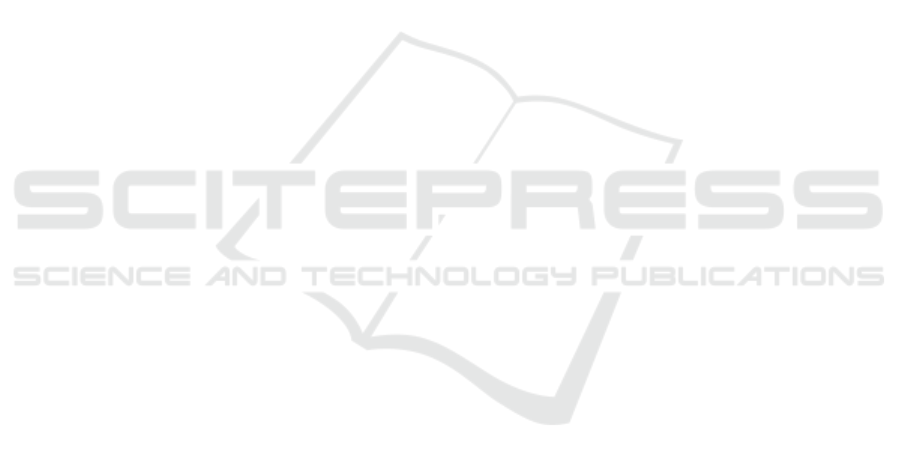
ques and frameworks (e.g. TensorFlow, Keras) on ad-
hoc hardware (e.g. NVidia GPUs) in order to further
improve the proposed approach.
ACKNOWLEDGEMENTS
This research is partially funded by Regione Sar-
degna, Next generation Open Mobile Apps Develop-
ment (NOMAD) project, Pacchetti Integrati di Agevo-
lazione (PIA) - Industria Artigianato e Servizi (2013).
REFERENCES
Amrita and Ravulakollu, K. K. (2018). A hybrid intrusion
detection system: Integrating hybrid feature selection
approach with heterogeneous ensemble of intelligent
classifiers. I. J. Network Security, 20(1):41–55.
Bace, R. and Mell, P. (2001). Nist special publication on
intrusion detection systems. Technical report, BOOZ-
ALLEN AND HAMILTON INC MCLEAN VA.
Bijone, M. (2016). A survey on secure network: Intrusion
detection & prevention approaches. American Journal
of Information Systems, 4(3):69–88.
Bronte, R., Shahriar, H., and Haddad, H. M. (2016). A
signature-based intrusion detection system for web
applications based on genetic algorithm. In Procee-
dings of the 9th International Conference on Security
of Information and Networks, Newark, NJ, USA, July
20-22, 2016, pages 32–39. ACM.
Buczak, A. L. and Guven, E. (2016). A survey of data mi-
ning and machine learning methods for cyber security
intrusion detection. IEEE Communications Surveys
and Tutorials, 18(2):1153–1176.
Bukac, V., Tucek, P., and Deutsch, M. (2012). Advances
and challenges in standalone host-based intrusion de-
tection systems. In TrustBus, volume 7449 of Lecture
Notes in Computer Science, pages 105–117. Springer.
Das, N. and Sarkar, T. (2014). Survey on host and net-
work based intrusion detection system. Internatio-
nal Journal of Advanced Networking and Applicati-
ons, 6(2):2266.
Dietterich, T. G. (2000). Ensemble methods in machine le-
arning. In Multiple Classifier Systems, volume 1857
of Lecture Notes in Computer Science, pages 1–15.
Springer.
Garcia-Teodoro, P., D
´
ıaz-Verdejo, J. E., Maci
´
a-Fern
´
andez,
G., and V
´
azquez, E. (2009). Anomaly-based network
intrusion detection: Techniques, systems and challen-
ges. Computers & Security, 28(1-2):18–28.
Ghorbani, A. A., Lu, W., and Tavallaee, M. (2010). Net-
work Intrusion Detection and Prevention - Concepts
and Techniques, volume 47 of Advances in Informa-
tion Security. Springer.
Gomes, H. M., Barddal, J. P., Enembreck, F., and Bifet, A.
(2017). A survey on ensemble learning for data stream
classification. ACM Comput. Surv., 50(2):23:1–23:36.
Guo, H., Li, Y., Shang, J., Mingyun, G., Yuanyue, H.,
and Bing, G. (2017). Learning from class-imbalanced
data: Review of methods and applications. Expert
Syst. Appl., 73:220–239.
Holm, H. (2014). Signature based intrusion detection for
zero-day attacks: (not) A closed chapter? In 47th
Hawaii International Conference on System Sciences,
HICSS 2014, Waikoloa, HI, USA, January 6-9, 2014,
pages 4895–4904. IEEE Computer Society.
Munaiah, N., Meneely, A., Wilson, R., and Short, B. (2016).
Are intrusion detection studies evaluated consistently?
a systematic literature review.
Pfleeger, C. P. and Pfleeger, S. L. (2012). Security in Com-
puting, 4th Edition. Prentice Hall.
Potluri, S. and Diedrich, C. (2016). High performance in-
trusion detection and prevention systems: A survey. In
ECCWS2016-Proceedings fo the 15th European Con-
ference on Cyber Warfare and Security, page 260.
Academic Conferences and publishing limited.
Sagi, O. and Rokach, L. (2018). Ensemble learning: A sur-
vey. Wiley Interdisc. Rew.: Data Mining and Know-
ledge Discovery, 8(4).
Sekar, R., Gupta, A., Frullo, J., Shanbhag, T., Tiwari, A.,
Yang, H., and Zhou, S. (2002). Specification-based
anomaly detection: a new approach for detecting net-
work intrusions. In ACM Conference on Computer
and Communications Security, pages 265–274. ACM.
Sommer, P. (1999). Intrusion detection systems as evidence.
Computer Networks, 31(23-24):2477–2487.
Uppuluri, P. and Sekar, R. (2001). Experiences with
specification-based intrusion detection. In Recent Ad-
vances in Intrusion Detection, volume 2212 of Lecture
Notes in Computer Science, pages 172–189. Springer.
Viegas, E., Santin, A. O., and Oliveira, L. S. (2017). Toward
a reliable anomaly-based intrusion detection in real-
world environments. Computer Networks, 127:200–
216.
Wang, Y., Yang, K., Jing, X., and Jin, H. L. (2014). Pro-
blems of kdd cup 99 dataset existed and data prepro-
cessing. In Applied Mechanics and Materials, volume
667, pages 218–225. Trans Tech Publ.
Zainal, A., Maarof, M. A., Shamsuddin, S. M. H., and
Abraham, A. (2008). Ensemble of one-class classi-
fiers for network intrusion detection system. In IAS,
pages 180–185. IEEE Computer Society.
KDIR 2018 - 10th International Conference on Knowledge Discovery and Information Retrieval
148