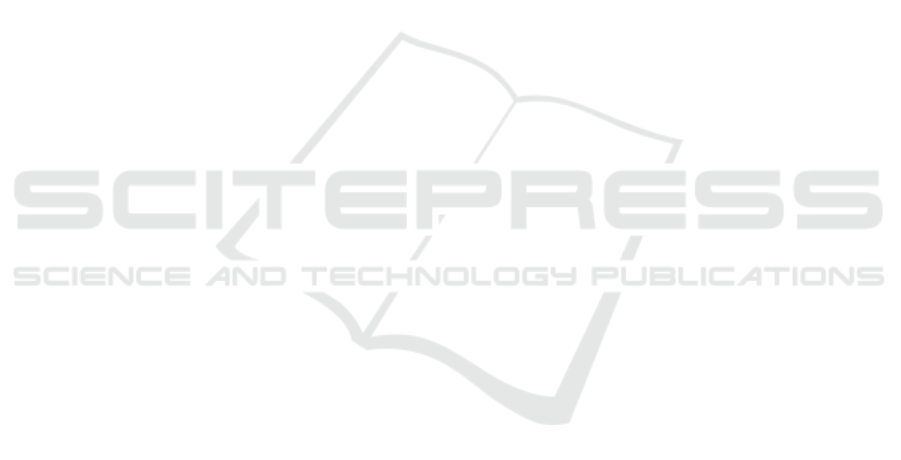
REFERENCES
Abraham, A., Grosan, C., and Martin-Vide, C. (2007). Evo-
lutionary design of intrusion detection programs. IJ
Network Security, 4(3):328–339.
Aburomman, A. A. and Reaz, M. B. I. (2017). A survey
of intrusion detection systems based on ensemble and
hybrid classifiers. Computers & Security, 65:135–
152.
Alkasassbeh, M., Al-Naymat, G., Hassanat, A. B., and
Almseidin, M. (2016). Detecting distributed denial of
service attacks using data mining techniques. Inter-
national Journal of Advanced Computer Science and
Applications, 7(1).
Ambusaidi, M. A., He, X., Nanda, P., and Tan, Z. (2016).
Building an intrusion detection system using a filter-
based feature selection algorithm. IEEE transactions
on computers, 65(10):2986–2998.
Clegg, J., Walker, J. A., and Miller, J. F. (2007). A new cros-
sover technique for cartesian genetic programming. In
Proceedings of the 9th annual conference on Gene-
tic and evolutionary computation, pages 1580–1587.
ACM.
Crosbie, M., Spafford, G., et al. (1995). Applying genetic
programming to intrusion detection. In Working No-
tes for the AAAI Symposium on Genetic Programming,
pages 1–8. Cambridge, MA: MIT Press.
Folino, G. and Pisani, F. S. (2015). Combining ensemble
of classifiers by using genetic programming for cyber
security applications. In European Conference on the
Applications of Evolutionary Computation, pages 54–
66. Springer.
Folino, G., Pizzuti, C., and Spezzano, G. (2005). Gp ensem-
ble for distributed intrusion detection systems. Pattern
Recognition and Data Mining, pages 54–62.
H2O.ai (2018). h2o: R Interface for H2O. Package version
3.18.0.2.
Luke, S. (1998). ECJ evolutionary computation li-
brary. Available for free at http://cs.gmu.edu/
∼eclab/projects/ecj/.
Miller, J. F. (2011). Cartesian genetic programming. In
Cartesian Genetic Programming, pages 17–34. Sprin-
ger.
Miller, J. F. and Smith, S. L. (2006). Redundancy and com-
putational efficiency in cartesian genetic program-
ming. IEEE Transactions on Evolutionary Computa-
tion, 10(2):167–174.
Miller, J. F. and Thomson, P. (2000). Cartesian genetic pro-
gramming. In European Conference on Genetic Pro-
gramming, pages 121–132. Springer.
Mohammad, R. M., McCluskey, L., and Thabtah, F.
(2015). UCI machine learning repository: Phis-
hing websites data set. https://archive.ics.uci.edu/
ml/datasets/Phishing+Websites.
Moustafa, N. and Slay, J. (2015). Unsw-nb15: a com-
prehensive data set for network intrusion detection
systems (unsw-nb15 network data set). In Mili-
tary Communications and Information Systems Con-
ference (MilCIS), 2015, pages 1–6. IEEE.
Moustafa, N. and Slay, J. (2016). The evaluation of net-
work anomaly detection systems: Statistical analysis
of the unsw-nb15 data set and the comparison with
the kdd99 data set. Information Security Journal: A
Global Perspective, 25(1-3):18–31.
Orfila, A., Estevez-Tapiador, J. M., and Ribagorda, A.
(2009). Evolving high-speed, easy-to-understand net-
work intrusion detection rules with genetic program-
ming. In Workshops on Applications of Evolutionary
Computation, pages 93–98. Springer.
Scarfone, K. and Mell, P. (2007). Guide to intrusion de-
tection and prevention systems (idps). NIST special
publication, 800(2007):94.
Sen, S. (2015). A survey of intrusion detection systems
using evolutionary computation. Bio-Inspired Com-
putation in Telecommunications, pages 73–94.
Sen, S. and Clark, J. A. (2011). Evolutionary computation
techniques for intrusion detection in mobile ad hoc
networks. Computer Networks, 55(15):3441–3457.
Song, J., Takakura, H., Okabe, Y., Eto, M., Inoue, D., and
Nakao, K. (2011). Statistical analysis of honeypot
data and building of kyoto 2006+ dataset for nids eva-
luation. In Proceedings of the First Workshop on Buil-
ding Analysis Datasets and Gathering Experience Re-
turns for Security, pages 29–36. ACM.
Thabtah, F., Mohammad, R. M., and McCluskey, L. (2016).
A dynamic self-structuring neural network model to
combat phishing. In Neural Networks (IJCNN), 2016
International Joint Conference on, pages 4221–4226.
IEEE.
Wolpert, D. H. (1992). Stacked generalization. Neural net-
works, 5(2):241–259.
Wu, S. X. and Banzhaf, W. (2010). The use of computa-
tional intelligence in intrusion detection systems: A
review. Applied Soft Computing, 10(1):1–35.
Applying Cartesian Genetic Programming to Evolve Rules for Intrusion Detection System
183