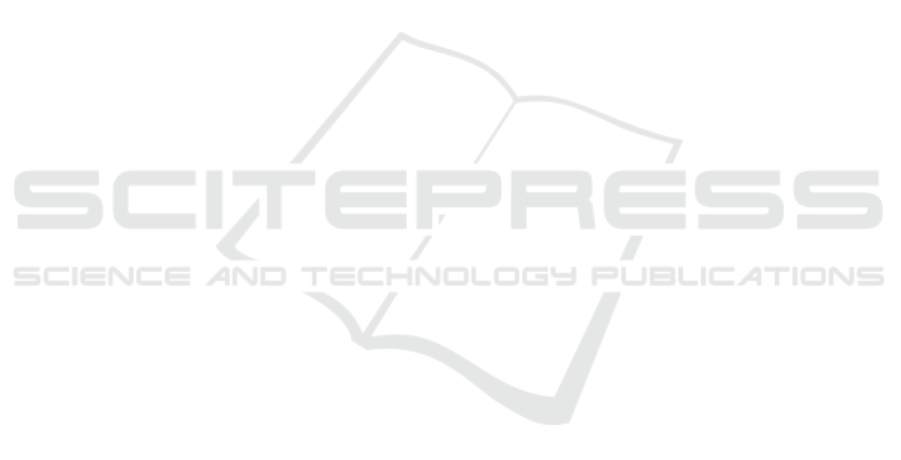
approach that well suits a niche of energy resources
where it outperformed the established SVD.
All in all, the XSD has several advantages regard-
ing performance in scenarios where a frequent train-
ing is necessary and it produces a set of schedules for
optimization that better resembles the original feasi-
ble region especially at the boundary. Thus the flex-
ibility of the energy unit is better harnessed. Nev-
ertheless, it has some problems capturing the opera-
tional interdependencies over time with some devices
like an electric vehicle charging station, where a set
of functionals has to be captured by the decoder that
all produce a given value for the integral over the
planning horizon. So, the χ-shape decoder may not
completely replace the support vector decoder but is
a good extension beside it with advantages for many
types of energy units.
REFERENCES
Arnold, D. V. and Hansen, N. (2012). A (1+1)-cma-es
for constrained optimisation. In Proceedings of the
14th Annual Conference on Genetic and Evolution-
ary Computation, GECCO ’12, pages 297–304, New
York, NY, USA. ACM.
Braune, C., Dankel, M., and Kruse, R. (2016). Obtaining
shape descriptors from a concave hull-based cluster-
ing algorithm. In Boström, H., Knobbe, A., Soares,
C., and Papapetrou, P., editors, Advances in Intelli-
gent Data Analysis XV, pages 61–72, Cham. Springer
International Publishing.
Bremer, J. and Lehnhoff, S. (2017). Hybrid Multi-ensemble
Scheduling, pages 342–358. Springer International
Publishing, Cham.
Bremer, J. and Lehnhoff, S. (2018). Phase-space sampling
of energy ensembles with CMA-ES. In EvoApplica-
tions, volume 10784 of Lecture Notes in Computer
Science, pages 222–230. Springer.
Bremer, J., Rapp, B., and Sonnenschein, M. (2010). Sup-
port vector based encoding of distributed energy re-
sources’ feasible load spaces. In IEEE PES Confer-
ence on Innovative Smart Grid Technologies Europe,
Chalmers Lindholmen, Gothenburg, Sweden.
Bremer, J., Rapp, B., and Sonnenschein, M. (2011). Encod-
ing distributed search spaces for virtual power plants.
In IEEE Symposium Series on Computational Intelli-
gence 2011 (SSCI 2011), Paris, France.
Bremer, J. and Sonnenschein, M. (2013). Constraint-
handling for optimization with support vector surro-
gate models – a novel decoder approach. In Fil-
ipe, J. and Fred, A., editors, Proceedings of the
5th International Conference on Agents and Artifi-
cial Intelligence (2), pages 91–105, Barcelona, Spain.
SciTePress.
Duckham, M., Kulik, L., Worboys, M., and Galton, A.
(2008). Efficient generation of simple polygons for
characterizing the shape of a set of points in the plane.
Pattern Recognition, 41(10):3224 – 3236.
Edelsbrunner, H., Kirkpatrick, D., and Seidel, R. (2006).
On the shape of a set of points in the plane. IEEE
Trans. Inf. Theor., 29(4):551–559.
Fröhlinger, J. (2017). Abstract Flexibility Description for
Virtual Power Plant Scheduling. phd thesis, Carl von
Ossietzly Universität, Oldenburg.
Hansen, N. (2006). The CMA evolution strategy: a compar-
ing review. In Lozano, J., Larranaga, P., Inza, I., and
Bengoetxea, E., editors, Towards a new evolutionary
computation. Advances on estimation of distribution
algorithms, pages 75–102. Springer.
Hansen, N. (2011). The CMA Evolution Strategy: A Tuto-
rial. Technical report.
Hörding, J. P. (2017). Umsetzung eines Dekoders für
kaskadierende χ-Shape Modelle verteilter Energiean-
lagen. Bachelorthesis, Department of Energyinfor-
matics, University of Oldenburg, Oldenburg, Ger-
many.
Kim, D. G. (1998). Riemann mapping based constraint han-
dling for evolutionary search. In SAC, pages 379–385.
Koziel, S. and Michalewicz, Z. (1999). Evolutionary al-
gorithms, homomorphous mappings, and constrained
parameter optimization. Evol. Comput., 7:19–44.
Kramer, O., Barthelmes, A., and Rudolph, G. (2009). Sur-
rogate constraint functions for cma evolution strate-
gies. In Proceedings of the 32Nd Annual German
Conference on Advances in Artificial Intelligence,
KI’09, pages 169–176, Berlin, Heidelberg. Springer-
Verlag.
Melkemi, M. and Djebali, M. (2000). Computing the shape
of a planar points set. Pattern Recognition, 33(9):1423
– 1436.
Moreira, A. and Santos, M. Y. (2007). Concave hull: A
k-nearest neighbours approach for the computation of
the region occupied by a set of points. Proceedings
of the Second International Conference on Computer
Graphics Theory and Applications.
Neugebauer, J., Bremer, J., Hinrichs, C., Kramer, O., and
Sonnenschein, M. (2016). Generalized cascade classi-
fication model with customized transformation based
ensembles. In IJCNN.
Ostermeier, A., Gawelczyk, A., and Hansen, N. (1994). A
derandomized approach to self-adaptation of evolu-
tion strategies. Evolutionary Computation, 2(4):369–
380.
Park, J.-S. and Oh, S.-J. (2012). A new concave hull al-
gorithm and concaveness measure for n-dimensional
datasets. Journal of Information science and engi-
neering, 28(3):587–600.
Rosen, E., Jansson, E., and Brundin, M. (2014). Implemen-
tation of a fast and efficient concave hull algorithm.
Technical report, Uppsala Univ, Finland.
Shimrat, M. (1962). Algorithm 112: Position of point rela-
tive to polygon. Commun. ACM, 5(8):434–.
Sonnenschein, M., Lünsdorf, O., Bremer, J., and Tröschel,
M. (2014). Decentralized control of units in smart
grids for the support of renewable energy supply. En-
vironmental Impact Assessment Review, (0):–.
Tax, D. M. J. and Duin, R. P. W. (2004). Support vector data
description. Mach. Learn., 54(1):45–66.
A Cascading Chi-shapes based Decoder for Constraint-handling in Distributed Energy Management
191