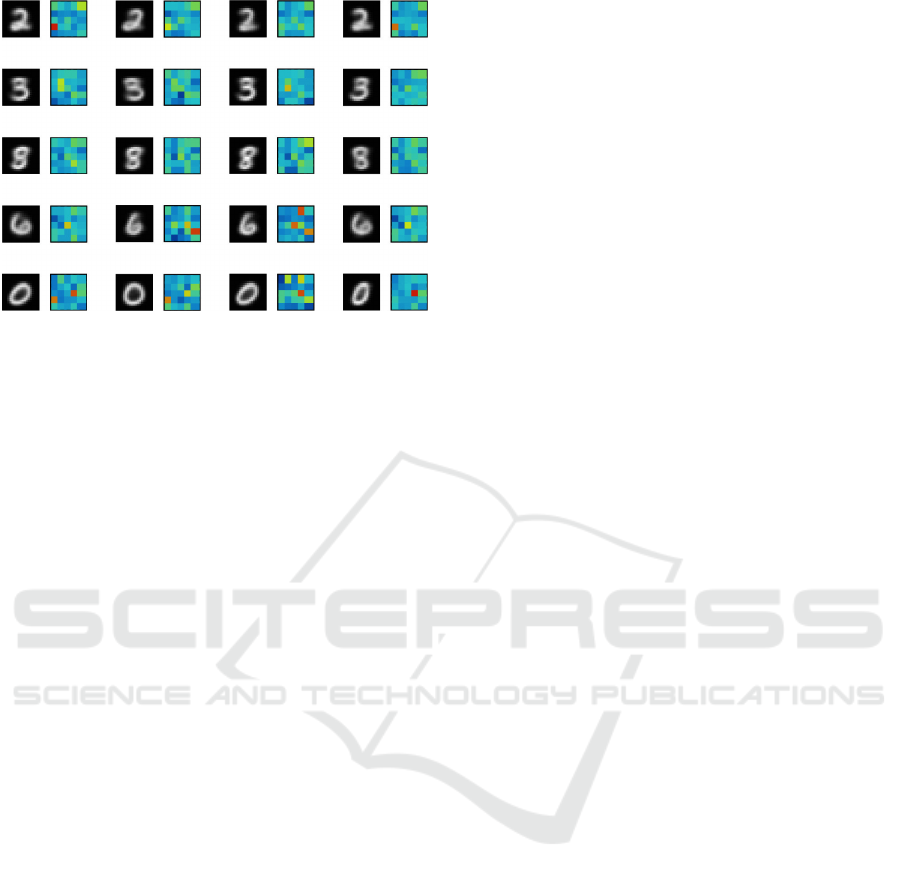
Figure 3: Twenty examples of learned prototypes from dif-
ferent neurons of group a that represent numbers of similar
shape. Visualization as in Fig. 2.
prototypes it can also be seen, that the ensemble acti-
vity tends to be sparse with only a few neurons exhi-
biting a strong activation in response to a particular
input.
One interesting question regarding such a sparse,
distributed representation is the degree of variation in
this ensemble code if very similar inputs are proces-
sed. Figure 3 shows twenty examples of dendritic pro-
totypes from different neurons of group a that repre-
sent numbers of similar shape. The captured ensem-
ble activities of group b indicate that the similarity of
activation patterns appears to match well with the si-
milarity of the corresponding MNIST patterns. Even
in cases with an overall low activation like the respon-
ses to the number “8” (third row), the corresponding
ensemble codes appear similar.
These first tentative results indicate, that the pro-
posed model appears to be able to learn a sparse, dis-
tributed representation of its main input space (here
the MNIST set), while simultaneously learning the
ensemble codes of an accompanying group of neu-
rons that operates on the same input space.
6 CONCLUSION
The cortical column model outlined in this paper is
still in a very early stage. Yet, it combines multiple,
novel ideas that will guide our future research. First,
the RGNG-based, unsupervised learning of a sparse,
distributed input space representation utilizes a clas-
sic approach of prototype-based learning in a novel
way. Instead of establishing a one-to-one relation be-
tween a learned representation (prototype) and a cor-
responding region of input space, it learns an ensem-
ble code that utilizes the response of multiple neurons
(sets of prototypes) to a given input. The presented
preliminary results indicate that learning such an en-
semble code appears feasible. Our future research in
this regard will focus on improving our understanding
of the resulting ensemble code, as well as improving
the RGNG algorithm in terms of learning speed and
robustness w.r.t. a continuous learning regime.
Second, the idea of reciprocally connecting two
neuron groups that process a shared input space ena-
bles the creation of an autoassociative memory cell
(AMC) that is able to maintain an active state even in
the absence of any input. In addition, such an AMC
may exhibit some form of attractor dynamics where
the activities of the two, reciprocally connected neu-
ron groups self-stabilize. A precondition for such an
AMC is the groups’ ability to learn the ensemble code
of the respective other group. The presented prelimi-
nary results indicate that this is possible. Our future
research will focus on understanding the characteris-
tics of the dynamics of such an AMC, e.g., in response
to various kinds of input disturbances.
Third, the outlined cortical column model sug-
gests that the cortex may consist of a network of
autoassociative memory cells that influence each ot-
her via feedback as well as feedforward connections
while trying to achieve locally stable attractor states.
In addition, further cortical circuitry may modulate
the activity of individual cortical columns to facilitate
competition among cortical columns or groups of cor-
tical columns on a more global level, which may then
lead to the emergence of stable, global attractor states
that are able to temporarily bind together sets of corti-
cal columns. In this context our research is still in its
infancy and will focus on implementing a first version
of a full cortical column model that can then be tested
in small hierarchical network configurations, e.g., to
process more complex visual input.
REFERENCES
Buxhoeveden, D. P. and Casanova, M. F. (2002). The
minicolumn hypothesis in neuroscience. Brain,
125(5):935–951.
Chen, T.-W., Wardill, T. J., Sun, Y., Pulver, S. R., Rennin-
ger, S. L., Baohan, A., Schreiter, E. R., Kerr, R. A.,
Orger, M. B., Jayaraman, V., Looger, L. L., Svoboda,
K., and Kim, D. S. (2013). Ultrasensitive fluores-
cent proteins for imaging neuronal activity. Nature,
499(7458):295–300.
Fyhn, M., Molden, S., Witter, M. P., Moser, E. I., and Mo-
ser, M.-B. (2004). Spatial representation in the entor-
hinal cortex. Science, 305(5688):1258–1264.
Hafting, T., Fyhn, M., Molden, S., Moser, M.-B., and Mo-
ser, E. I. (2005). Microstructure of a spatial map in
the entorhinal cortex. Nature, 436(7052):801–806.
A Grid Cell Inspired Model of Cortical Column Function
209