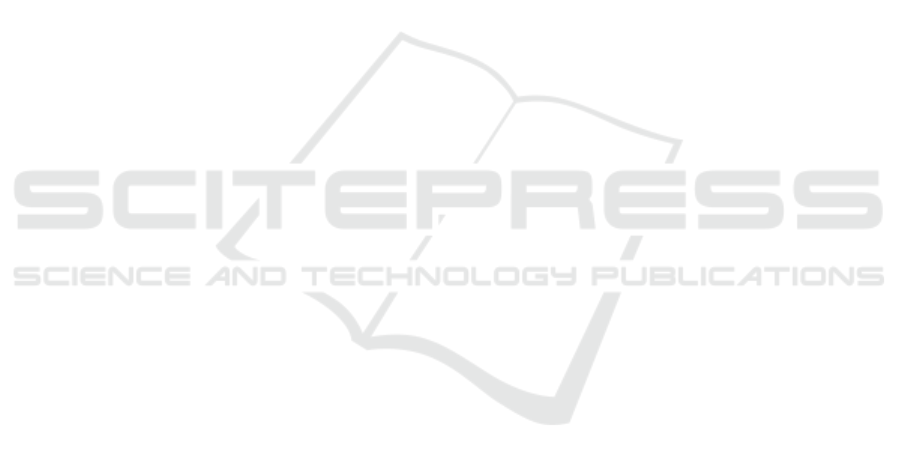
to support the clinical research on the development of
new imaging biomarkers by combining clinical data
with information coming from different medical dom-
ains (2) to improve the quality of the clinical healt-
hcare that tend to provide personalized treatments to
patients via the use of clinical guidelines that are ba-
sed on evaluation criteria. In this paper, we employed
the VASARI terminology as a proof of concept for the
demonstration of the feasibility and the importance of
making RDF and OWL data available to describe ce-
rebral tumors observations and determining the key
concepts and relationships that are central in their eva-
luation. Our work can be easily expanded to answer
to other use cases; thanks to the modular aspect of
the ontology and to the OWL language that is self-
descriptive (concepts are textually and formally des-
cribed in the ontology to guide users) and extendable.
REFERENCES
Amdouni, E. and Gibaud, B. (2016). Concept-based ver-
sus realism-based approach to represent neuroima-
ging observations. In Keod: Proceedings of The
8th International Joint Conference On Knowledge
Discovery, Knowledge Engineering and Knowledge
Management-Vol. 2, pages 179–185.
Bennett, B., Chaudhri, V., and Dinesh, N. (2013). A voca-
bulary of topological and containment relations for a
practical biological ontology. In International Confe-
rence on Spatial Information Theory, pages 418–437.
Springer.
Bittner, T. and Smith, B. (2004). Normalizing medical on-
tologies using basic formal ontology.
Brinkman, R., Courtot, M., Derom, D., Fostel, J., He, Y.,
Lord, P., Malone, J., Parkinson, H., Peters, B., Rocca-
Serra, P., et al. (2010). Modeling biomedical experi-
mental processes with OBI. J. Biomedical Semantics,
1(S-1):S7.
Ceusters, W. (2012). An information artifact ontology per-
spective on data collections and associated represen-
tational artifacts. In MIE, pages 68–72.
Ceusters, W., Elkin, P., and Smith, B. (2006). Referent
tracking: The problem of negative findings. Studies
in health technology and informatics, 124:741.
Ceusters, W. and Smith, B. (2005). Tracking referents in
electronic health records. Studies in health technology
and informatics, 116:71.
Channin, D., Mongkolwat, P., Kleper, V., Sepukar, K.,
and Rubin, D. (2010). The caBIG annotation and
image markup project. Journal of digital imaging,
23(2):217–225.
Cimino, J. (2006). In defense of the desiderata. Journal of
biomedical informatics, 39(3):299–306.
Clunie, D. (2000). DICOM structured reporting. PixelMed
Publishing.
Clunie, D. (2007). DICOM structured reporting and cancer
clinical trials results. Cancer informatics, 4:33.
Eisenhauer, E., Therasse, P., B. J., Schwartz, L., Sargent,
D., Ford, R., Dancey, J., Arbuck, S., Gwyther, S., M.,
M., et al. (2009). New response evaluation criteria in
solid tumours: revised recist guideline (version 1.1).
European journal of cancer, 45(2):228–247.
ESR (2011). Medical imaging in personalised medicine:
a white paper of the research committee of the euro-
pean society of radiology (esr). Insights into imaging,
2(6):621–630.
Gillies, R., Kinahan, P., and Hricak, H. (2015). Radiomics:
images are more than pictures, they are data. Radio-
logy, 278(2):563–577.
Gkoutos, G., Schofield, P., and Hoehndorf, R. (2012). The
units ontology: a tool for integrating units of measu-
rement in science. Database, 2012:bas033.
Grams, A., Gempt, J., Ringel, F., Soehngen, E., Astner, S.,
Schlegel, J., Meyer, B., Zimmer, C., and Forschler,
A. (2014). Multimodal imaging to delineate tumor
heterogeneity in cerebral gliomas. Open Journal of
Radiology, 4(02):182.
Gruber, T. (1993). A translation approach to portable onto-
logy specifications. Knowledge acquisition, 5(2):199–
220.
Gutman, D., Cooper, L., Hwang, S., Holder, C., Gao, J.,
Aurora, T., Dunn Jr, W., Scarpace, L., Mikkelsen,
T., Jain, R., et al. (2013). Mr imaging predictors
of molecular profile and survival: multi-institutional
study of the tcga glioblastoma data set. Radiology,
267(2):560–569.
Kahn J.R., C., Langlotz, C., Channin, D., and Rubin, D.
(2011). Informatics in radiology: an information mo-
del of the dicom standard. Radiographics, 31(1):295–
304.
Levy, M., Freymann, J., Kirby, J., Fedorov, A., Fennessy,
F., Eschrich, S., Berglund, A., Fenstermacher, D., Tan,
Y., G. X., et al. (2012). Informatics methods to enable
sharing of quantitative imaging research data. Magne-
tic resonance imaging, 30(9):1249–1256.
Levy, M., OConnor, M., and Rubin, D. (2009). Seman-
tic reasoning with image annotations for tumor asses-
sment. In AMIA Annual Symposium Proceedings, vo-
lume 2009, page 359. American Medical Informatics
Association.
Madhavan, S., Zenklusen, J., Kotliarov, Y., Sahni, H., Fine,
H., and Buetow, K. (2009). Rembrandt: helping per-
sonalized medicine become a reality through integra-
tive translational research. Molecular cancer rese-
arch, 7(2):157–167.
M
¨
oller, M., Regel, S., and Sintek, M. (2009). Radsem: Se-
mantic annotation and retrieval for medical images.
In European Semantic Web Conference, pages 21–35.
Springer.
Mungall, C., Gkoutos, G., Washington, N., and Lewis, S.
(2007). Representing phenotypes in OWL. In Pro-
ceedings of the OWLED 2007 Workshop on OWL: Ex-
perience and Directions. Innsbruck, Austria Edited by
Golbreich C, Kalyanpur A, Parsia B.
Oberkampf, H., Zillner, S., Bauer, B., and Hammon, M.
(2012). Interpreting patient data using medical back-
ground knowledge. ICBO, 897:3.
Semantic Representation of Neuroimaging Observations: Proof of Concept based on the VASARI Terminology
73