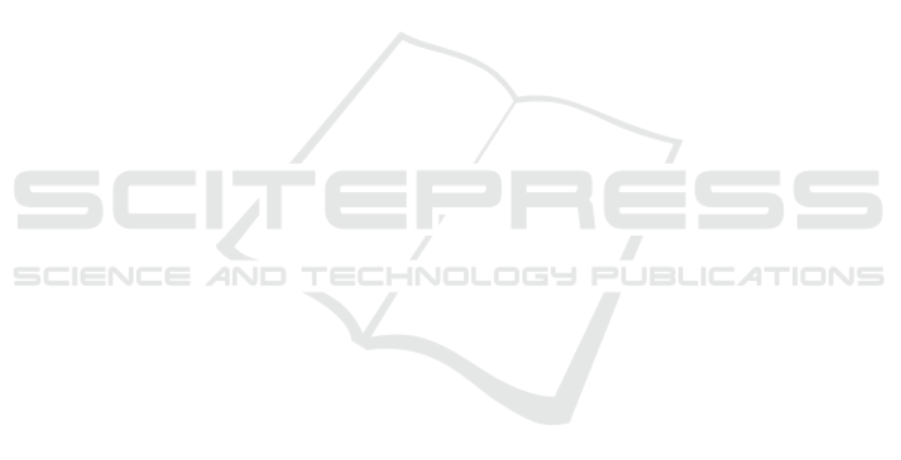
most recent information. It is important to highlight
the dynamism of the cube as it supports the
continuous inclusion of new measures and
dimensions.
Among the main benefits of this framework is the
fact that the indicators are directly linked to the social
measures, so that it is possible to easily identify the
origin of the values of these indicators. On the other
hand, the fact that the indicators are also semantic
data, makes it possible to apply validation techniques
during their definition and derivation.
As future work will be studied the automatic
creation of descriptions and queries associated with
the calculation of social indicators, as well as the
discovery of appropriate metrics to evaluate strategic
objectives of the organization. Due to the dynamism
of the cubes, the volume and fluctuating character of
the data, makes it impracticable to store historical
data, so it is necessary to establish the appropriate
mechanisms to find the right time window to apply
predictive algorithms and compare measurement
trends.
ACKNOWLEDGEMENTS
This work has been financed by the Ministry of
Economy and Trade with the project of the National
R&D Plan with contract number TIN2017-88805-R.
We also have the support of the Universitat Jaume I
pre-doctoral scholarship programme
(PREDOC/2017/28).
REFERENCES
Barone, D., Jiang, L., Amyot, D. and Mylopoulos, J., 2011.
Composite Indicators for Business Intelligence.
Conference on Conceptual Modeling ER 2011. Lecture
Notes in Computer Science, 6998, pp. 448–458.
Berlanga, R. and Nebot, V., 2015. Context-Aware Business
Intelligence. Business Intelligence. Lecture Notes in
Business Information Processing, 253, pp. 87-110.
Berlanga, R., García-Moya, L., Nebot, V., Aramburu, M.,
Sanz, I. and Llidó, D., 2015. SLOD-BI: An Open Data
Infrastructure for Enabling Social Business
Intelligence. International Journal on Data
Warehousing and Data Mining, 11(4), pp. 1-28.
Chae, B. K., 2015. Insights from hashtag# supplychain and
Twitter analytics: Considering Twitter and Twitter data
for supply chain practice and research. International
Journal of Production Economics, 165, pp. 247-259.
Dai, W., Han, D., Dai, Y. and Xu, D., 2015. Emotion
recognition and affective computing on vocal social
media. Information & Management, 52(7), pp. 777-
788.
Diamantini, C., Potena, D. and Storti, E., 2016. SemPI: A
semantic framework for the collaborative construction
and maintenance of a shared dictionary of performance
indicators. Future Generation Comp. Syst., 54, pp. 352-
365.
Fan, W. and Gordon, M. D., 2014. The Power of Social
Media Analytics. Communications of the ACM, 57(6),
pp. 74-81.
Feng, W., Zhang, C., Zhang, W., Han, J., Wang, J.,
Aggarwal, C. and Huang, J., 2015. STREAMCUBE:
Hierarchical spatio-temporal hashtag clustering for
event exploration over the Twitter stream. IEEE 31st
International Conference on Data Engineering, pp.
1561-1572.
García-Moya, L., 2016. Modeling and analyzing opinions
from customer reviews. Tesis Doctoral, Departamento
de Lenguajes y Sistemas Informáticos, Universitat
Jaume I, Castellón.
He, W., Wu, H., Yan, G., Akula, V. and Shen, J., 2015. A
novel social media competitive analytics framework
with sentiment benchmarks. Information &
Management, 52(7), pp. 801-812.
Liu, X., Tang, K., Hancock, J., Han, J., Song, M., Xu, R.,
and Pokorny, B., 2013. A Text Cube Approach to
Human, Social and Cultural Behavior in the Twitter
Stream. International Conference on Social
Computing, Behavioral-Cultural Modeling and
Prediction, pp. 321-330.
Maté, A., Trujillo, J. and Mylopoulosb, J., 2017.
Specification and derivation of key performance
indicators for business analytics: A semantic approach.
Data & Knowledge Engineering journal, pp. 30–49.
Nebot, V. and Berlanga, R., 2016. Statistically-driven
generation of multidimensional analytical schemas
from linked data. Knowledge-Based Systems, 110, pp.
15-29.
Popova, V. and Sharpanskykh, A., 2011. Formal modelling
of organisational goals based on performance
indicators. Data & Knowledge Engineering, 70(4), pp.
335-364.
Wang, G. A., Jiao, J., Abrahams, A. S., Fan, W. and Zhang,
Z., 2013. ExpertRank: A topic-aware expert finding
algorithm for online knowledge communities. Decision
Support Systems, 54(3), pp. 1442-1451.
Yan, Z., Xing, M., Zhang, D. and Ma, B., 2015. EXPRS:
An extended pagerank method for product feature
extraction from online consumer reviews. Information
& Management, 52(7), pp. 850-858.
Zhou, M., Lei, L., Wang, J., Fan, W. and Wang, A. G.,
2015. Social Media Adoption and Corporate
Disclosure. Journal of Information Systems, 29(2), pp.
23-50.
KDIR 2018 - 10th International Conference on Knowledge Discovery and Information Retrieval
228